The Deep-Match Framework: R-Peak Detection in Ear-ECG.
IEEE transactions on bio-medical engineering(2024)
摘要
The Ear-ECG provides a continuous Lead I like electrocardiogram (ECG) by measuring the potential difference related to heart activity by electrodes which are embedded within earphones. However, the significant increase in wearability and comfort enabled by Ear-ECG is often accompanied by a degradation in signal quality - a common obstacle that is shared by the majority of wearable technologies. We aim to resolve this issue by introducing a Deep Matched Filter (Deep-MF) for the highly accurate detection of R-peaks in wearable ECG, thus enhancing the utility of Ear-ECG in real-world scenarios. The Deep-MF consists of an encoder stage (trained as part of an encoder-decoder module to reproduce ground truth ECG), and an R-peak classifier stage. Through its operation as a Matched Filter, the encoder section searches for matches with an ECG template pattern in the input signal, prior to filtering these matches with the subsequent convolutional layers and selecting peaks corresponding to the ground truth ECG. The so condensed latent representation of R-peak information is then fed into a simple R-peak classifier, of which the output provides precise R-peak locations. The proposed Deep Matched Filter is evaluated using leave-one-subject-out cross-validation over 36 subjects with an age range of 18-75, with the Deep-MF outperforming existing algorithms for R-peak detection in noisy ECG. The Deep-MF is benchmarked against a ground truth ECG, in the form of either chest-ECG or arm-ECG, via both R-peak recall and R-peak precision metrics. The Deep-MF achieves a median R-peak recall of 94.9% and a median precision of 91.2% across subjects when evaluated with leave-one-subject-out cross validation. Moreover, when evaluated across a range of thresholds, the Deep-MF achieves an area under the curve (AUC) value of 0.97. The interpretability of Deep-MF as a Matched Filter is further strengthened by the analysis of its response to partial initialisation with an ECG template. We demonstrate that the Deep Matched Filter algorithm not only retains the initialised ECG kernel structure during the training process, but also amplifies portions of the ECG which it deems most valuable - namely the P wave, and each aspect of the QRS complex. Overall, this Deep-Match framework serves as a valuable step forward for the real-world functionality of Ear-ECG and, through its interpretable operation, the acceptance of deep learning models in e-Health.
更多查看译文
AI 理解论文
溯源树
样例
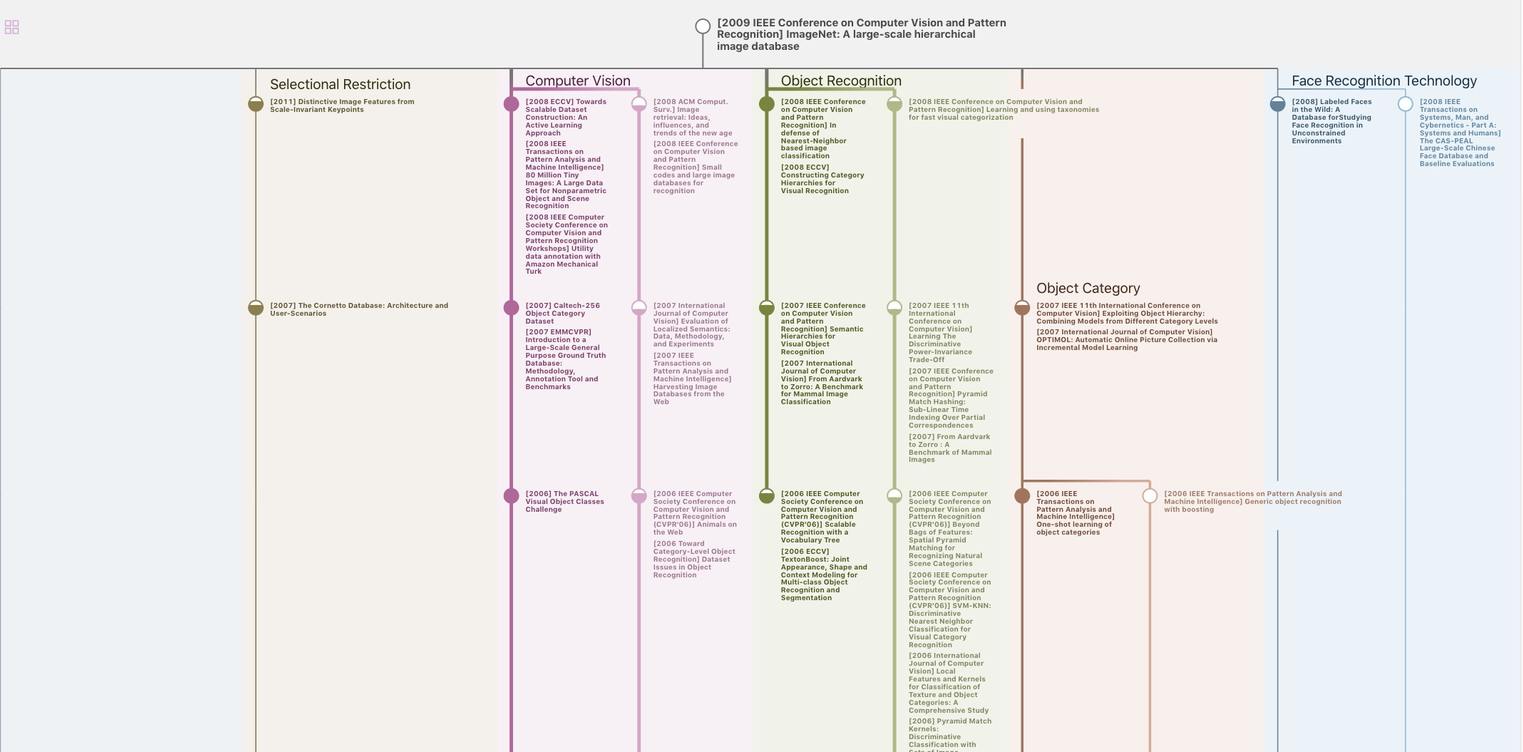
生成溯源树,研究论文发展脉络
Chat Paper
正在生成论文摘要