Sparse to Dense Ground Truth Pre-Processing in Hyperspectral Imaging for In-Vivo Brain Tumour Detection
2023 IEEE International Conference on Metrology for eXtended Reality, Artificial Intelligence and Neural Engineering (MetroXRAINE)(2023)
关键词
segmentation,ground truth,brain tumour,hyperspectral
AI 理解论文
溯源树
样例
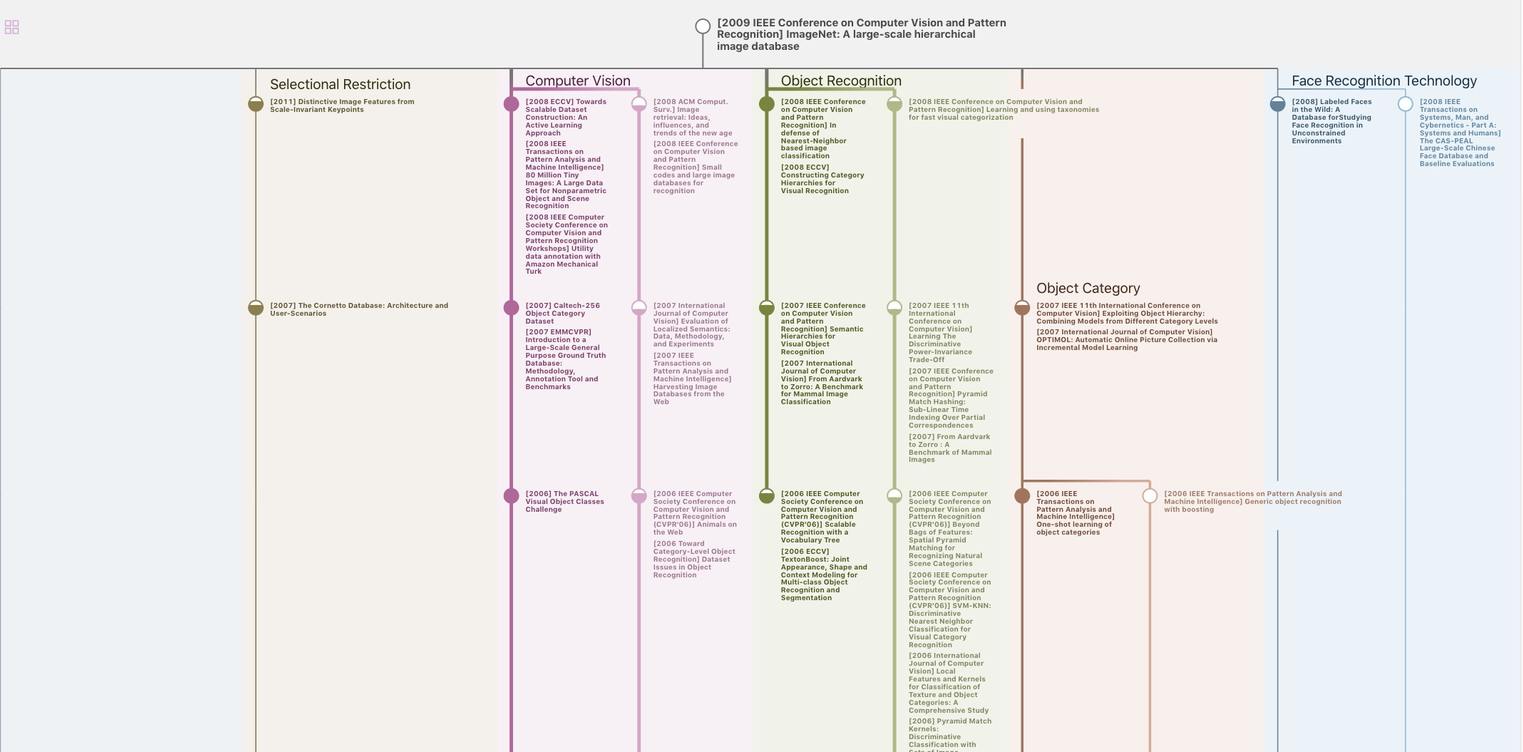
生成溯源树,研究论文发展脉络
Chat Paper
正在生成论文摘要