Text-Conditioned Image Synthesis - A Review
2023 IEEE Silchar Subsection Conference (SILCON)(2023)
Abstract
With the advent of Generative Adversarial Networks and diffusion models, image synthesis conditioned on text description has been an active area of research. Generative Adversarial Networks are a flexible and intuitive way of conditional image synthesis and significant progress has been made in the last few years regarding visual realism, diversity, and semantic alignment. In recent years, Diffusion probabilistic models have been shown to perform better in the task of image synthesis and have been used extensively for the task of text-to-image synthesis, significantly improving visual realism, semantic alignment, and generation of high-resolution images. However, the field still faces many challenges such as the generation of high-resolution images with multiple objects and the development of suitable and reliable evaluation metrics. In this review, we contextualize the state-of-the-art text-conditioned image generation models, and critically examine current evaluation strategies, architectures, model training, and datasets. This review complements previous surveys on text-conditioned image synthesis which we believe will help researchers to further advance this field.
MoreTranslated text
Key words
Generative Adversarial Networks,Diffusion Models,text-to-image synthesis
AI Read Science
Must-Reading Tree
Example
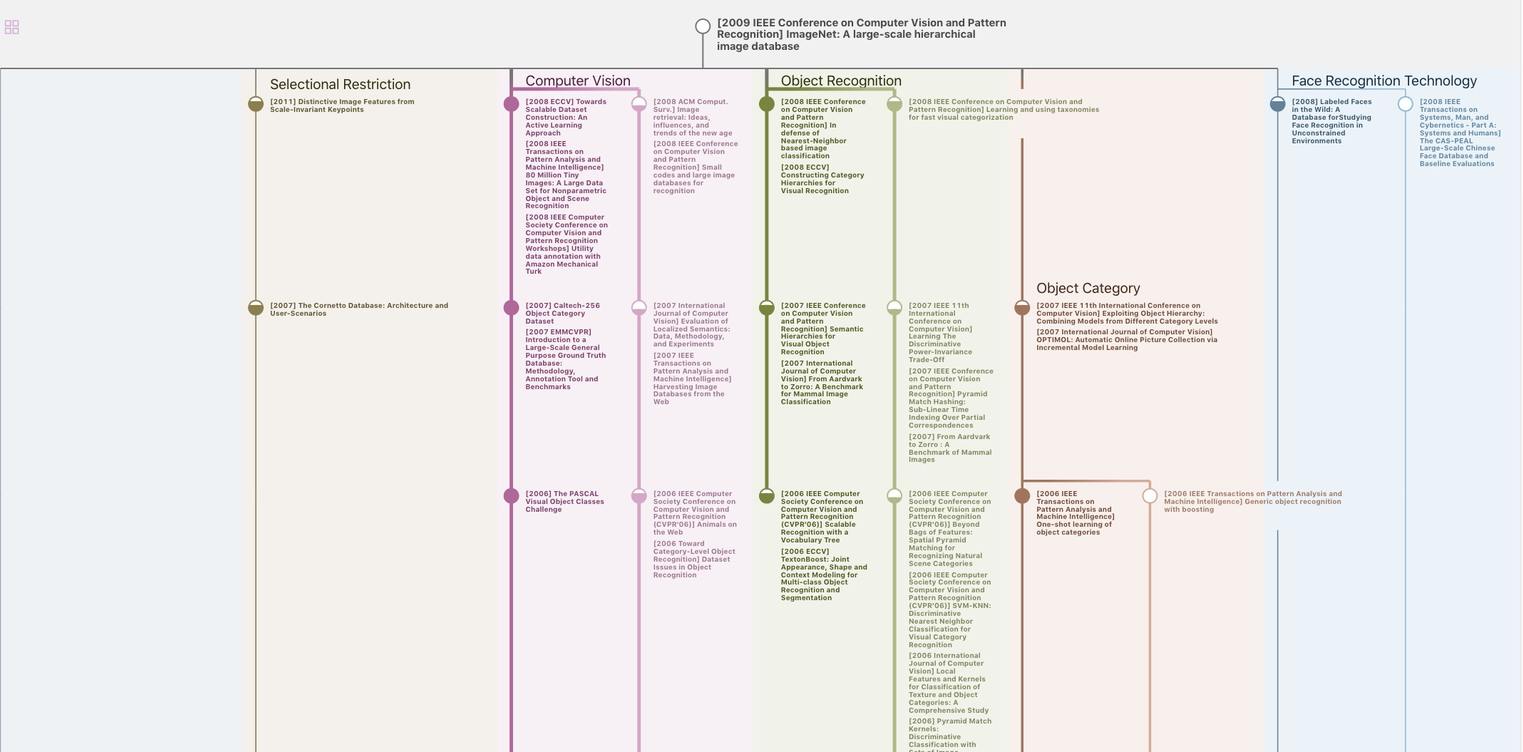
Generate MRT to find the research sequence of this paper
Chat Paper
Summary is being generated by the instructions you defined