Causal Dynamic Bayesian Networks for Simulation Metamodeling.
Winter Simulation Conference(2023)
摘要
A traditional metamodel for a discrete-event simulation approximates a real-valued performance measure as a function of the input-parameter values. We introduce a novel class of metamodels based on modular dynamic Bayesian networks (MDBNs), a subclass of probabilistic graphical models which can be used to efficiently answer a rich class of probabilistic and causal queries (PCQs). Such queries represent the joint probability distribution of the system state at multiple time points, given observations of, and interventions on, other state variables and input parameters. This paper is a first demonstration of how the extensive theory and technology of causal graphical models can be used to enhance simulation metamodeling. We demonstrate this potential by showing how a single MDBN for an M/M/1 queue can be learned from simulation data and then be used to quickly and accurately answer a variety of PCQs, most of which are out-of-scope for existing metamodels.
更多查看译文
关键词
Bayesian Model,Dynamic Network,Dynamic Bayesian Network,Simulated Data,System State,State Variables,Input Parameters,Graphical Model,Discrete Event Simulation,Probabilistic Graphical Models,Stationary Probability Distribution,Training Data,Simulation Model,State Space,Timing Of Events,Conditional Probability,Conditional Distribution,Length Distribution,Service Rate,Family Of Models,Arrival Rate,Queue Length,Conditional Probability Distribution,Time Slice,Set Of Transformations,Simulation Run,Exact Inference,Subset Of Variables,Continuous-time Process,Stochastic Simulations
AI 理解论文
溯源树
样例
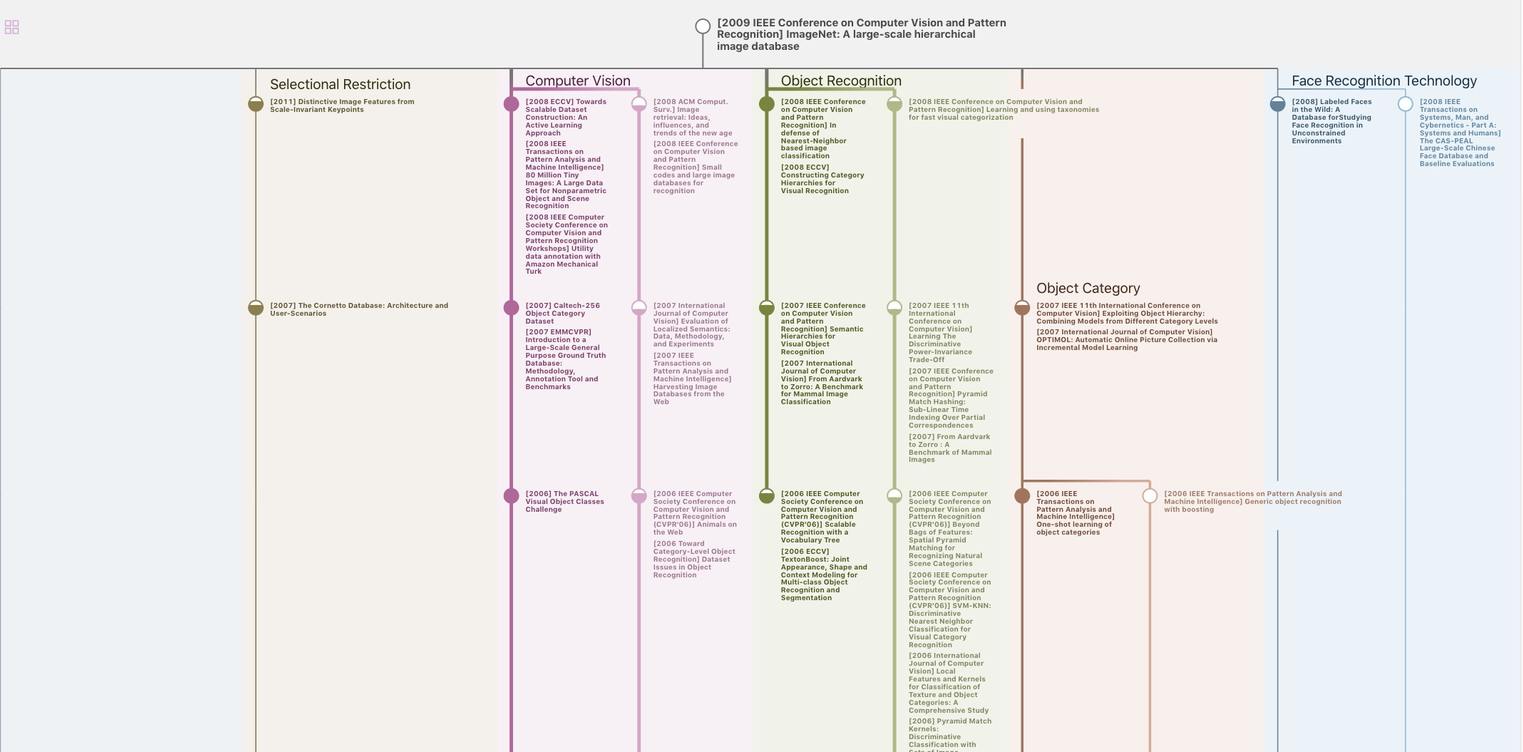
生成溯源树,研究论文发展脉络
Chat Paper
正在生成论文摘要