CPR++: Object Localization via Single Coarse Point Supervision.
IEEE transactions on pattern analysis and machine intelligence(2024)
摘要
Point-based object localization (POL), which pursues high-performance object sensing under low-cost data annotation, has attracted increased attention. However, the point annotation mode inevitably introduces semantic variance due to the inconsistency of annotated points. Existing POL heavily rely on strict annotation rules, which are difficult to define and apply, to handle the problem. In this study, we propose coarse point refinement (CPR), which to our best knowledge is the first attempt to alleviate semantic variance from an algorithmic perspective. CPR reduces the semantic variance by selecting a semantic centre point in a neighbourhood region to replace the initial annotated point. We further integrate a variance regularization into the structure to concentrate the predicted scores, yielding CPR++. We observe that CPR++ can obtain scale information and further reduce the semantic variance in a global region, thus guaranteeing high-performance object localization. Extensive experiments on four challenging datasets validate the effectiveness of both CPR and CPR++. We hope our work can inspire more research on designing algorithms rather than annotation rules to address the semantic variance problem in POL. The dataset and code will be public at github.com/ucas-vg/PointTinyBenchmark.
更多查看译文
关键词
Object Localization,Semantic Variance,Point Annotation
AI 理解论文
溯源树
样例
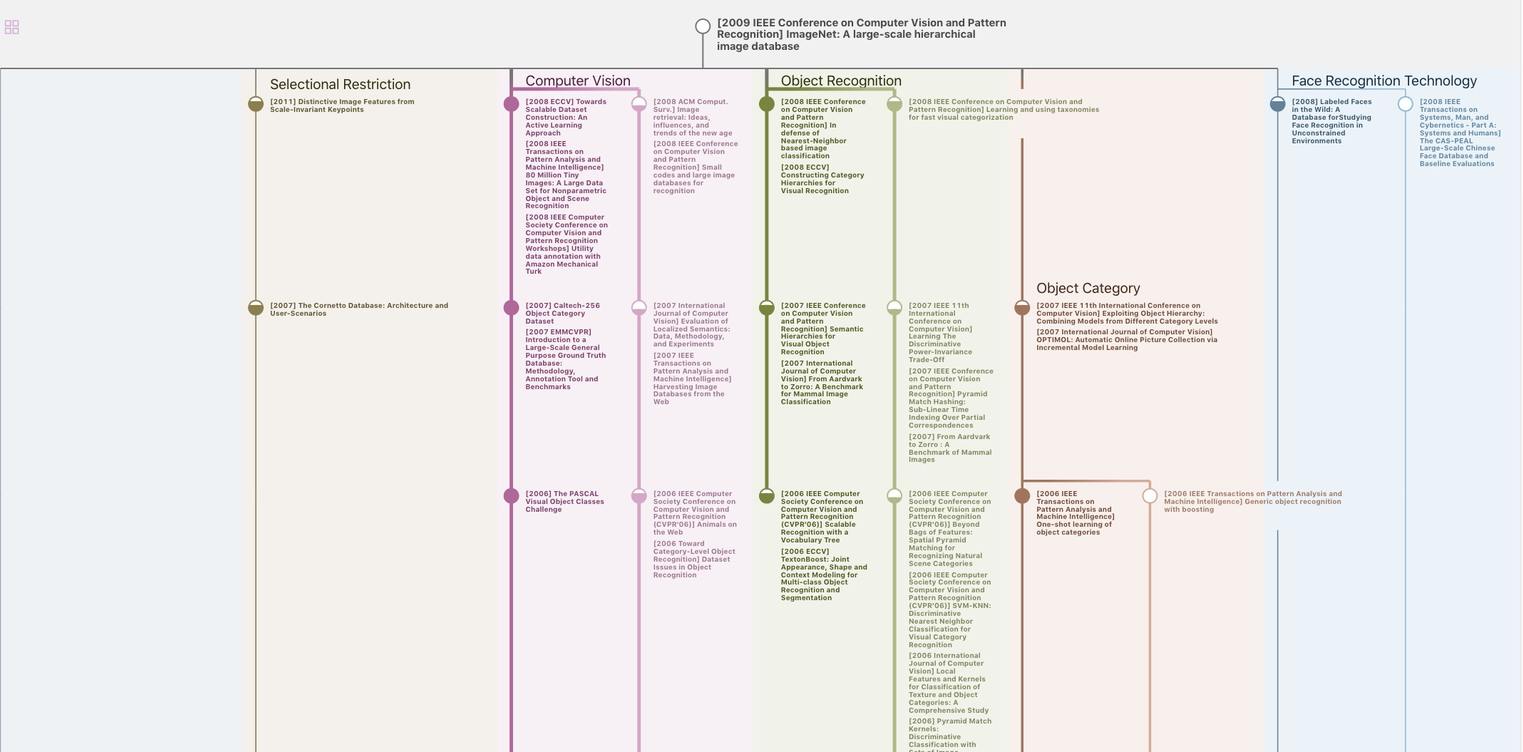
生成溯源树,研究论文发展脉络
Chat Paper
正在生成论文摘要