Clark-Park Transformation based Autoencoder for 3-Phase Electrical Signals.
IEEE PES Innovative Smart Grid Technologies Conference - Europe(2023)
摘要
During the past decades, significant progress has been made in the field of artificial neural networks to process images (Convolutional Neural Networks), audio signals (Temporal Convolutional Networks), or textual information (Transformers). However, for electrical three-phase signals processing, these network architectures ignore important characteristics and therefore lack of computational efficiency. This can lead to performance problems and limits the application potential of neural networks for a fast and efficient local analysis of three-phase electrical current or voltage waveforms. To address this issue, a novel autoencoder architecture is proposed in this paper, which incorporates Clark-Park transformation to learn the representations of three-phase electrical signals. Using unbalanced and noisy voltage signals, the Clark-Park based autoencoder shows superior performance and computational efficiency compared to recurrent and convolutional benchmark architectures.
更多查看译文
关键词
Autoencoder,IEC 61850 Sampled Values,Neural Networks,Space-Vector Transformation,Time Series Analysis
AI 理解论文
溯源树
样例
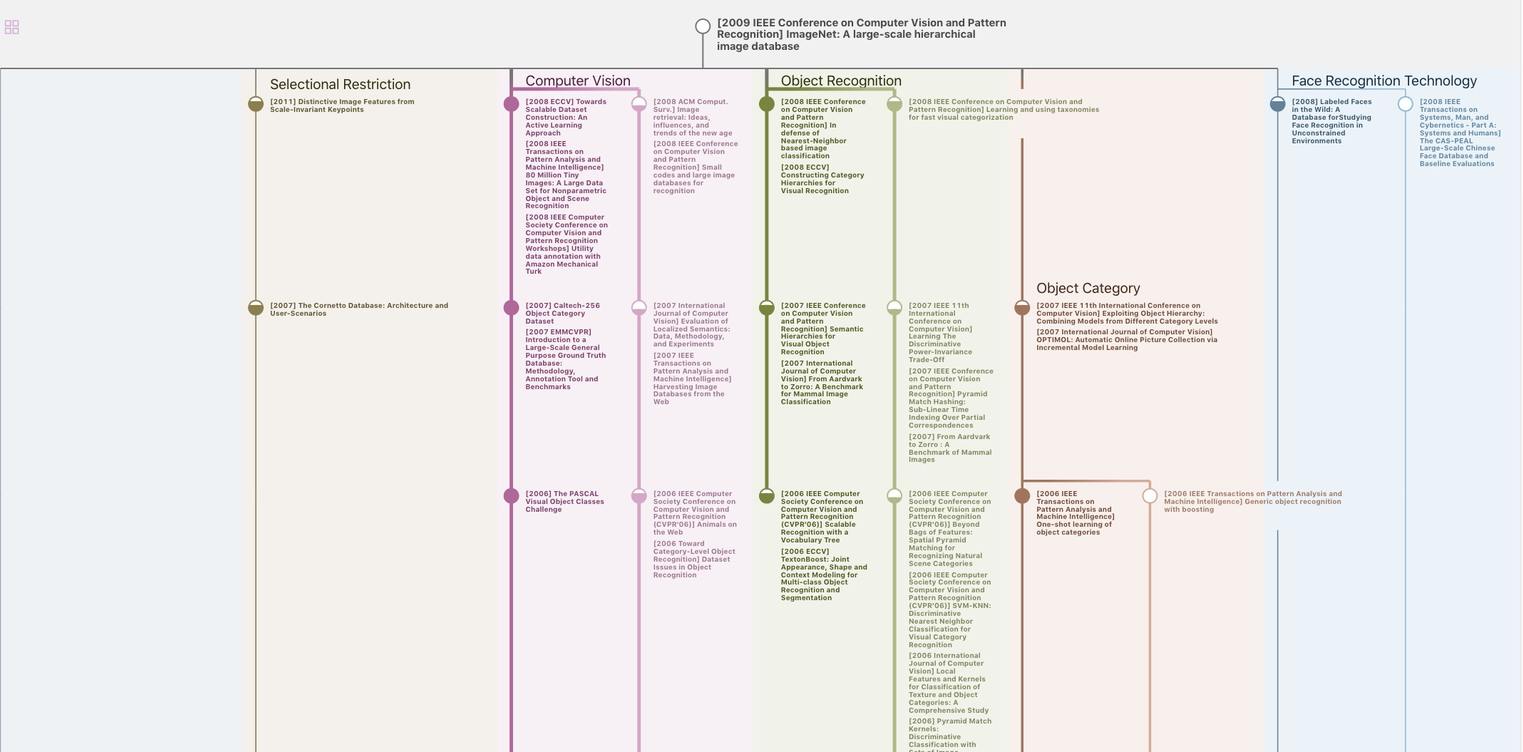
生成溯源树,研究论文发展脉络
Chat Paper
正在生成论文摘要