Motion Planning of Industrial Robot by Data-Driven Optimization Using Petri Nets.
2023 IEEE International Conference on Industrial Engineering and Engineering Management (IEEM)(2023)
Abstract
Industrial robots have been actively introduced in various fields such as food, cosmetics, and pharmaceuticals, in addition to the automotive and electronics industries. Currently, the introduction of industrial robots requires instruction by skilled personnel. Therefore, there is a need to understand the entire system and detect errors so that not only skilled operators can easily instruct robots. In this study, we propose a motion planning method based on data-driven optimization of industrial robots using Petri nets, which are a discrete event system based on robot teaching data by skilled operators. Petri nets are automatically generated from the robot's event logs using an
$\alpha$
-algorithm. Using a Petri net simulator, we verify the consistency of automatically generated Petri nets and output the optimal firing sequence. The motion program is applied to a 6-axis robot arm (VS-060) on the robot operating system (ROS) to verify the effectiveness of motion planning optimization in multiple problem settings, including pick-and-place motion from multiple posture candidates. Experimental results show that our proposed method can reduce the sum of angle changes approximately 3% compared with conventional motion planning method using RRT using different initial postures.
MoreTranslated text
Key words
Path Planning,Petri Nets,Optimal Sequence,Multiple Candidate,Automatic Generation,Robot Operating System,Event Log,Direct Relationship,Amount Of Change,Data Logger,Position Information,Process Mining,Incidence Matrix,Robot Motion,Robot Behavior,Millennial Generation,Robot State,Flexible Manufacturing Systems
AI Read Science
Must-Reading Tree
Example
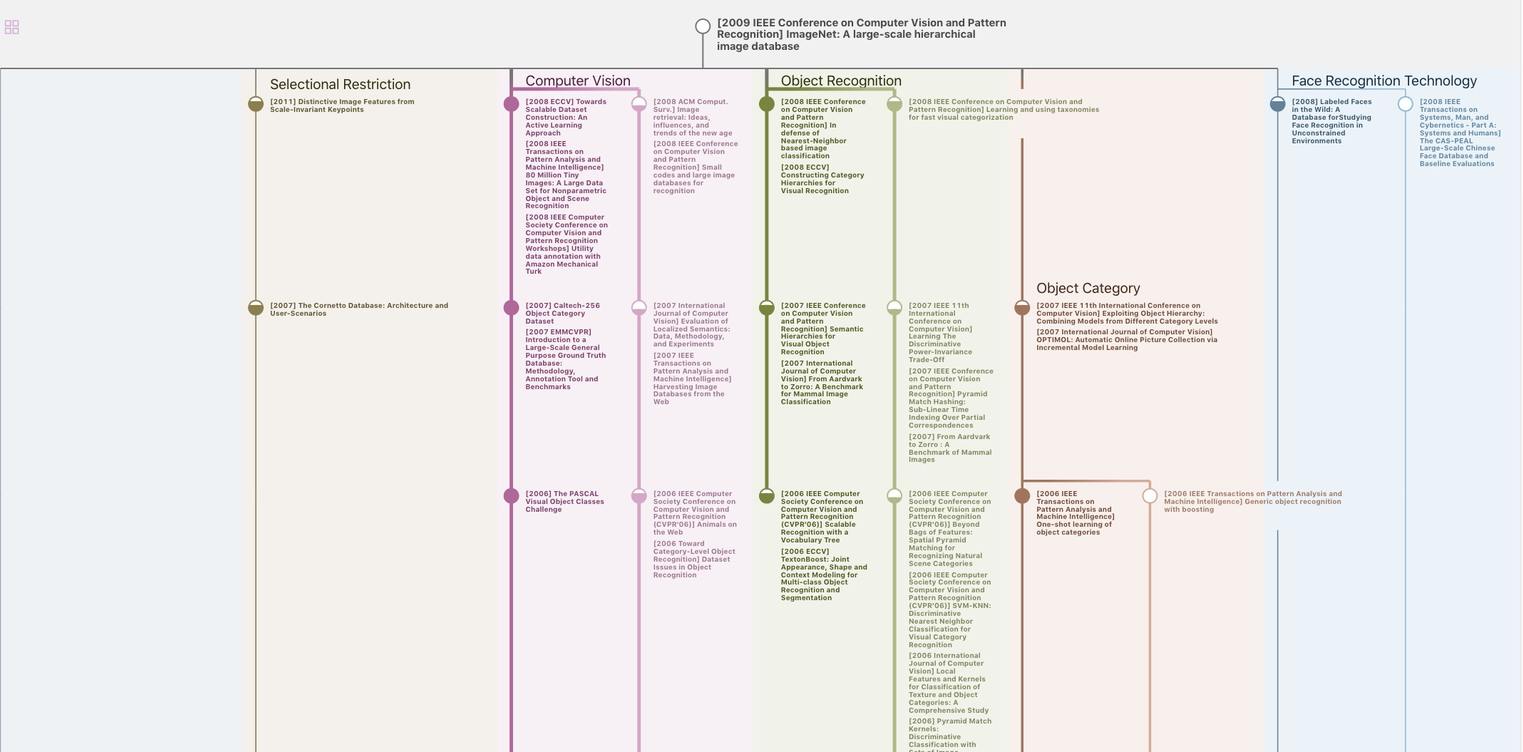
Generate MRT to find the research sequence of this paper
Chat Paper
Summary is being generated by the instructions you defined