Multiple Hybrid AI Model-based Optimal Charging Profile of Lithium-ion Battery for SOH Enhancement
2023 IEEE International Conference on Power Electronics, Smart Grid, and Renewable Energy (PESGRE)(2023)
Abstract
Battery is one of the major components of electric vehicles, which highly influences the performance of Electric Vehicles (EVs). However, enhancing the life of a Lithium-ion (Li-ion) battery is a challenging task because they have a high risk of fire hazards due to the electrochemical properties of Li-ion. Various key factors that have a significant influence on the health of the battery include a number of charge-discharge cycles, temperature, voltage, and current profiles. Hence, a highly efficient Battery Management System (BMS) with an optimal charging facility is needed. The main objective of the proposed work is to generate Multiple Hybrid Artificial Intelligence (MHAI)-based optimal charging current profiles for Li-ion batteries with minimum charging time and temperature rise in order to enhance the State Of Health (SOH). In this work, an open-source dataset of Li-ion-18650 from the National Aeronautics and Space Administration (NASA) was used. Firstly, the battery charging profile range from 0 to 100% is divided into 4 groups (0-25%, 26-50%, 51-75%,76-100%), and four hybrid AI models are developed and validated. to find the optimal charging current in each of the 4 regions instead of using a single AI model for the entire charging profile. For model development, temperature, maximum chargeable capacity, and charging time are considered as outputs, and charging voltage and current are taken as inputs. Long Short Term Memory (LSTM), Random Forest (RF), and Coulomb Counting (White Box Model) are used to develop models to predict temperature, maximum chargeable capacity, and charging time, respectively. Finally, Particle Swarm Optimization (PSO) is used to find the optimal current value for the developed models to minimize both the charging time and temperature rise using the weighted-sum method of the MultiObjective Particle Swarm Optimization (MOPSO) technique. The results show the feasibility of the proposed approach.
MoreTranslated text
Key words
MHAI,SOH,LSTM,data-driven,PSO,optimal charging,MOPSO
AI Read Science
Must-Reading Tree
Example
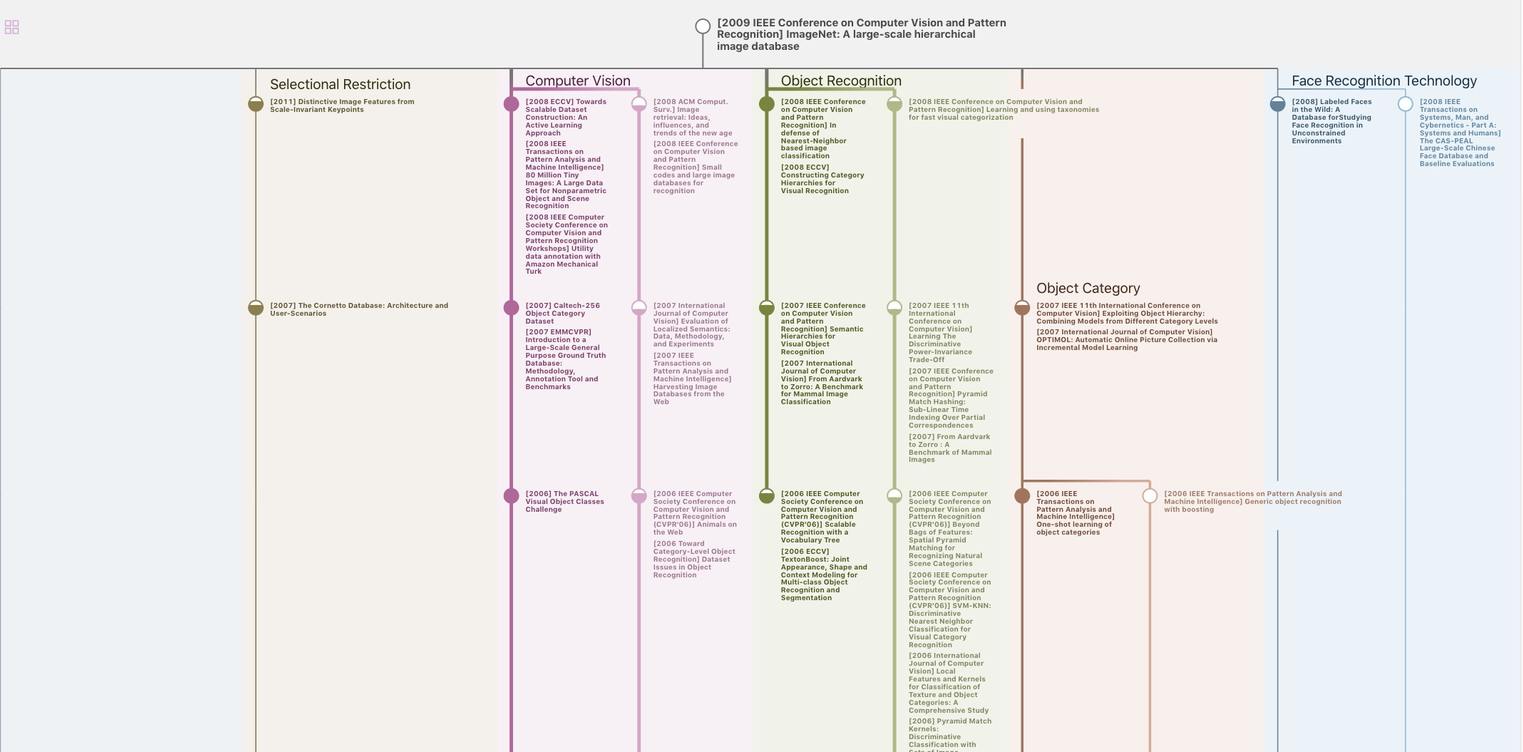
Generate MRT to find the research sequence of this paper
Chat Paper
Summary is being generated by the instructions you defined