Optimal Batching Under Computation Budget.
Winter Simulation Conference(2023)
摘要
Batching methods operate by dividing data into batches and conducting inference by aggregating estimates from batched data. These methods have been used extensively in simulation output analysis and, among other strengths, an advantage is the light computation cost when using a small number of batches. However, under computation budget constraints, it is open to our knowledge which batching approach among the range of alternatives is statistically optimal, which is important in guiding procedural configuration. We show that standard batching, but also certain carefully designed schemes using uneven-size batches or overlapping batches, are large-sample optimal in the sense of so-called uniformly most accurate unbiasedness from a dual view of hypothesis testing.
更多查看译文
关键词
Hypothesis Testing,Batch Method,Null Hypothesis,Variance Estimates,Point Estimates,Batch Size,Proof Of Theorem,Empirical Distribution,Asymptotic Distribution,Regularity Conditions,Central Limit Theorem,Model Run,Input Distribution,Nuisance Parameters,Converges In Distribution,Standard T-test,Proof Sketch,Unbiased Test,Asymptotically Unbiased
AI 理解论文
溯源树
样例
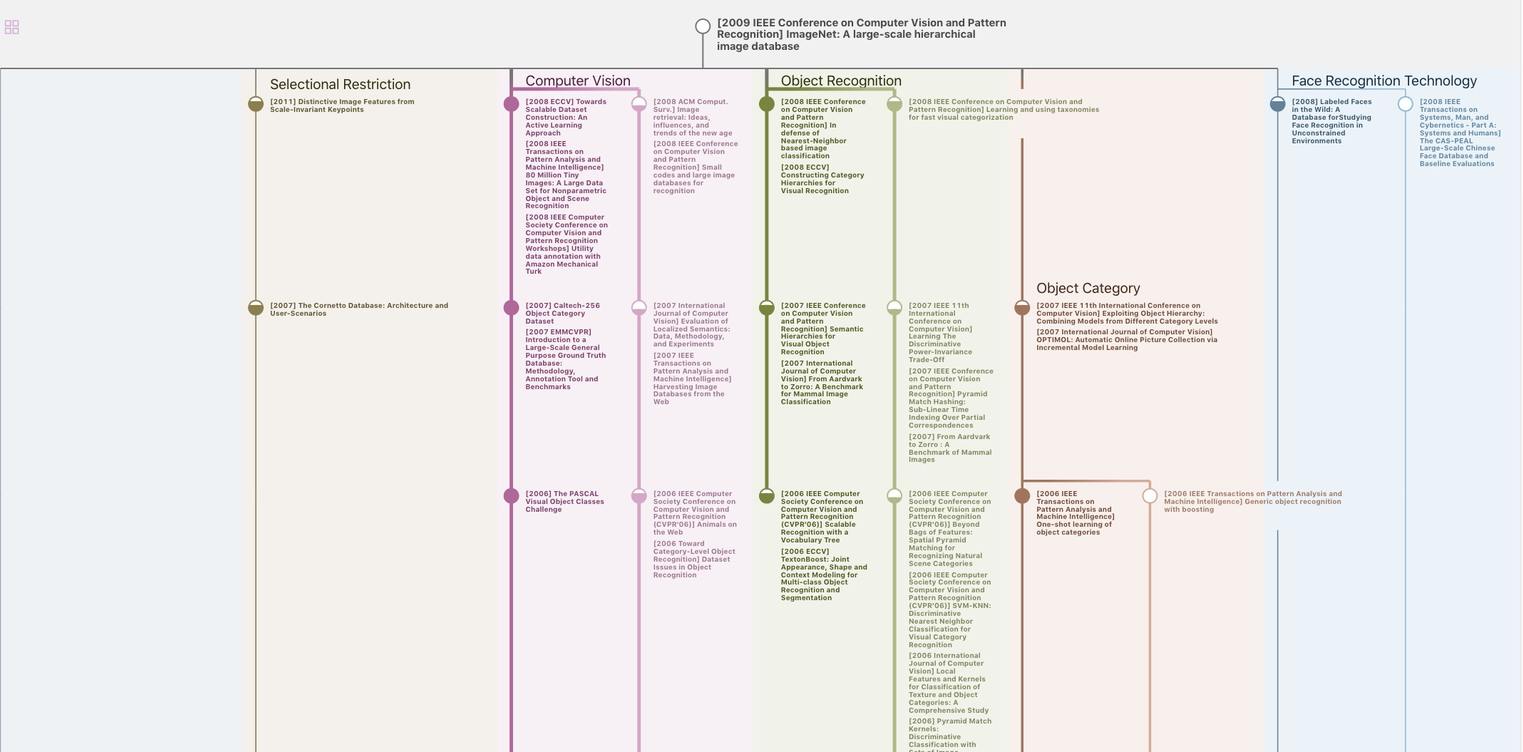
生成溯源树,研究论文发展脉络
Chat Paper
正在生成论文摘要