GREAT- IQA: Integrating Global Perception and Local Task-Specific Information for CT Image Quality Assessment
2023 IEEE International Conference on Medical Artificial Intelligence (MedAI)(2023)
摘要
Image quality assessment (IQA) is a crucial step for computed tomography (CT) system design and scanning protocol optimization. The lack of high-quality/high-dose and low-quality/low-dose CT image pairs makes only the no-reference IQA (NR-IQA) method available for clinical practice. Recently, deep learning (DL) techniques have been proven effective for CT IQA. However, applying DL-based methods to CT IQA faces two major challenges: 1) labels are noisy due to inter-observer variability and 2) IQA results fail to reflect the task-specific performance of CT images perfectly. In this work, we presented a novel NR-IQA model to integrate Global peRcEption and locAl Task-specific information for CT images, termed GREAT-IQA. First, we used a convolutional neural network to learn global perception-related features from training data, which leverages two objective IQA metrics, i.e. peak signal-to-noise ratio and structural similarity index, to reduce the inter-observer variability. Second, to more precisely quantify the task-specific performance of CT images, we considered two local diagnosis-related features of the chest and abdominal CT images: low-contrast detectability and edge sharpness, where contrast-to-noise ratio and maximum gradient and variability of gradients are used to measure them separately. Finally, the overall image quality is predicted by combining the global image assessment and local region of interest assessment components. By conducting experiments on a total of 1,600 degraded chest and abdominal CT images, reconstructed retrospectively with different doses and kernels, and evaluated with three experienced experts, this paper shows the proposed method outperforms the competing IQA methods, especially full-reference IQA methods.
更多查看译文
关键词
Computed tomography,image quality assessment,no-reference,convolutional neural network,inter-observer variability,task-specific performance assessment
AI 理解论文
溯源树
样例
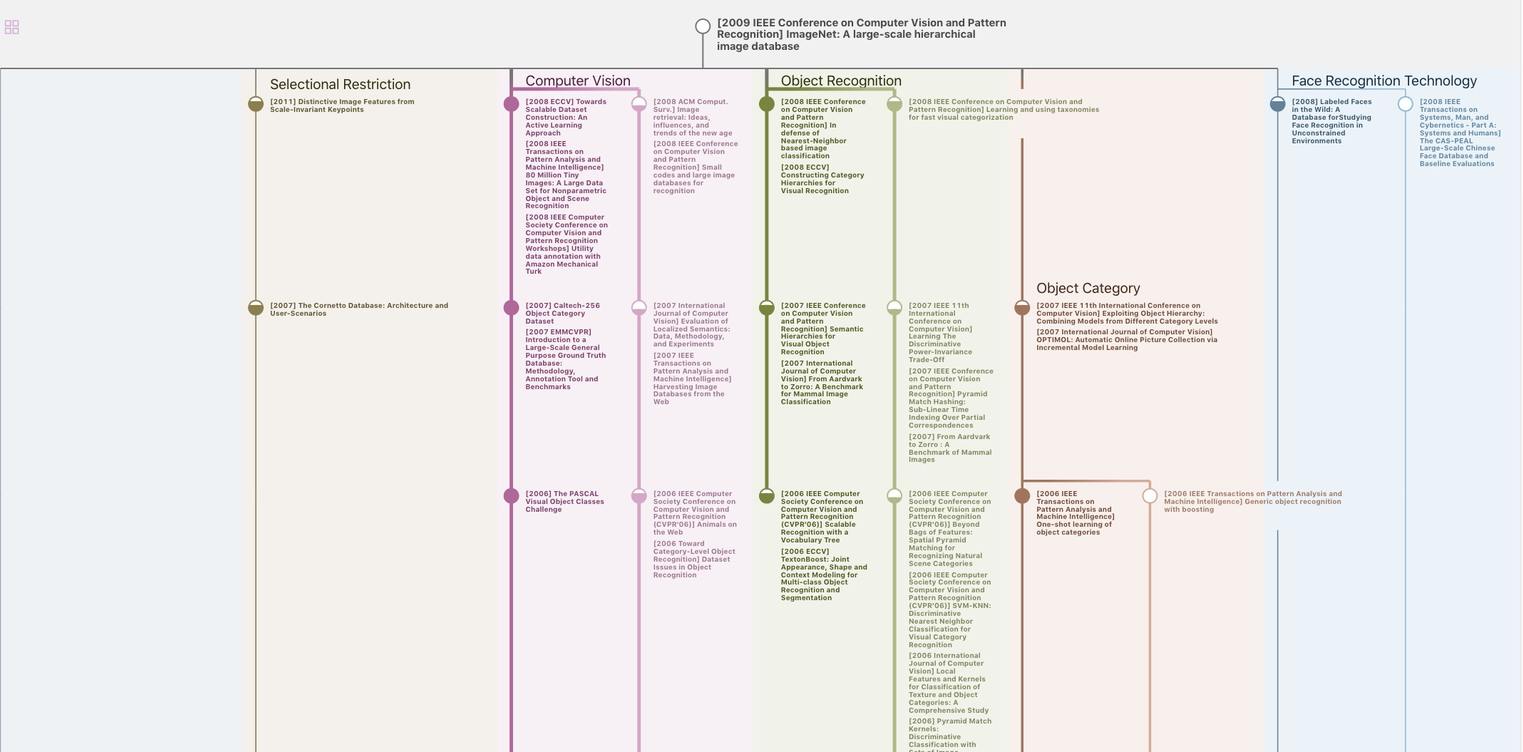
生成溯源树,研究论文发展脉络
Chat Paper
正在生成论文摘要