Design Space Exploration Tool for Mixed-Signal Spiking Neural Network.
Midwest Symposium on Circuits and Systems(2023)
摘要
Spiking Neural Networks (SNNs) are biologically inspired neural network algorithms that process data based on events. Neuromorphic hardware is used to compute SNNs which enables significant energy efficiency. Specifically, mixed-signal circuits such as analog synapses and mixed-signal neurons are used to closely emulate biological neurons and synapses and also to compute the SNNs at low power. However, the mixed-signal implementation of spiking neural networks (SNNs) involves non-linear computation and process variation. This study presents a tool to model these nonidealities while learning the parameters of the SNNs. Currently, the tool allows exploring different network architectures (Fully-Connected and Recurrent) with two hardware-aware neuron models and Floating-Gate (FG) transistor-based synapses. Further, it enables users to train the SNNs using biologically inspired local learning rules while using spiking datasets. The tool estimates the network's area, power consumption, and accuracy based on the measurement and simulations performed in the 65nm CMOS process.
更多查看译文
关键词
Neural Network,Spiking Neural Networks,Design Space Exploration,Power Consumption,Bioinspired,Neuron Model,Learning Rule,Local Rules,CMOS Process,Neuromorphic Hardware,Learning Algorithms,Temporal Dynamics,Neurons In Layer,Voltage Source,Neuronal Firing,Field-effect Transistors,Threshold Voltage,Metal Oxide Semiconductor,Random Matrix,Leaky Integrate-and-fire,Input Capacitance,Spike-timing-dependent Plasticity,Spike Trains,Hot Electrons,Spike Frequency Adaptation,MNIST Dataset,Drain Voltage,Accuracy Metrics,Area Estimation
AI 理解论文
溯源树
样例
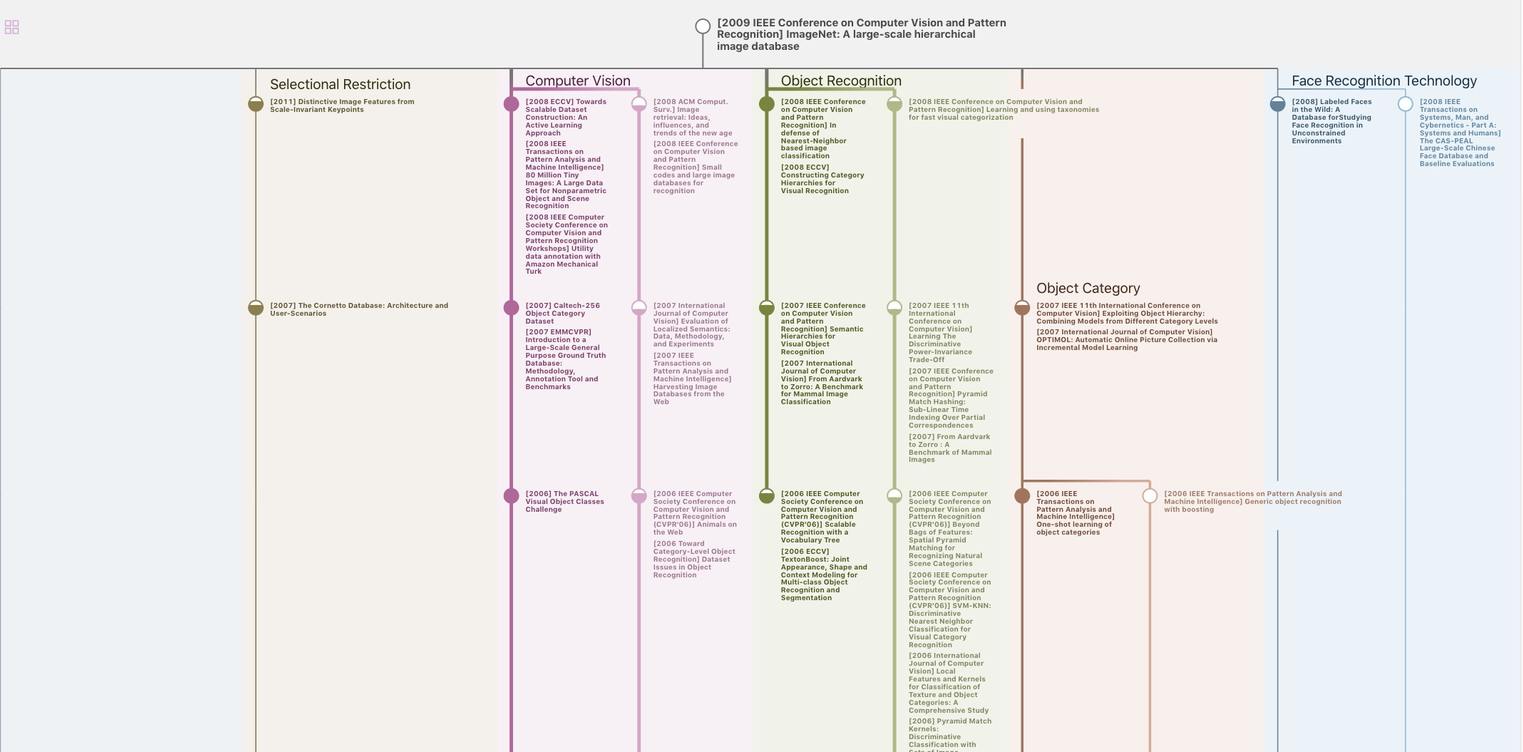
生成溯源树,研究论文发展脉络
Chat Paper
正在生成论文摘要