Federated Learning with Common Representation Learning Criterion and Personalized Predictor.
2023 IEEE International Conference on Systems, Man, and Cybernetics (SMC)(2023)
摘要
Federated learning (FL) enables model training on decentralized devices while preserving data privacy. However, data heterogeneity poses a significant challenge to FL, and various approaches have been proposed to address it. Existing research has mainly focused on either enhancing global models or customizing personalized models for clients. This paper proposes a novel approach, FedCRC, that decouples the machine learning model into a representation extractor and predictor. This enables us to enhance both generalization and personalization, thereby addressing the challenge of data heterogeneity in FL. The approach employs a stable global predictor to unify the representation learning criterion during the training of the representation extractor. Additionally, a personalized predictor is trained for each client to achieve a personalized model tailored to the local data distribution. Our FedCRC algorithm was evaluated on multiple benchmark datasets with varying distributions, covering diverse settings. Extensive experimental results demonstrate the effectiveness of our method.
更多查看译文
关键词
Federated Learning,Data Heterogeneity,Representation Learning,Personalized Predictor
AI 理解论文
溯源树
样例
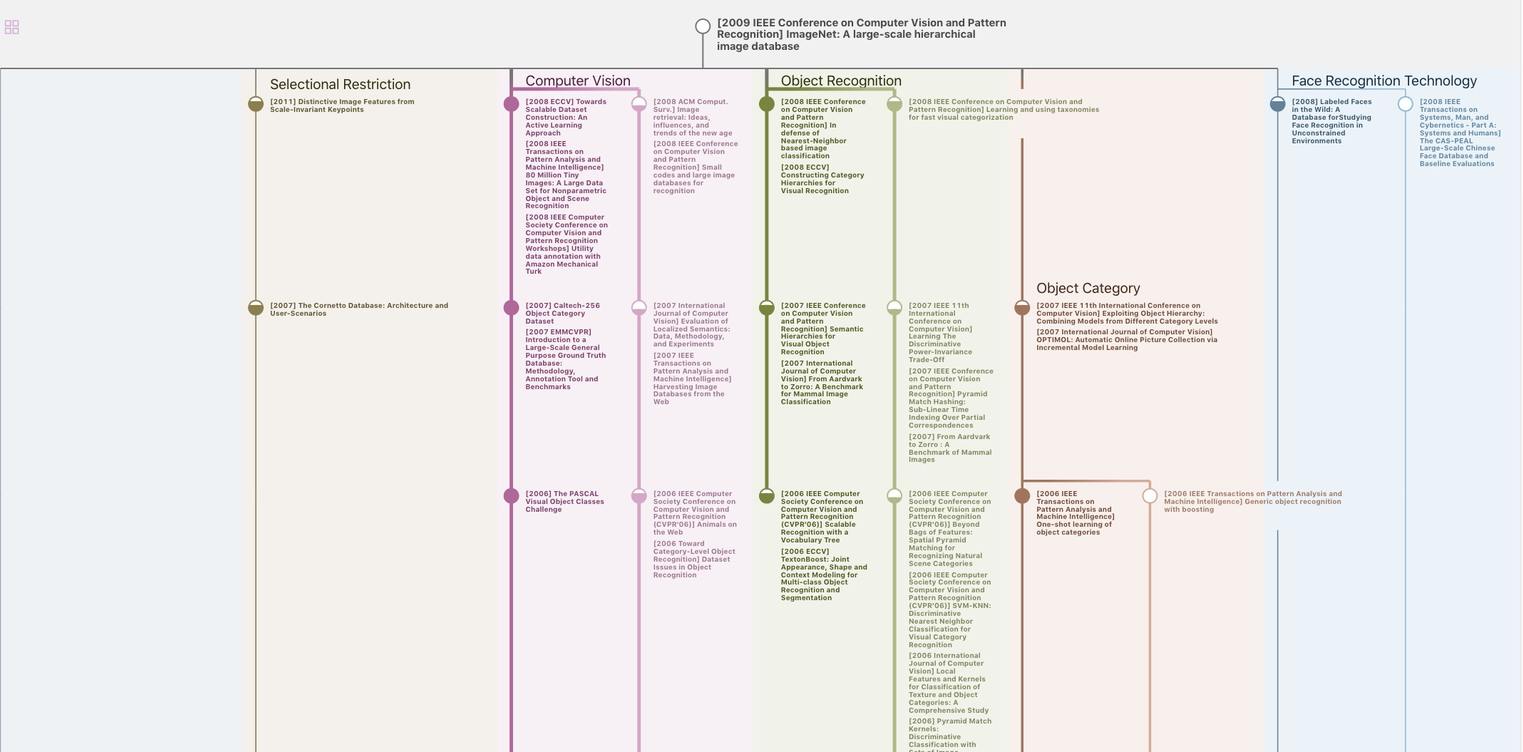
生成溯源树,研究论文发展脉络
Chat Paper
正在生成论文摘要