Quantifying near-fault impulsive effect on inelastic displacement ratios of self-centering SDOF systems using spectral-equivalent ground motions
STRUCTURES(2024)
摘要
Self-centering structures, which aim at minimizing post-earthquake residual deformations, have drawn increasing attention. Near-fault ground motions are known for their impulsive characteristics and may impose high seismic demands on self-centering structures compared to ordinary ground motions. This study investigates the near-fault impulsive effect on inelastic displacement ratios (CR, i.e., inelastic to elastic peak displacement ratio) of self-centering single-degree-of-freedom (SDOF) systems by developing the impulsive amplification ratio (Ap) spectra. First, the near-fault impulsive effect is quantified in isolation from the effect of elastic spectral shape by using spectral-equivalent pulse-like and ordinary ground motions. Constant-strength spectral analyses are conducted to develop the Ap spectra. Then, the probabilistic characteristics of Ap are analyzed, and systematic lognormality tests are conducted to evaluate the effectiveness of the lognormal distribution model for representing the probabilistic characteristics of Ap. The results show that the Ap spectra exhibit a complex multi-peak shape with strong dependence on the normalized period (T/Tp) and the hysteretic parameters of self-centering SDOF systems. Moreover, the lognormal distribution model can properly represent the probabilistic characteristics of the Ap index with superior robustness over variant combinations of the hysteretic parameters of selfcentering SDOF systems. Finally, the probabilistic prediction model for the Ap spectra is proposed using artificial neural network techniques, and the sufficiency of the model for assessing the near-fault impulsive effect under various probability levels is confirmed.
更多查看译文
关键词
Self-centering structures,Near-fault pulse-like ground motions,Response spectra,Single-degree-of-freedom systems,Probability distribution,Artificial neural networks
AI 理解论文
溯源树
样例
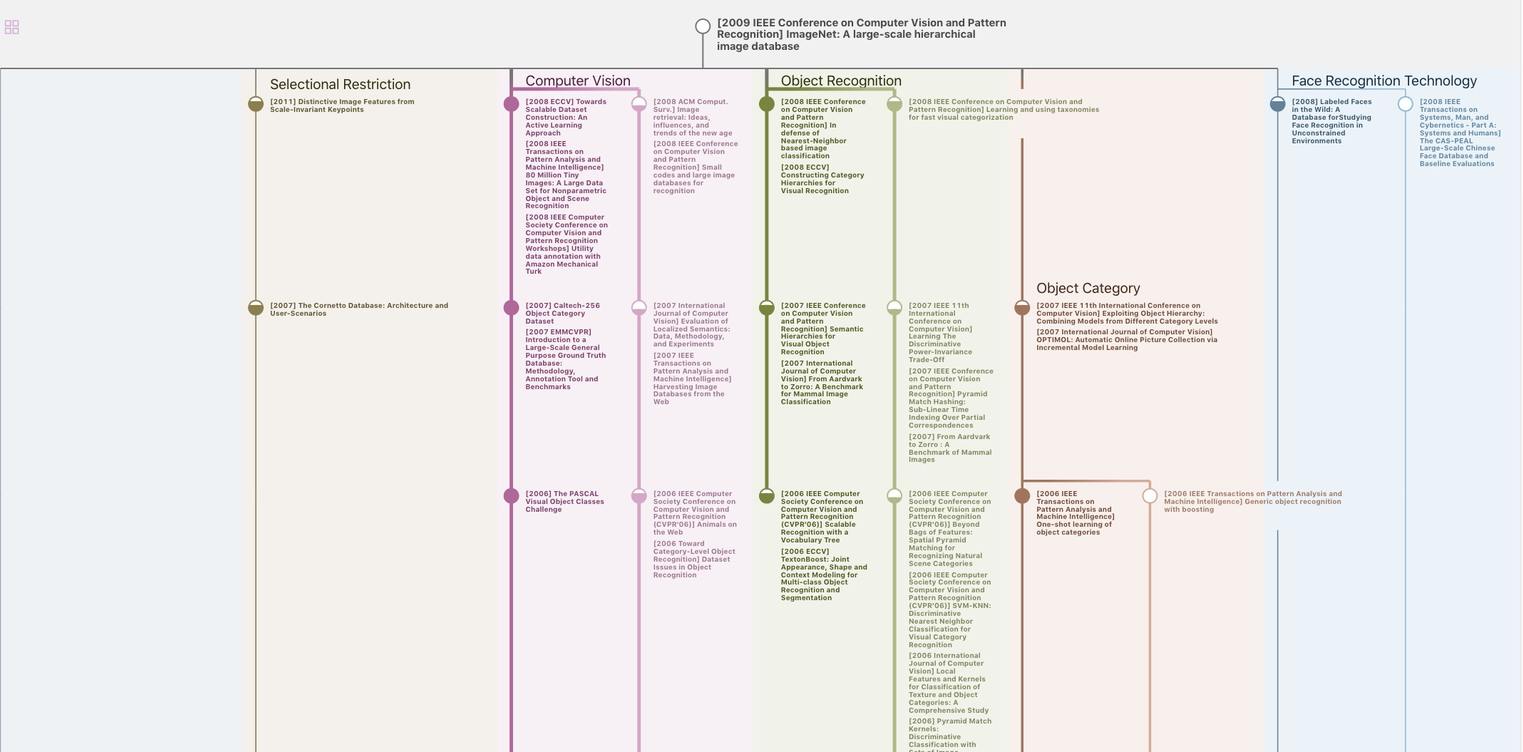
生成溯源树,研究论文发展脉络
Chat Paper
正在生成论文摘要