Replicating enzymatic activity by positioning active sites with synthetic protein scaffolds
biorxiv(2024)
摘要
Evolutionary constraints significantly limit the diversity of naturally occurring enzymes, thereby reducing the sequence repertoire available for enzyme discovery and engineering. Recent breakthroughs in protein structure prediction and de novo design, powered by artificial intelligence, now enable us to create enzymes with desired functions without relying on traditional genome mining. Here, we demonstrate a computational strategy for creating new-to-nature PET hydrolases by leveraging the known catalytic mechanisms and implementing multiple deep learning algorithms and molecular computations. This strategy includes the extraction of functional motifs from a template enzyme (here we use leaf-branch compost cutinase, LCC), regeneration of new protein scaffolds, computational screening, experimental validation, and sequence refinement. We successfully replicate PET hydrolytic activity with designer enzymes that are at least 30% shorter in sequence length than LCC. Among them, RsPETase 1 stands out due to its robust expressibility. It exhibits comparable activity to IsPETase and considerable thermostability with a melting temperature of 56 °C, despite sharing only 34% sequence similarity with LCC. This work suggests that enzyme diversity can be expanded by recapitulating functional motifs with computationally built protein scaffolds, thus generating opportunities to acquire highly active and robust enzymes that do not exist in nature.
### Competing Interest Statement
The authors have declared no competing interest.
更多查看译文
AI 理解论文
溯源树
样例
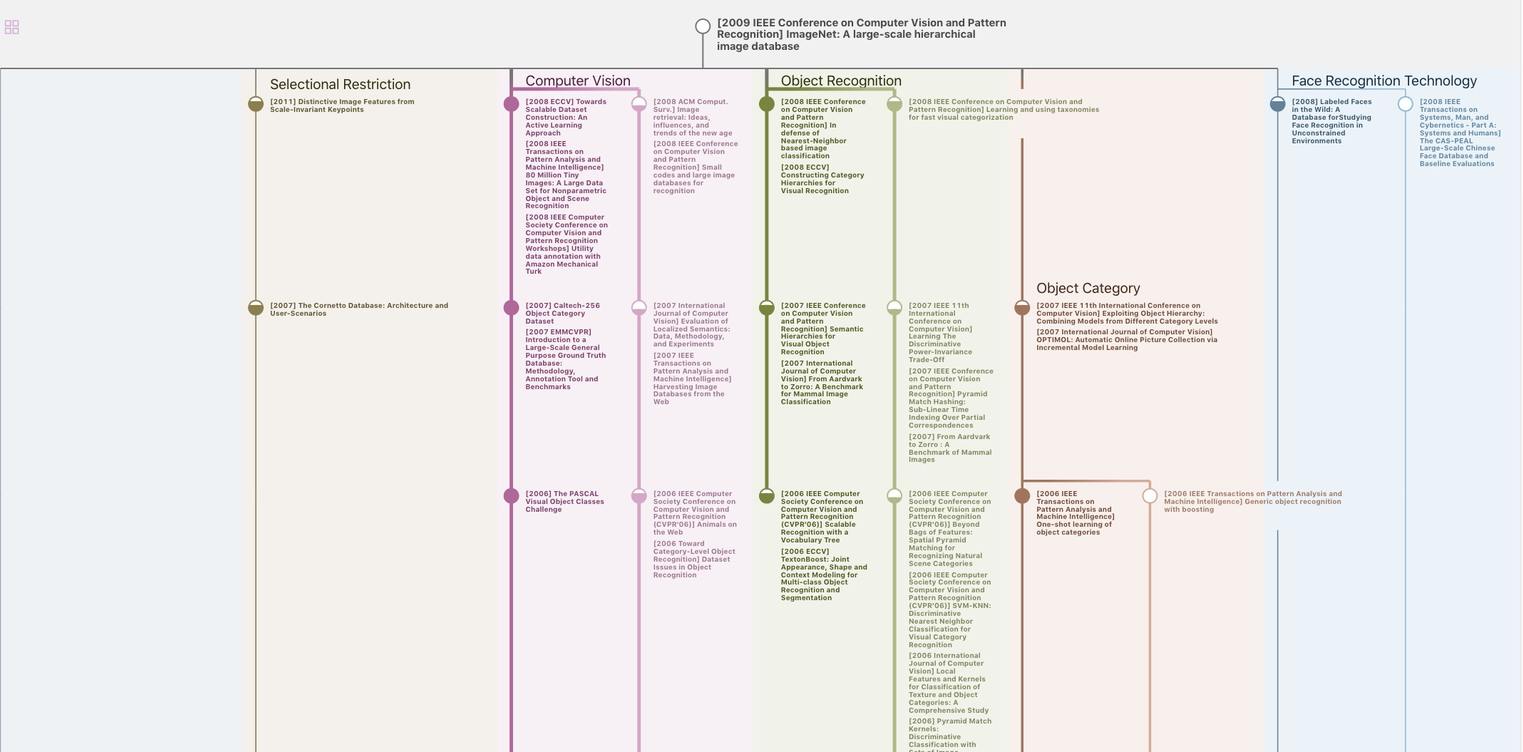
生成溯源树,研究论文发展脉络
Chat Paper
正在生成论文摘要