Recent methods from statistical inference and machine learning to improve integrative modeling of macromolecular assemblies
arxiv(2024)
摘要
Integrative modeling of macromolecular assemblies allows for structural
characterization of large assemblies that are recalcitrant to direct
experimental observation. A Bayesian inference approach facilitates combining
data from complementary experiments along with physical principles, statistics
of known structures, and prior models, for structure determination. Here, we
review recent methods for integrative modeling based on statistical inference
and machine learning. These methods improve over the current state-of-the-art
by enhancing the data collection, optimizing coarse-grained model
representations, making scoring functions more accurate, sampling more
efficient, and model analysis more rigorous. We also discuss three new
frontiers in integrative modeling: incorporating recent deep learning-based
methods, integrative modeling with in situ data, and metamodeling.
更多查看译文
AI 理解论文
溯源树
样例
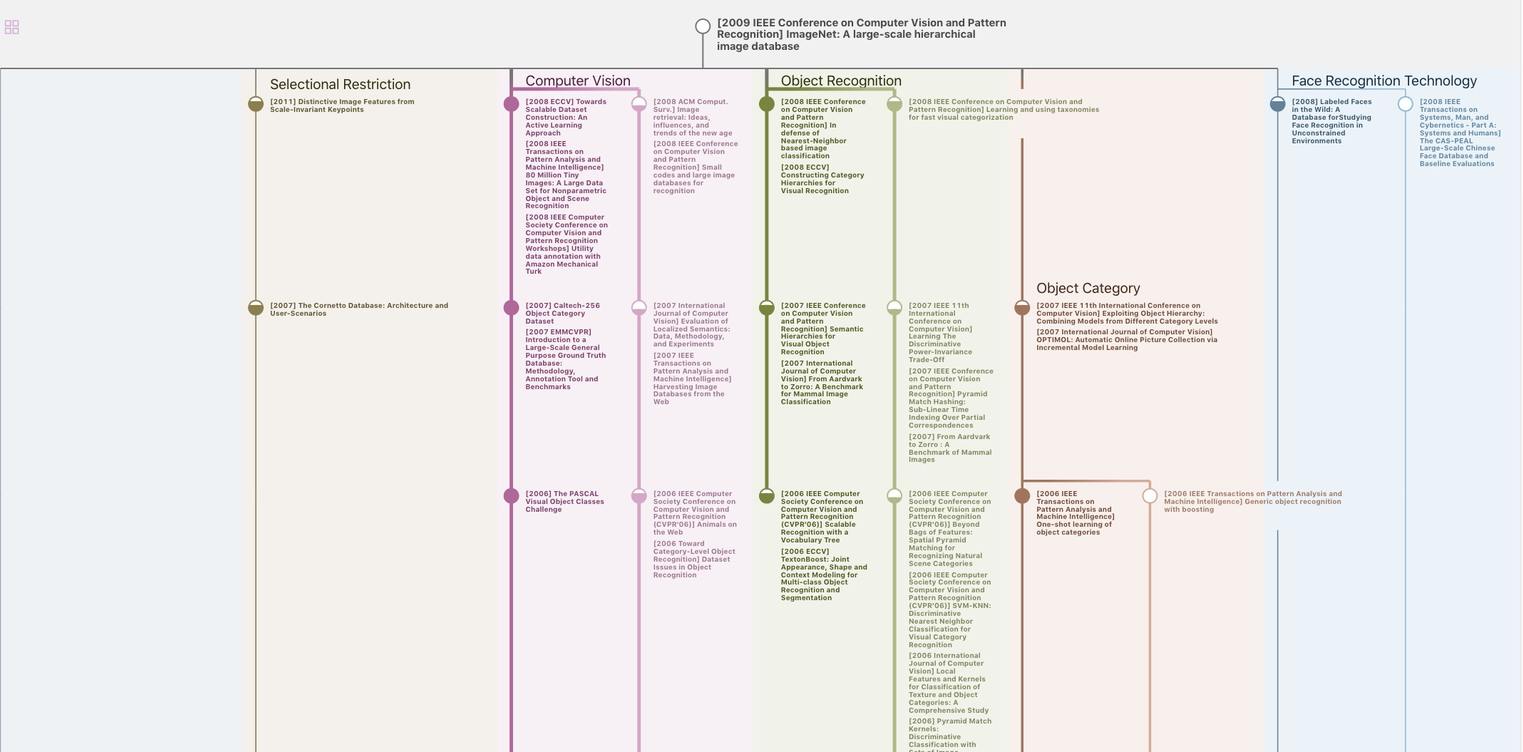
生成溯源树,研究论文发展脉络
Chat Paper
正在生成论文摘要