Representative Calibration Using Black-Box Optimization and Clustering.
Winter Simulation Conference(2023)
摘要
Calibration is a crucial step for model validity, yet its representation is often disregarded. This paper proposes a two-stage approach to calibrate a model that represents target data by identifying multiple diverse parameter sets while remaining computationally efficient. The first stage employs a black-box optimization algorithm to generate near-optimal parameter sets, the second stage clusters the generated parameter sets. Five black-box optimization algorithms, namely, Latin Hypercube Sampling (LHS), Sequential Model-based Algorithm Configuration (SMAC), Optuna, Simulated Annealing (SA), and Genetic Algorithm (GA), are tested and compared using a disease-opinion compartmental model with predicted health outcomes. Results show that LHS and Optuna allow more exploration and capture more variety in possible future health outcomes. SMAC, SA, and GA, are better at finding the best parameter set but their sampling approach generates less diverse model outcomes. This two-stage approach can reduce computation time while producing robust and representative calibration.
更多查看译文
关键词
Black-box Optimization,Health Outcomes,Computation Time,Computational Efficiency,Optimization Algorithm,Simulated Annealing,Target Data,Two-stage Approach,Sequential Algorithm,Latin Hypercube Sampling,Future Health Outcomes,Black Box Algorithms,Evaluation Of Function,Multi-agent,Mean Absolute Error,Sum Of Squares,Infected Population,Clustering Results,Range Of Settings,Hyperparameter Tuning,Total Sum Of Squares,Single Set Of Parameters,Future Trajectories,Calibration Period,Within-cluster Sum Of Squares,Dead Population,Goodness Of Fit Measures,Good Point,Actual Point,Bayesian Optimization
AI 理解论文
溯源树
样例
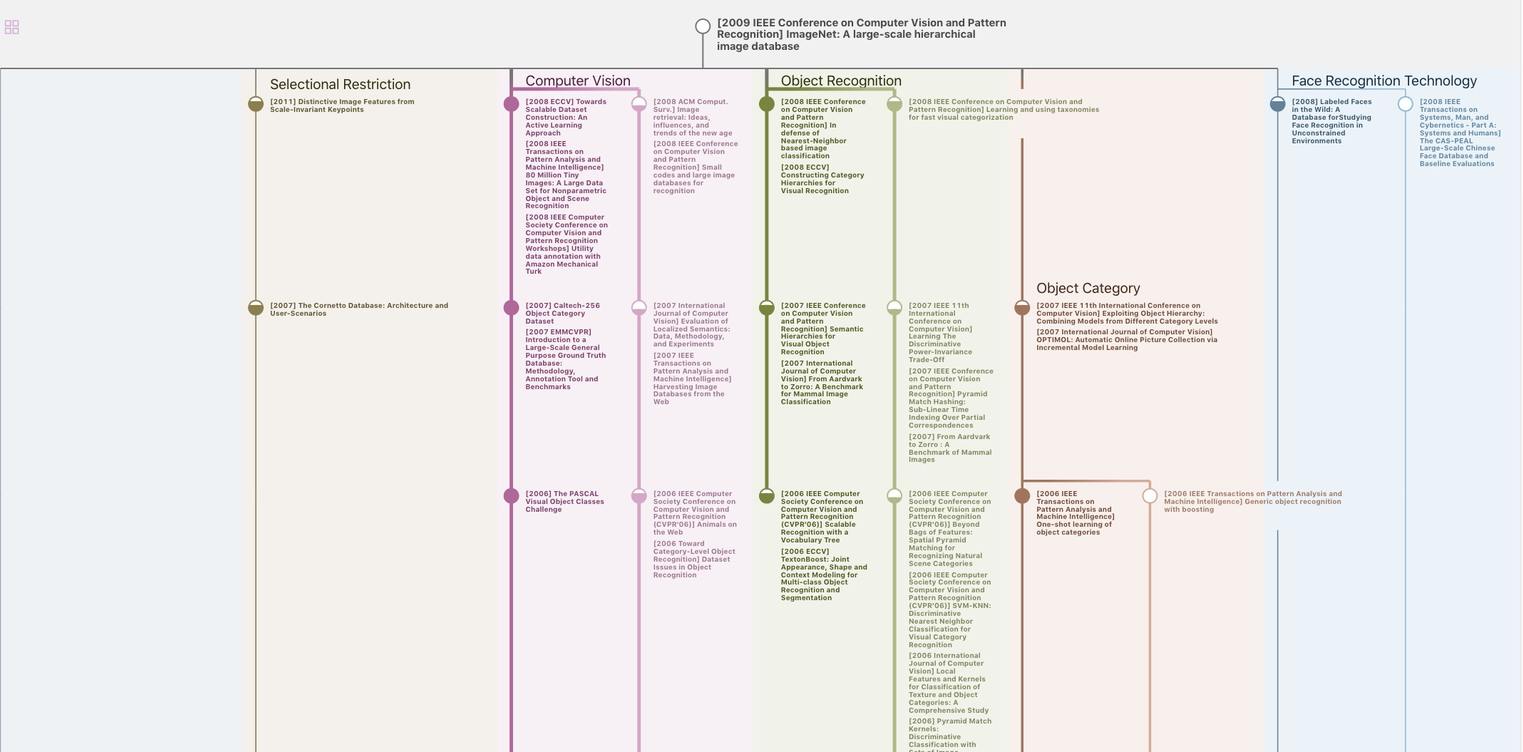
生成溯源树,研究论文发展脉络
Chat Paper
正在生成论文摘要