DLM-DTI: a dual language model for the prediction of drug-target interaction with hint-based learning
Journal of Cheminformatics(2024)
摘要
The drug discovery process is demanding and time-consuming, and machine learning-based research is increasingly proposed to enhance efficiency. A significant challenge in this field is predicting whether a drug molecule’s structure will interact with a target protein. A recent study attempted to address this challenge by utilizing an encoder that leverages prior knowledge of molecular and protein structures, resulting in notable improvements in the prediction performance of the drug-target interactions task. Nonetheless, the target encoders employed in previous studies exhibit computational complexity that increases quadratically with the input length, thereby limiting their practical utility. To overcome this challenge, we adopt a hint-based learning strategy to develop a compact and efficient target encoder. With the adaptation parameter, our model can blend general knowledge and target-oriented knowledge to build features of the protein sequences. This approach yielded considerable performance enhancements and improved learning efficiency on three benchmark datasets: BIOSNAP, DAVIS, and Binding DB. Furthermore, our methodology boasts the merit of necessitating only a minimal Video RAM (VRAM) allocation, specifically 7.7GB, during the training phase (16.24% of the previous state-of-the-art model). This ensures the feasibility of training and inference even with constrained computational resources.
更多查看译文
关键词
Drug-target interactions,Pre-trained language model,Knowledge adaptation,Lightweight framework
AI 理解论文
溯源树
样例
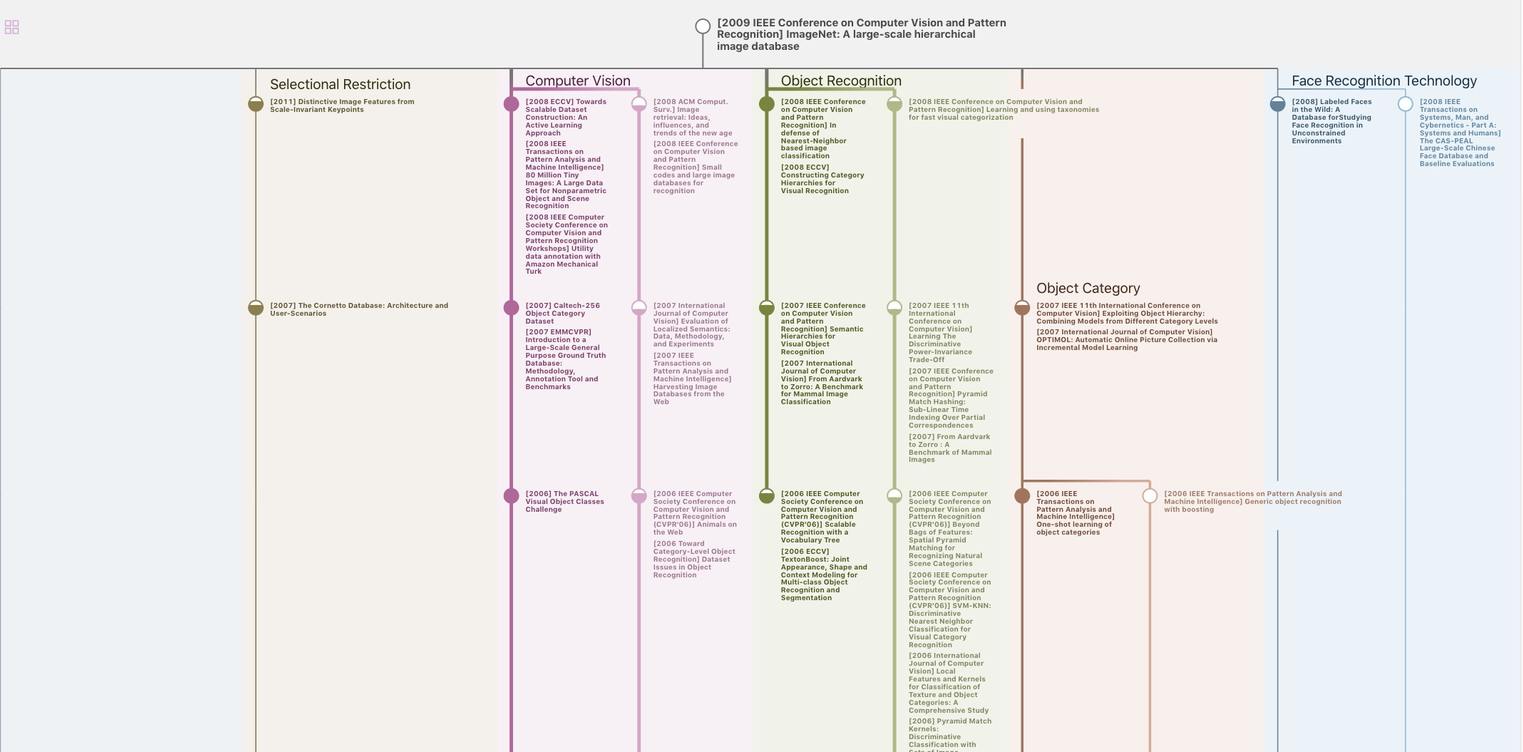
生成溯源树,研究论文发展脉络
Chat Paper
正在生成论文摘要