GrMC: Towards Interpretable Classification Models That Are Also Accurate
2023 IEEE INTERNATIONAL CONFERENCE ON KNOWLEDGE GRAPH, ICKG(2023)
摘要
Interpretability of classification models is an important issue. However, there is a lack of intrinsically interpretable models that are also highly accurate. To fill the gap, we introduce a new classification model type, namely Group Model Committee (GrMC), and an associated learning algorithm. Our key ideas are: (1) Divide a classification task's data space into several groups such that each group is defined by a simple condition and it has a unique, interpretable group model; (2) A data instance belongs to a group if it satisfies the group's defining condition and it is classified by utilizing the group models of the groups it belongs to. Experiments show that small interpretable GrMC models are often more accurate than existing intrinsically interpretable models, and also more accurate than Random Forests models. GrMC also has other strengths.
更多查看译文
关键词
classification model,model type,interpretability,accuracy,instance group,group definition,group model,small,committee of group models,heterogeneity
AI 理解论文
溯源树
样例
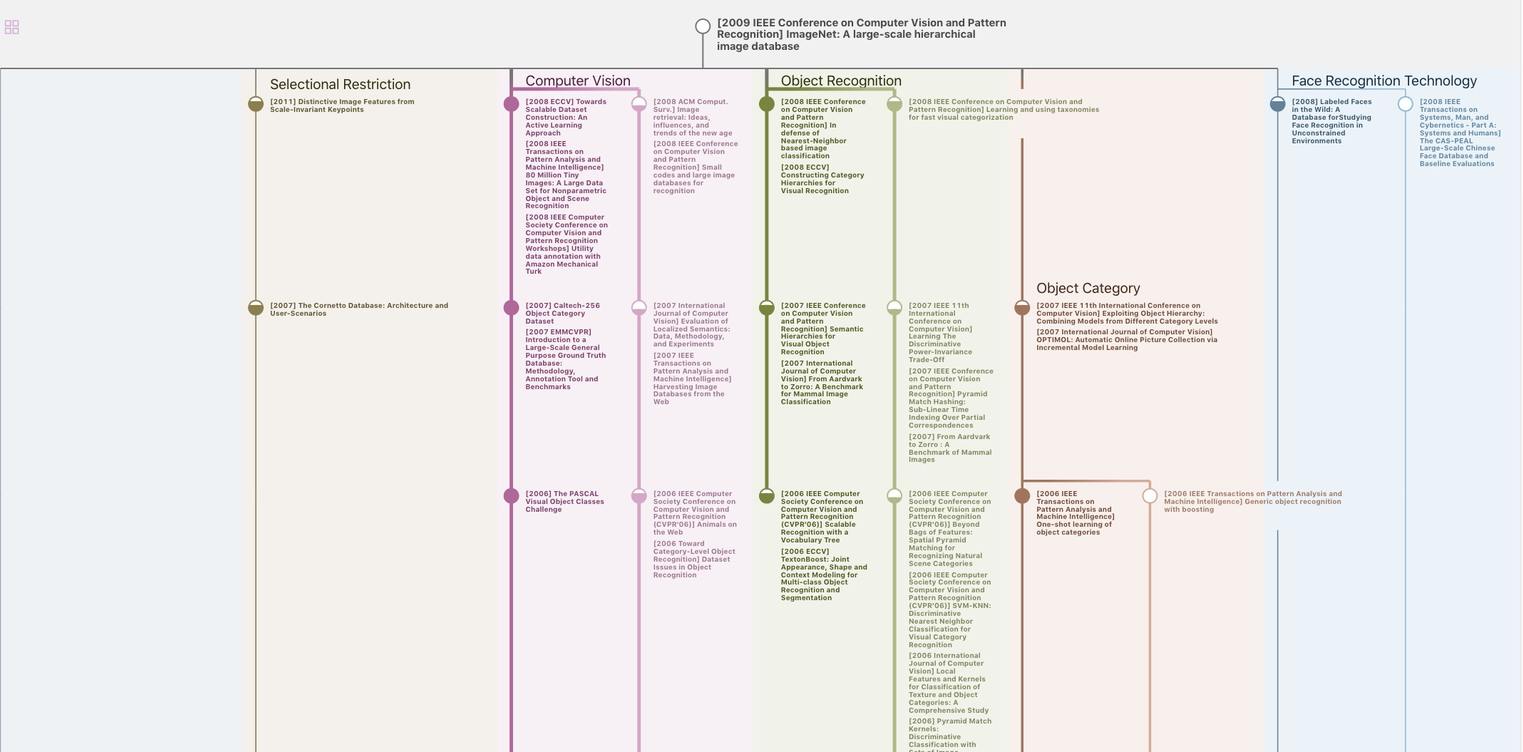
生成溯源树,研究论文发展脉络
Chat Paper
正在生成论文摘要