Energy-conserving equivariant GNN for elasticity of lattice architected metamaterials
CoRR(2024)
摘要
Lattices are architected metamaterials whose properties strongly depend on
their geometrical design. The analogy between lattices and graphs enables the
use of graph neural networks (GNNs) as a faster surrogate model compared to
traditional methods such as finite element modelling. In this work we present a
higher-order GNN model trained to predict the fourth-order stiffness tensor of
periodic strut-based lattices. The key features of the model are (i) SE(3)
equivariance, and (ii) consistency with the thermodynamic law of conservation
of energy. We compare the model to non-equivariant models based on a number of
error metrics and demonstrate the benefits of the encoded equivariance and
energy conservation in terms of predictive performance and reduced training
requirements.
更多查看译文
关键词
mechanical metamaterials,lattices,elasticity,GNN,equivariant,positive definite,energy conservation
AI 理解论文
溯源树
样例
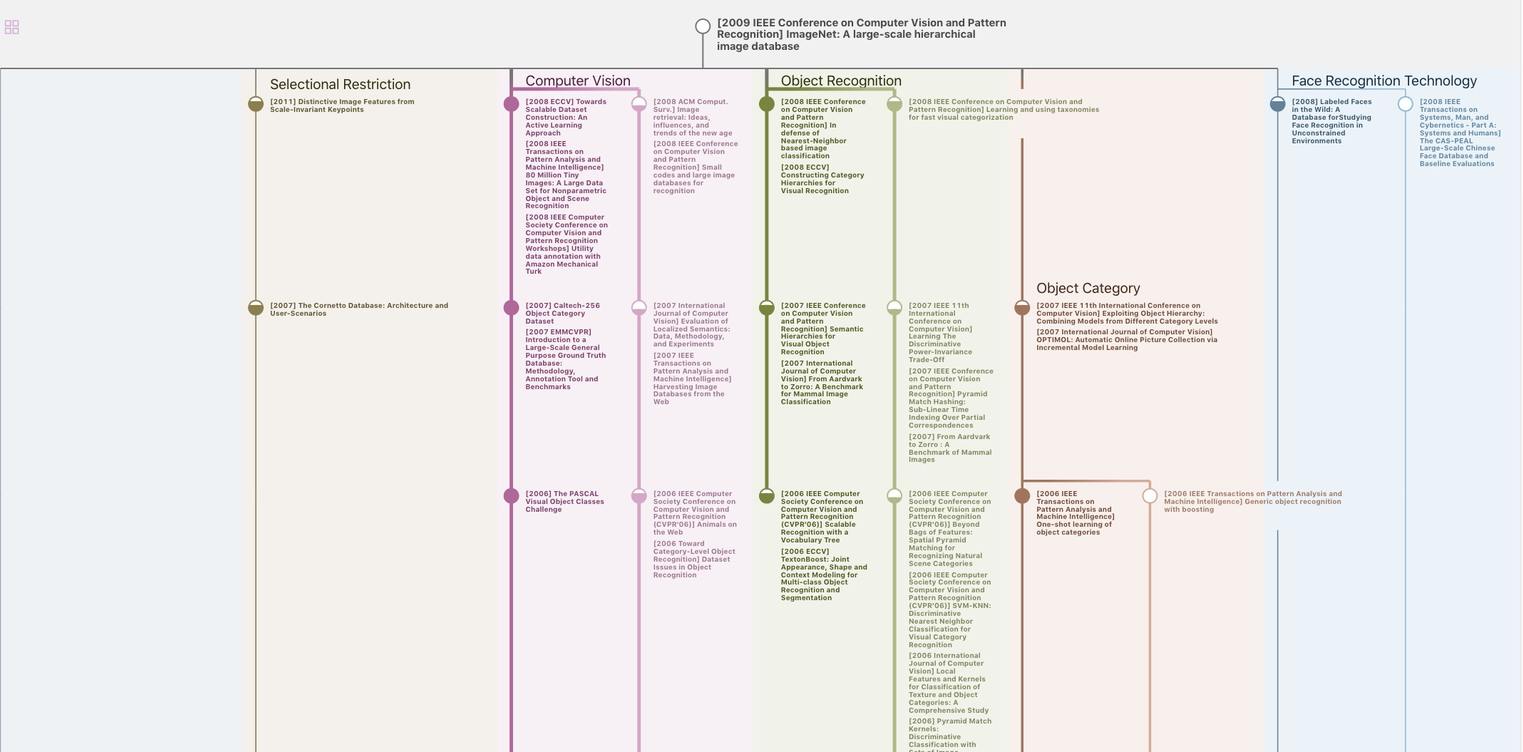
生成溯源树,研究论文发展脉络
Chat Paper
正在生成论文摘要