Feasibility of Tree-Based Machine Learning Models to Discriminate Safe and Unsafe Posture During Weight Lifting.
MetroXRAINE(2023)
Abstract
The weight lifting is defined as any activity requiring the use of human force to lift or move a load which can be potentially harmful of onsetting work-related musculoskeletal disorders. The purpose of this study was to explore the feasibility of four tree-based Machine Learning (ML) models - fed with time-domain features extracted from signals acquired by means of one inertial measurement unit (IMU) - to classify safe and unsafe postures during weight lifting. Inertial signals -linear acceleration and angular velocity - acquired from sternum of 4 healthy subjects were registered using the Mobility Lab System. The signals were manually segmented in order to extract for each region of interest, corresponding to the lifting, several time-domain features. Four tree-based predictive models - namely Decision Tree, Random Forest, Rotation Forest and AdaBoost Tree - were implemented and their performances were tested. Interesting results in terms of evaluation metrics for a binary safe/unsafe posture classification were obtained with accuracy values greater than 93%. In conclusion the present study indicated that tree-based ML models fed with specific features were able to discriminate safe and unsafe posture during weight lifting using only one IMU placed on the sternum. Future investigation on larger cohort could confirm the potential of the proposed methodology.
MoreTranslated text
Key words
weight lifting,feature extraction,health monitoring,lifting,safe/unsafe posture,inertial measurement unit,wearable sensors,occupational ergonomics,machine learning,work-related musculoskeletal disorders
AI Read Science
Must-Reading Tree
Example
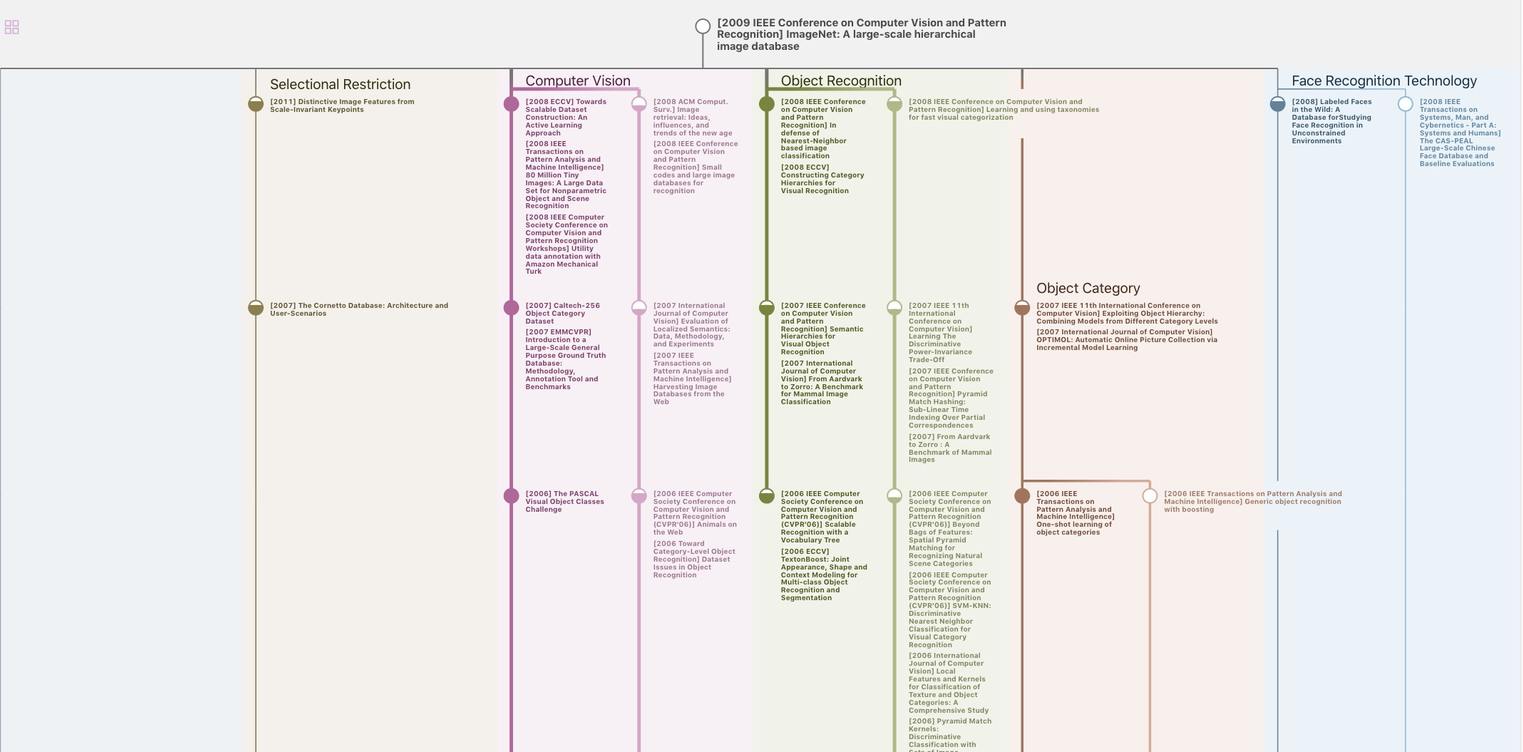
Generate MRT to find the research sequence of this paper
Chat Paper
Summary is being generated by the instructions you defined