AUGER: A Multi-Objective Design Space Exploration Framework for CGRAs
2023 INTERNATIONAL CONFERENCE ON FIELD PROGRAMMABLE TECHNOLOGY, ICFPT(2023)
Abstract
Coarse-grained reconfigurable architectures (CGRAs) are gaining increasing attention as domain-specific accelerators due to their high flexibility and energy efficiency. CGRAs typically involve numerous design parameters, which results in a vast design space. Therefore, exploring a trade-off between various metrics, such as area and throughput, is challenging. Although many CGRA design space exploration (DSE) frameworks have been proposed to overcome this issue, a scalable and robust DSE framework that can achieve a trade-off between different metrics for a large design space is still lacking. In this paper, we propose AUGER, a multi-objective DSE framework for CGRAs. AUGER incorporates an unsupervised active learning-based sampling algorithm that maximizes both representativeness and diversity of initial samples. To enhance the robustness and scalability of AUGER, we construct a multiacquisition strategy and a hybrid surrogate model within the framework. Our approach demonstrates superior performance on two Pareto frontier metrics compared to state-of-the-art DSE algorithms on different datasets.
MoreTranslated text
Key words
Design space exploration,Bayesian optimization,Deep kernel learning,CGRA
AI Read Science
Must-Reading Tree
Example
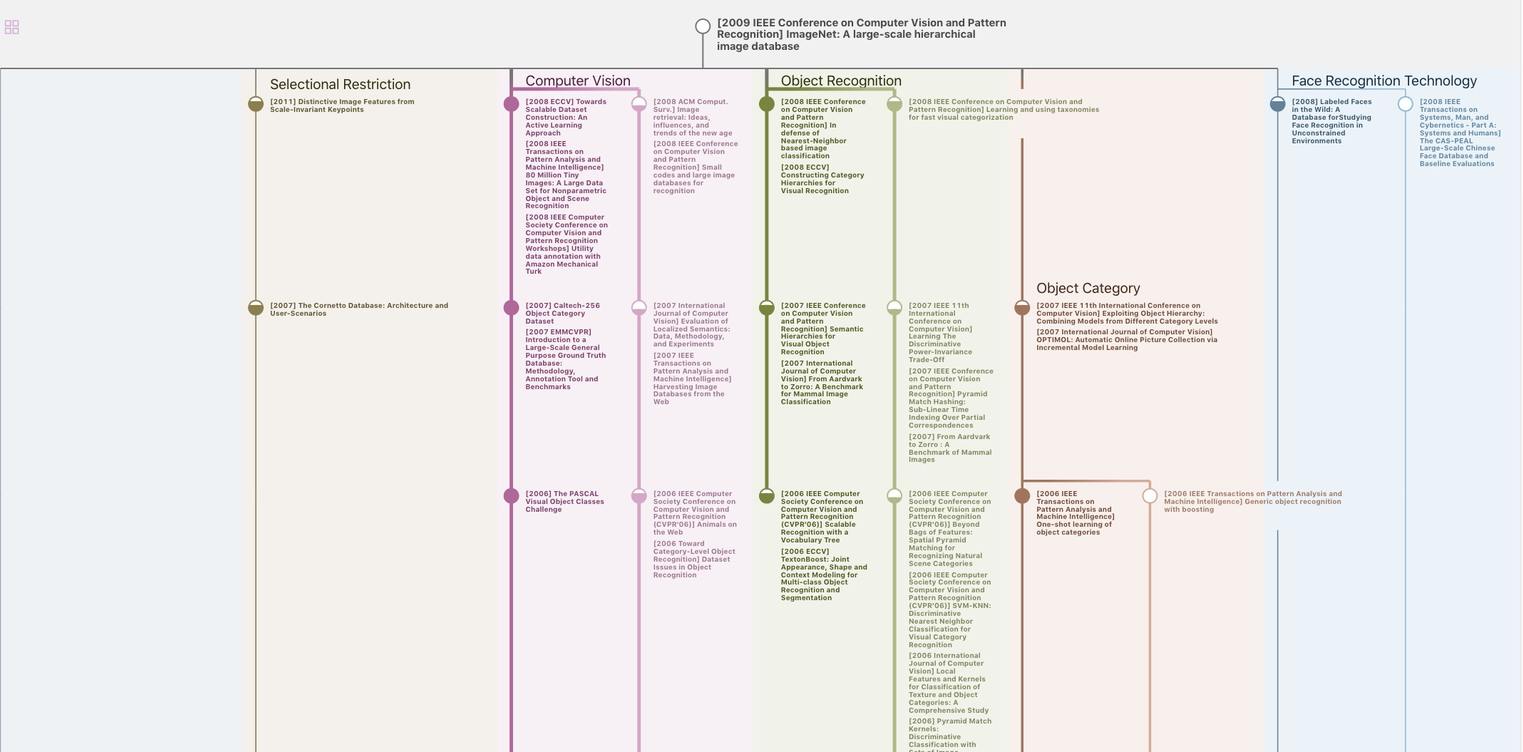
Generate MRT to find the research sequence of this paper
Chat Paper
Summary is being generated by the instructions you defined