Leveraging Nested MLMC for Sequential Neural Posterior Estimation with Intractable Likelihoods
CoRR(2024)
Abstract
Sequential neural posterior estimation (SNPE) techniques have been recently
proposed for dealing with simulation-based models with intractable likelihoods.
They are devoted to learning the posterior from adaptively proposed simulations
using neural network-based conditional density estimators. As a SNPE technique,
the automatic posterior transformation (APT) method proposed by Greenberg et
al. (2019) performs notably and scales to high dimensional data. However, the
APT method bears the computation of an expectation of the logarithm of an
intractable normalizing constant, i.e., a nested expectation. Although atomic
APT was proposed to solve this by discretizing the normalizing constant, it
remains challenging to analyze the convergence of learning. In this paper, we
propose a nested APT method to estimate the involved nested expectation
instead. This facilitates establishing the convergence analysis. Since the
nested estimators for the loss function and its gradient are biased, we make
use of unbiased multi-level Monte Carlo (MLMC) estimators for debiasing. To
further reduce the excessive variance of the unbiased estimators, this paper
also develops some truncated MLMC estimators by taking account of the trade-off
between the bias and the average cost. Numerical experiments for approximating
complex posteriors with multimodal in moderate dimensions are provided.
MoreTranslated text
AI Read Science
Must-Reading Tree
Example
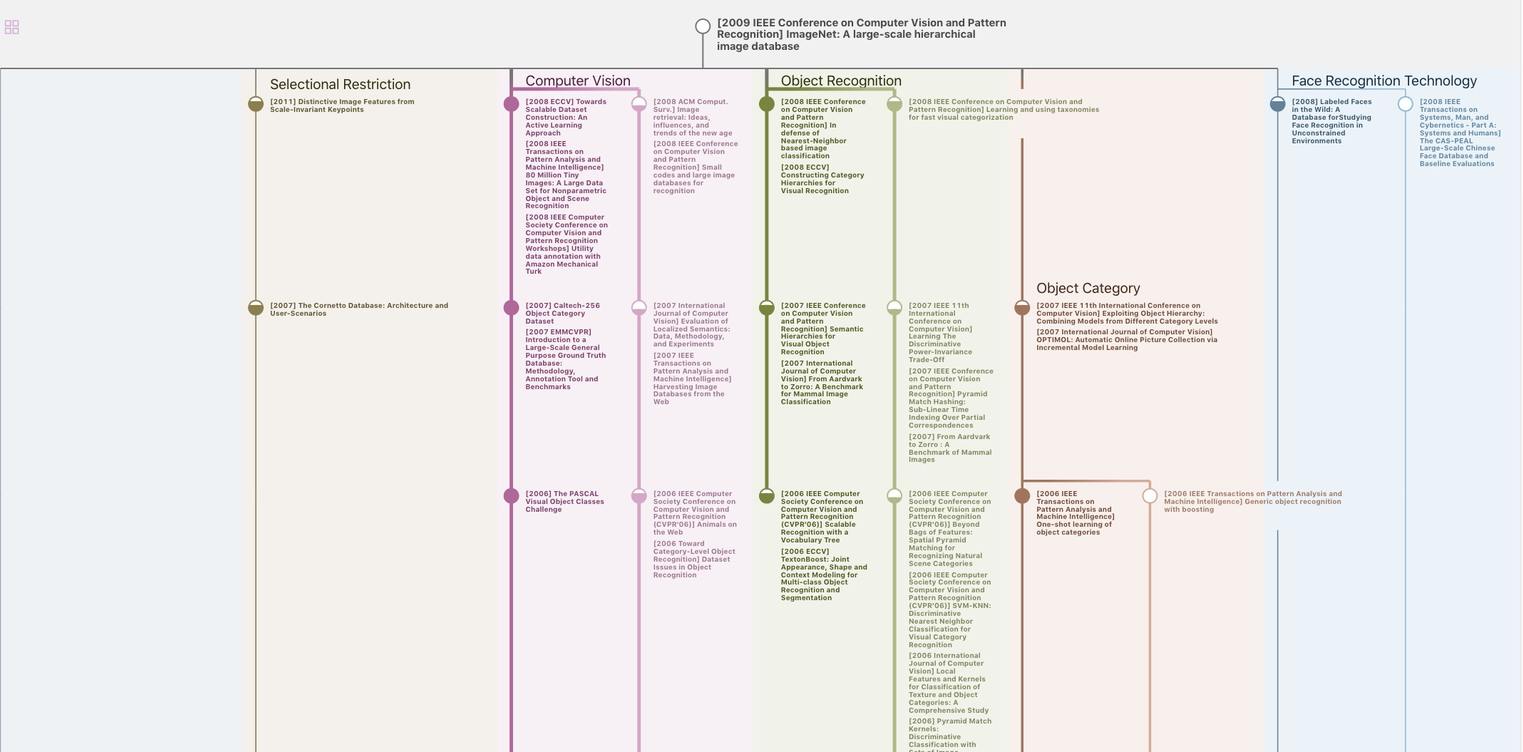
Generate MRT to find the research sequence of this paper
Chat Paper
Summary is being generated by the instructions you defined