Can you Remove the Downstream Model for Speaker Recognition with Self-Supervised Speech Features?
CoRR(2024)
摘要
Self-supervised features are typically used in place of filter-banks in
speaker verification models. However, these models were originally designed to
ingest filter-banks as inputs, and thus, training them on top of
self-supervised features assumes that both feature types require the same
amount of learning for the task. In this work, we observe that pre-trained
self-supervised speech features inherently include information required for
downstream speaker verification task, and therefore, we can simplify the
downstream model without sacrificing performance. To this end, we revisit the
design of the downstream model for speaker verification using self-supervised
features. We show that we can simplify the model to use 97.51
while achieving a 29.93
Consequently, we show that the simplified downstream model is more data
efficient compared to baseline–it achieves better performance with only 60
the training data.
更多查看译文
AI 理解论文
溯源树
样例
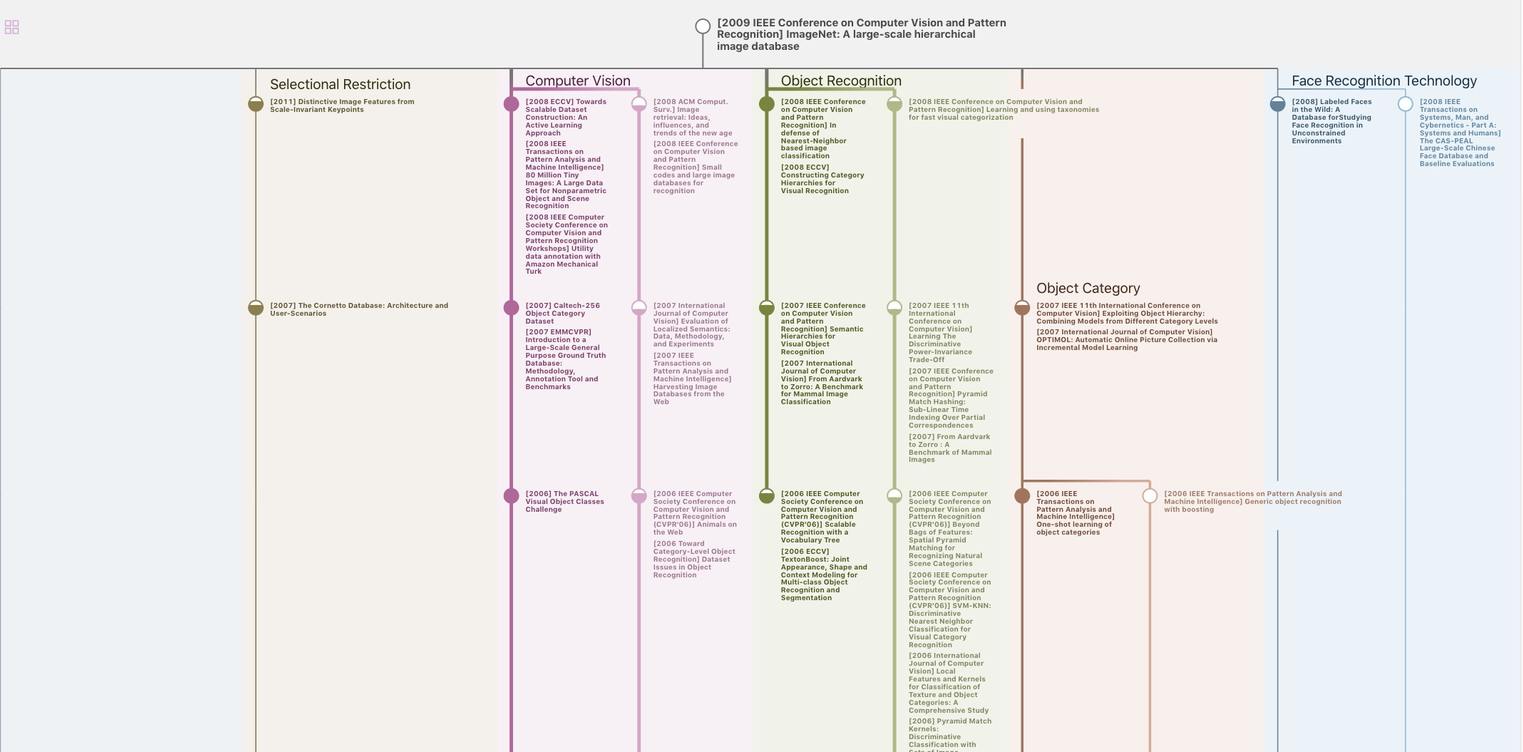
生成溯源树,研究论文发展脉络
Chat Paper
正在生成论文摘要