Scenario Predict-then-Optimize for Data-Driven Online Inventory Routing
arxiv(2024)
摘要
The real-time joint optimization of inventory replenishment and vehicle
routing is essential for cost-efficiently operating one-warehouse,
multiple-retailer systems. This is complex, as future demand predictions should
capture correlation retailer demand, and based upon such predictions,
replenishment and routing decisions must be taken. Traditionally, such
decisions are made by either making distributional assumptions or using
machine-learning-based point forecasts. The former approach ignores
nonstationary demand patterns, while the latter approach only provides a point
forecast ignoring the inherent forecast error. Consequently, in practice,
service levels often do not meet their targets, and truck fill rates fall
short, harming the efficiency and sustainability of daily operations. We
propose Scenario Predict-then-Optimize. This fully data-driven approach for
online inventory routing consists of two subsequent steps at each real-time
decision epoch. The scenario-predict step exploits neural networks,
specifically multi-horizon quantile recurrent neural networks, to predict
future demand quantiles, upon which we design a scenario sampling approach. The
subsequent scenario-optimize step then solves a scenario-based stochastic
programming approximation. Results show that our approach outperforms the
classic Predict-then-Optimize paradigm and empirical sampling methods. We show
this both on synthetic data and real-life data. Our approach is appealing to
practitioners. It is fast, does not rely on distributional assumptions, and
does not face the burden of single-scenario forecasts. We show it is robust for
various demand and cost parameters, enhancing the efficiency and sustainability
of daily replenishment and routing decisions. Finally, Scenario
Predict-then-Optimize is general and can be easily extended to account for
other operational constraints, making it a useful tool in practice.
更多查看译文
AI 理解论文
溯源树
样例
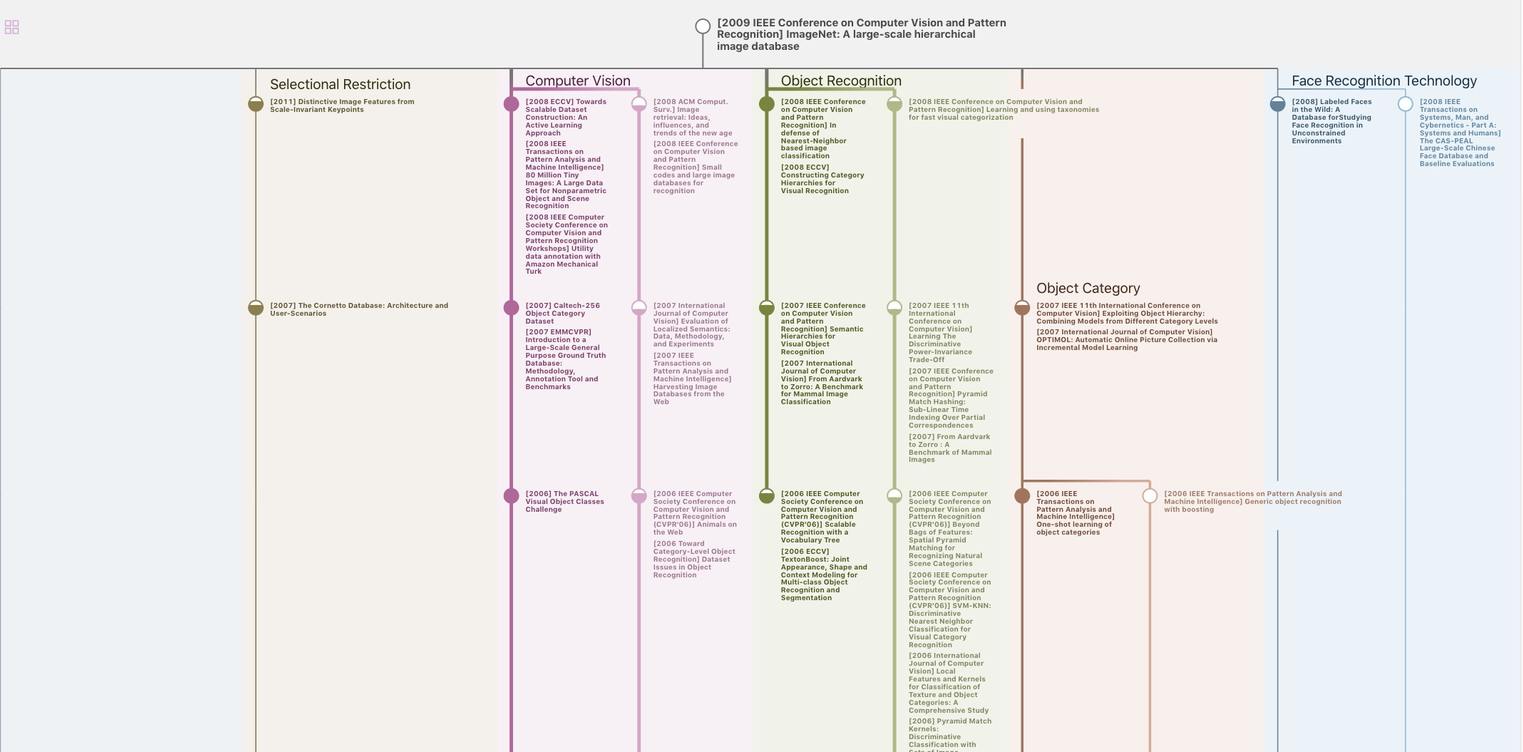
生成溯源树,研究论文发展脉络
Chat Paper
正在生成论文摘要