On the Choice of Loss Function in Learning-based Optimal Power Flow
CoRR(2024)
摘要
We analyze and contrast two ways to train machine learning models for solving
AC optimal power flow (OPF) problems, distinguished with the loss functions
used. The first trains a mapping from the loads to the optimal dispatch
decisions, utilizing mean square error (MSE) between predicted and optimal
dispatch decisions as the loss function. The other intends to learn the same
mapping, but directly uses the OPF cost of the predicted decisions, referred to
as decision loss, as the loss function. In addition to better aligning with the
OPF cost which results in reduced suboptimality, the use of decision loss can
circumvent feasibility issues that arise with MSE when the underlying mapping
from loads to optimal dispatch is discontinuous. Since decision loss does not
capture the OPF constraints, we further develop a neural network with a
specific structure and introduce a modified training algorithm incorporating
Lagrangian duality to improve feasibility.} This result in an improved
performance measured by feasibility and suboptimality as demonstrated with an
IEEE 39-bus case study.
更多查看译文
AI 理解论文
溯源树
样例
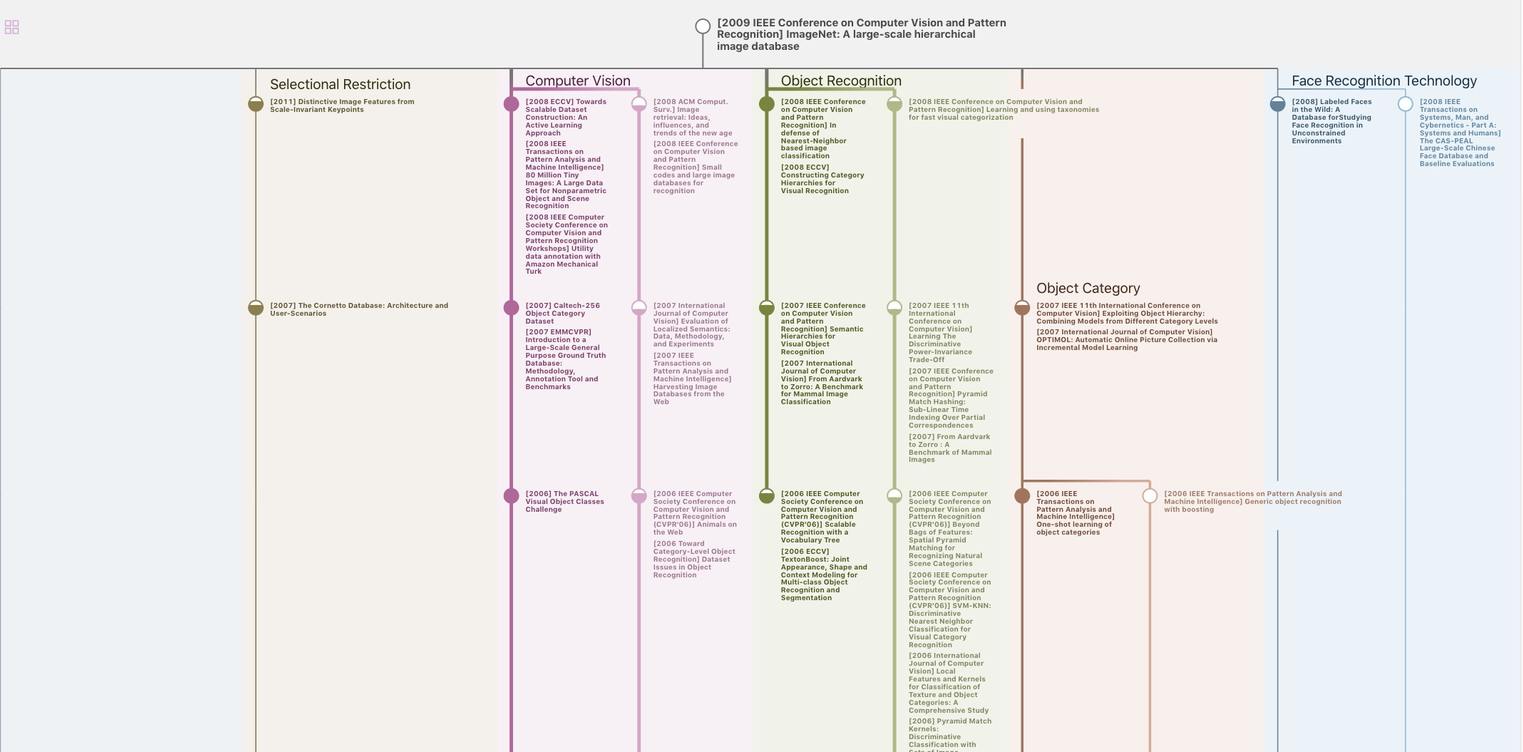
生成溯源树,研究论文发展脉络
Chat Paper
正在生成论文摘要