Statistical Model Checking based Analysis of Biological Networks
arXiv (Cornell University)(2018)
摘要
We introduce a framework for analyzing ordinary differential equation (ODE) models of biological networks using statistical model checking (SMC). A key aspect of our work is the modeling of single-cell variability by assigning a probability distribution to intervals of initial concentration values and kinetic rate constants. We propagate this distribution through the system dynamics to obtain a distribution over the set of trajectories of the ODEs. This in turn opens the door for performing statistical analysis of the ODE system's behavior. To illustrate this we first encode quantitative data and qualitative trends as bounded linear time temporal logic (BLTL) formulas. Based on this we construct a parameter estimation method using an SMC-driven evaluation procedure applied to the stochastic version of the behavior of the ODE system. We then describe how this SMC framework can be generalized to hybrid automata by exploiting the given distribution over the initial states and the--much more sophisticated--system dynamics to associate a Markov chain with the hybrid automaton. We then establish a strong relationship between the behaviors of the hybrid automaton and its associated Markov chain. Consequently, we sample trajectories from the hybrid automaton in a way that mimics the sampling of the trajectories of the Markov chain. This enables us to verify approximately that the Markov chain meets a BLTL specification with high probability. We have applied these methods to ODE based models of Toll-like receptor signaling and the crosstalk between autophagy and apoptosis, as well as to systems exhibiting hybrid dynamics including the circadian clock pathway and cardiac cell physiology. We present an overview of these applications and summarize the main empirical results. These case studies demonstrate that the our methods can be applied in a variety of practical settings.
更多查看译文
关键词
model checking,networks,statistical model
AI 理解论文
溯源树
样例
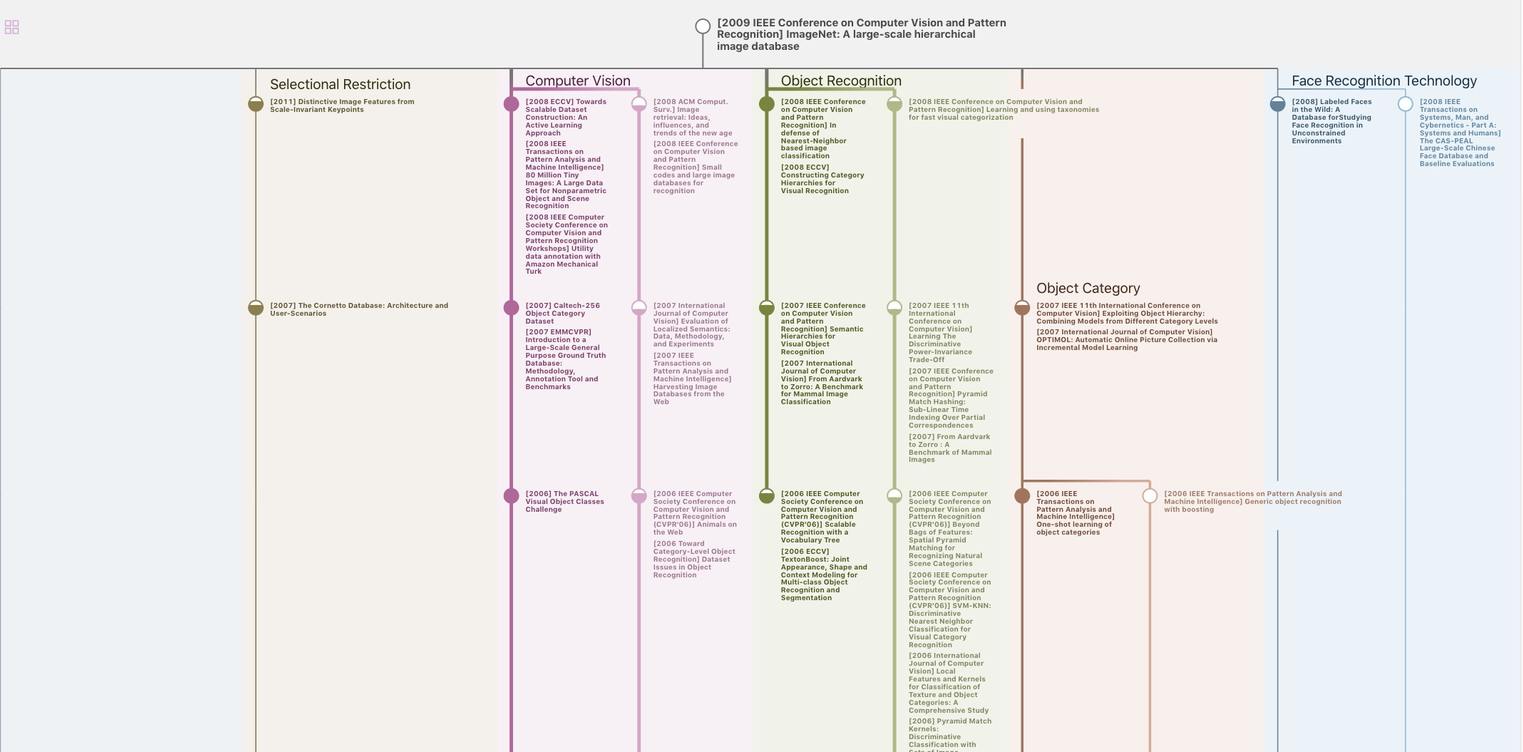
生成溯源树,研究论文发展脉络
Chat Paper
正在生成论文摘要