EPO-S: A Constrained RL Method to Enhance UAV Safety with Spatial Representation.
2023 IEEE International Conference on Systems, Man, and Cybernetics (SMC)(2023)
Abstract
Path planning and collision avoidance are critical components of UAV control algorithms that play a crucial role in executing UAV missions. As scenarios become increasingly complex, the traditional control methods just ain't cutting it to meet the requirements. Reinforcement learning is an emerging decision-making control algorithm that attempts to address these issues as an alternative to traditional methods and has made significant advances. Unfortunately, standard RL approaches only aim to maximize rewards, however balancing task performance and safety in completing UAV tasks poses a challenge since these two objectives sometimes conflict, leading to a trade-off often difficult to manage. This paper proposes three techniques to address this problem. First, we model the path planning and collision avoidance issue in a constrained RL framework, eliminating the need for complex reward engineering. Second, we expand our previous work in the UAV setting and introduce an exact penalty optimization (EPO) algorithm to provide stricter constraint guarantees. We also propose a novel spatial information representation method for the UAV scenario to help UAVs better understand environmental information. The experimental results demonstrate the effectiveness of the EPO and spatial representation modules proposed in this paper, through a significant reduction in collisions as well as a strong improvement in the rate of reaching the destination.
MoreTranslated text
Key words
UAV collision avoidance,spatial representation,constrained reinforcement learning,Exact Penalty Optimization
AI Read Science
Must-Reading Tree
Example
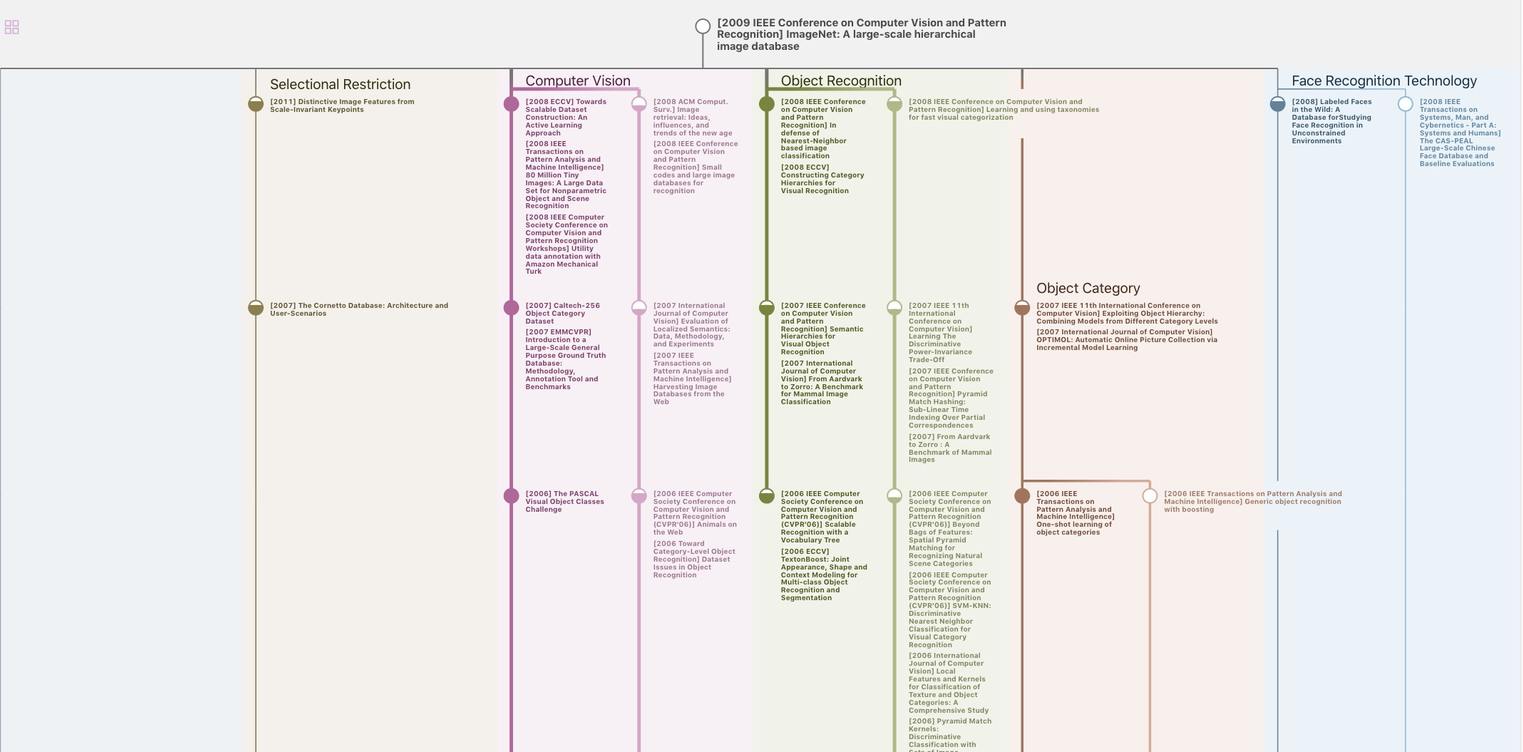
Generate MRT to find the research sequence of this paper
Chat Paper
Summary is being generated by the instructions you defined