Characterizing substructure via mixture modeling of genetic similarity in large-scale summary statistics
biorxiv(2024)
摘要
Genetic summary data are broadly accessible and highly useful including for risk prediction, causal inference, fine mapping, and incorporation of external controls. However, collapsing individual-level data into groups masks intra- and inter-sample heterogeneity, leading to confounding, reduced power, and bias. Ultimately, unaccounted substructure limits summary data usability, especially for understudied or admixed populations. Here, we present Summix2, a comprehensive set of methods and software based on a computationally efficient mixture model to estimate and adjust for substructure in genetic summary data. In extensive simulations and application to public data, Summix2 characterizes finer-scale population structure, identifies ascertainment bias, and identifies potential regions of selection due to local substructure deviation. Summix2 increases the robust use of diverse publicly available summary data resulting in improved and more equitable research.
### Competing Interest Statement
The authors have declared no competing interest.
更多查看译文
AI 理解论文
溯源树
样例
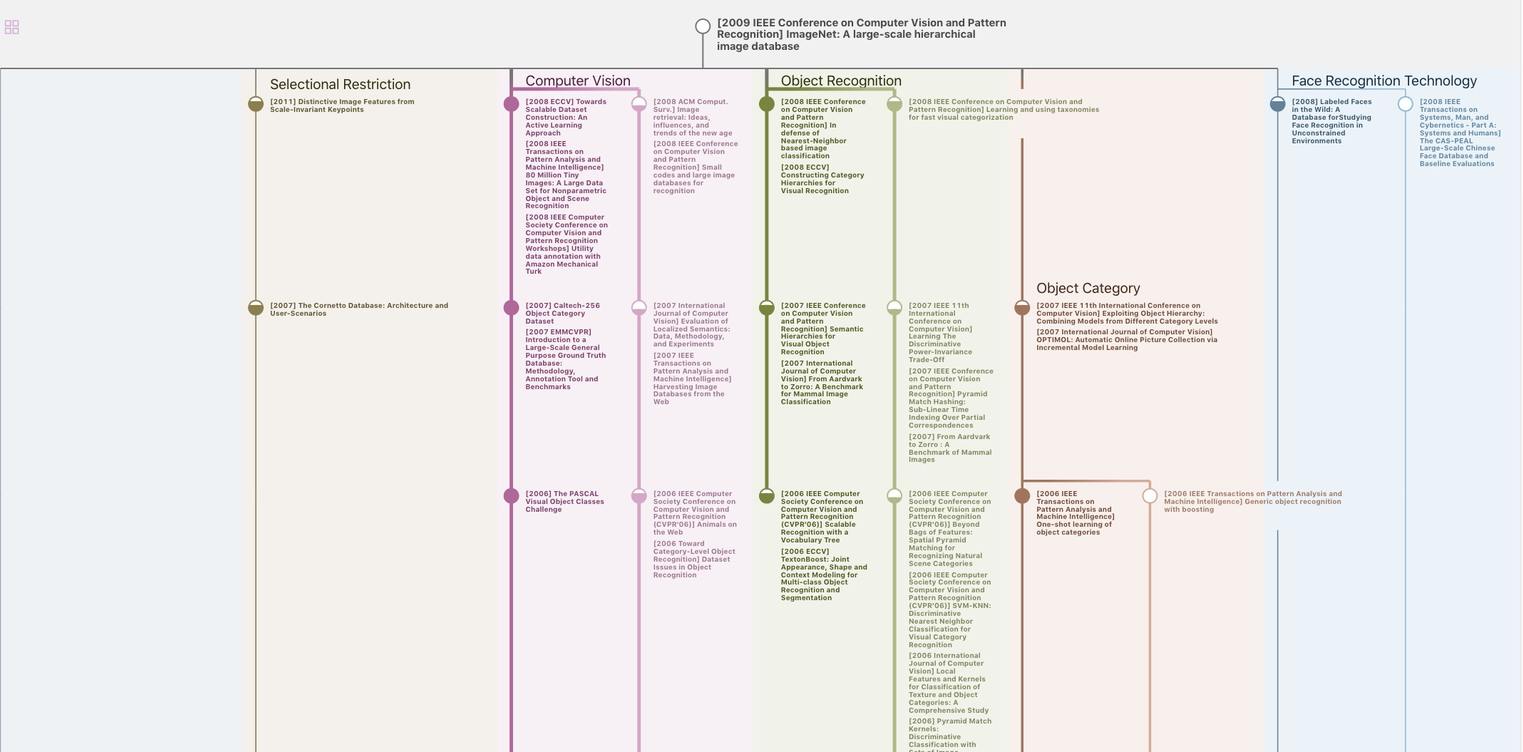
生成溯源树,研究论文发展脉络
Chat Paper
正在生成论文摘要