Semantic and geometric information propagation for oriented object detection in aerial images
Applied Intelligence(2024)
Abstract
Unlike the natural scenes, aerial objects are often in arbitrary orientations and surrounded by cluttered backgrounds, causing the heterogeneous object features contaminated with backgrounds and interleaved clustered objects. Though segmentation denoising has been proposed to enhance arbitrary-oriented object detection in previous studies, the typical semantic segmentation brings ambiguous background information and ignores the significant geometric information propagation. In this paper, we explore the non-monotonic impacts of segmentation on object detection. Based on this, we propose a novel aerial object detector named PCG-Net to enhance the semantic and geometric information, as well as, alleviate the noise interference through segmentation guidance. PCG-Net includes a pseudo-siamese multi-scale relation module (PSM), a complementary segmentation map (CSM) and a global context module with adaptive distinction(GCMA). PSM captures the critical geometric and semantic features through parallel horizontal and oriented box-wise segmentation branches with interaction. As the guidance of each branch in PSM, CSM synergizes binary segmentation map and foreground semantic segmentation map so as to refine the semantic information and restrain the ambiguous background simultaneously. Based on the segmentation features from PSM, GCMA rebuilds and complements the global contextual information with pixel-level discrimination. Through exploiting the rich semantic, geometric and contextual information, the proposed framework enhances the feature representation powerfully in aerial images and suppresses noise interference effectively. Experiment results on three challenging aerial datasets including DOTA, HRSC2016 and DIOR-R, demonstrate the effectiveness of our approach. Graphical abstract
MoreTranslated text
Key words
Aerial images,Object detection,Arbitrary orientation,Feature enhancement,Feature denoising
AI Read Science
Must-Reading Tree
Example
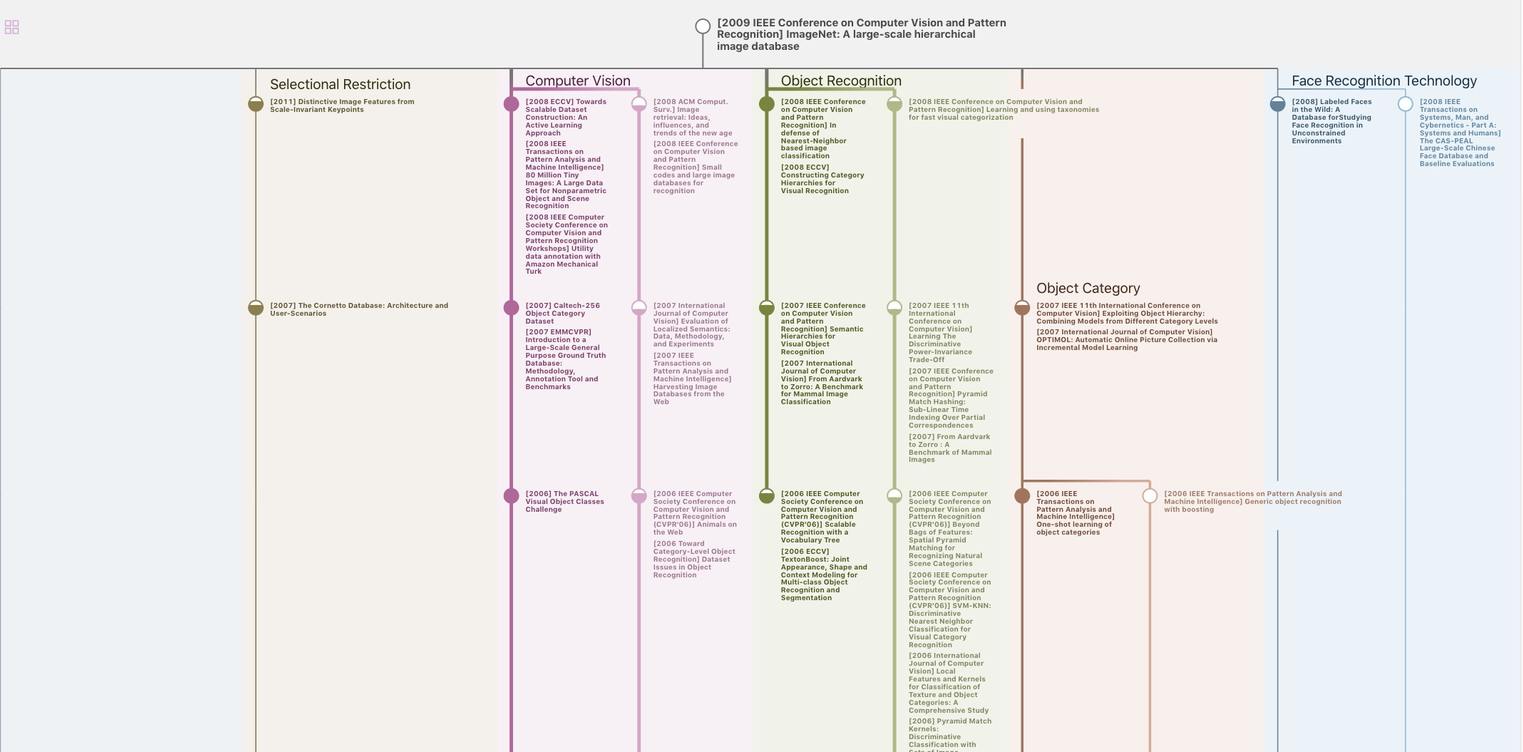
Generate MRT to find the research sequence of this paper
Chat Paper
Summary is being generated by the instructions you defined