Preclinical Assessment of Upper Limb Tremor in Parkinson's Disease with Deep Learning and Wearable Technology.
2023 IEEE International Conference on Systems, Man, and Cybernetics (SMC)(2023)
摘要
Tremors are typically experienced by patients at the beginning of Parkinson's disease (PD). Clinicians evaluate clinical symptoms based on scale and experience, but mild tremors do not have significant characteristics and are difficult to observe with the naked eye. Implementing an intelligent and objective method to identify PD patients with early tremor symptoms and healthy controls (HC) is necessary. This study used wearable sensors to collect 9-axis inertial signals and 2-channel sEMG signals at the wrists of 13 HC and 24 PD patients from Dalian Municipal Central Hospital. Based on a Long Short-Term Memory Network (LSTM), an attention mechanism, and a Fully Convolutional Network (FCN), we develop a model to classify and recognize data from PD patients. Compared the proposed method with several classification methods, the results showed that the proposed method achieved higher classification accuracy (91.78%), precision (100%), recall (87.50%), and F1-score (93.33%) of PD class than Support Vector Machine, FCN, and LSTM. The computing time of the proposed method is approximately 1 second. The proposed method identifies early PD patients by pre-clinical assessment of mild upper limb tremors, which is valuable for early treatment and rehabilitation.
更多查看译文
关键词
Upper Limb,Wearable Devices,Upper Limb Tremor,Computation Time,Support Vector Machine,Long Short-term Memory,Attention Mechanism,Naked Eye,Parkinson’s Disease Patients,Wearable Sensors,Long Short-term Memory Network,Intelligence Methods,Fully Convolutional Network,sEMG Signals,Recall Of Class,Tremor Symptoms,Training Set,Clinical Diagnosis,Learning Rate,Convolutional Neural Network,Parkinson’s Disease Subjects,Inertial Measurement Unit,Working Methods,Parkinson’s Disease Symptoms,Acceleration Signal,Variance In The Dataset,Temporal Features,Inertial Data,Clinical Doctors,Traditional Machine Learning Methods
AI 理解论文
溯源树
样例
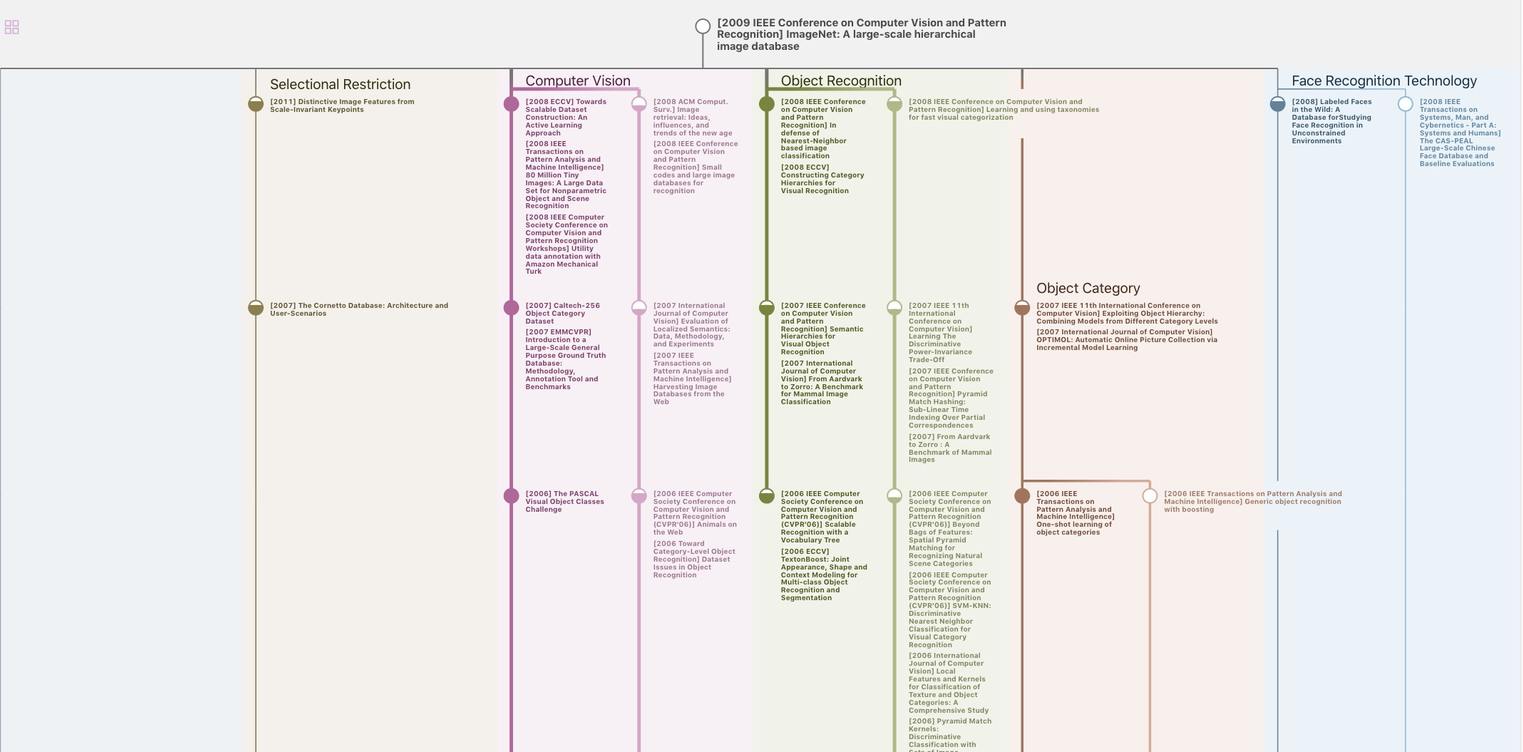
生成溯源树,研究论文发展脉络
Chat Paper
正在生成论文摘要