Local Feature Semantic Alignment Network for Few-Shot Image Classification
MULTIMEDIA TOOLS AND APPLICATIONS(2024)
关键词
Few-shot learning,Metric learning,Local feature,Semantic alignment
AI 理解论文
溯源树
样例
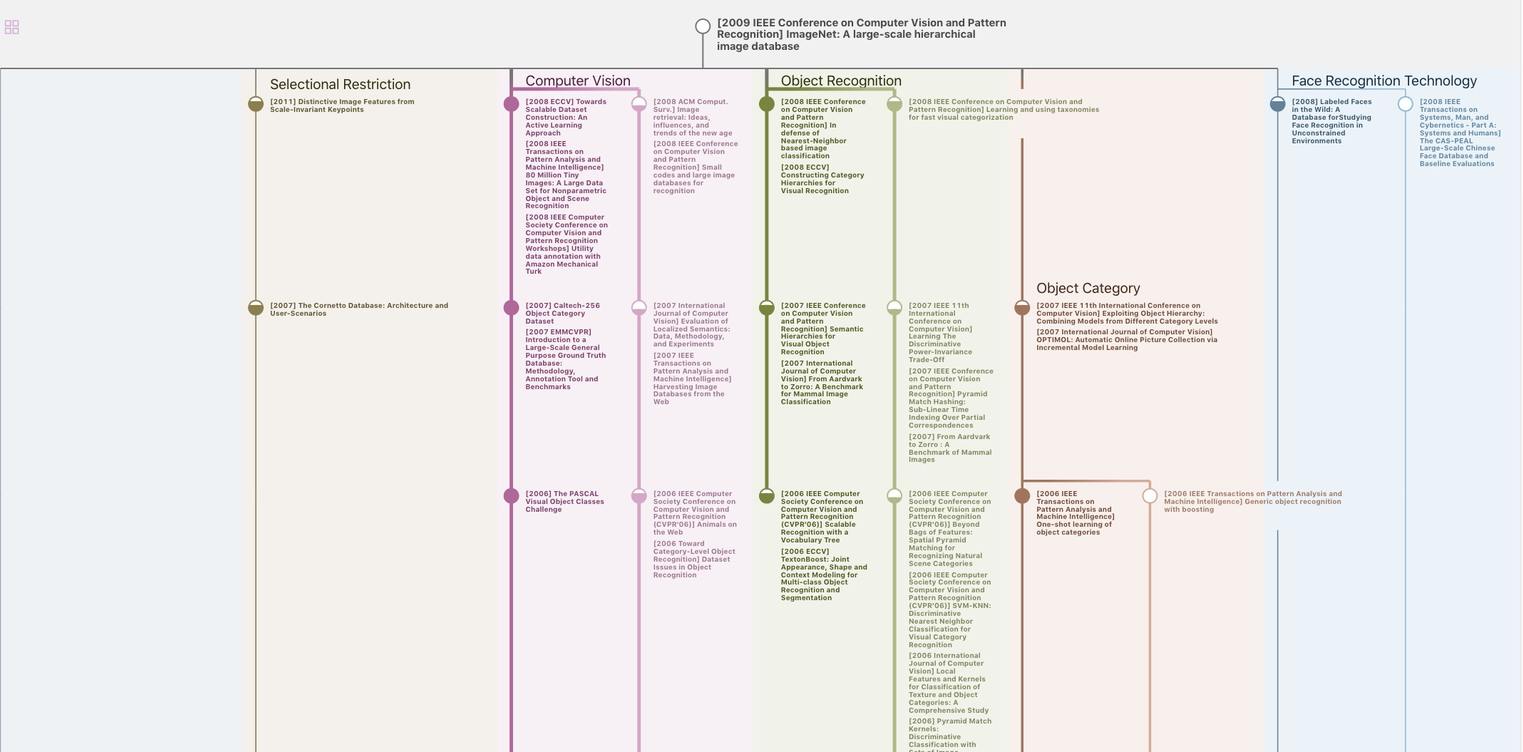
生成溯源树,研究论文发展脉络
Chat Paper
正在生成论文摘要
MULTIMEDIA TOOLS AND APPLICATIONS(2024)