Quantifying the Importance of Non-Suicidal Self-Injury Characteristics in Predicting Different Clinical Outcomes: Using Random Forest Model.
Journal of youth and adolescence(2024)
Abstract
Existing research on non-suicidal self-injury (NSSI) among adolescents has primarily concentrated on general risk factors, leaving a significant gap in understanding the specific NSSI characteristics that predict diverse psychopathological outcomes. This study aims to address this gap by using Random Forests to discern the significant predictors of different clinical outcomes. The study tracked 348 adolescents (64.7% girls; mean age = 13.31, SD = 0.91) over 6 months. Initially, 46 characteristics of NSSI were evaluated for their potential to predict the repetition of NSSI, as well as depression, anxiety, and suicidal risks at a follow-up (T2). The findings revealed distinct predictors for each psychopathology. Specifically, psychological pain was identified as a significant predictor for depression, anxiety, and suicidal risks, while the perceived effectiveness of NSSI was crucial in forecasting its repetition. These findings imply that it is feasible to identify high-risk individuals by assessing key NSSI characteristics, and also highlight the importance of considering diverse NSSI characteristics when working with self-injurers.
MoreTranslated text
Key words
Non-suicidal self-injury,Depression,Anxiety,Suicide,Data mining,Random forest
AI Read Science
Must-Reading Tree
Example
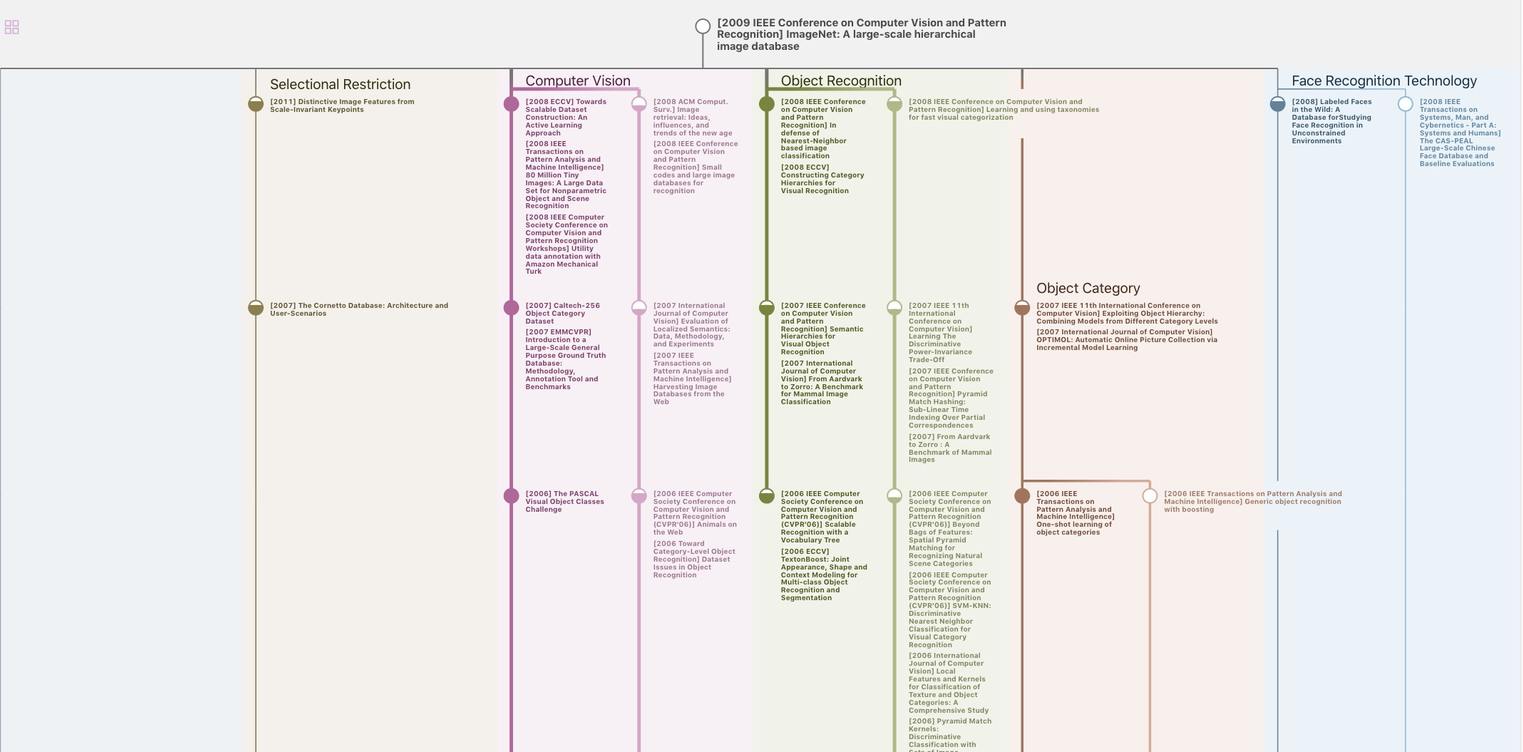
Generate MRT to find the research sequence of this paper
Chat Paper
Summary is being generated by the instructions you defined