A Development of Time-Varying Weight Model Predictive Control for Autonomous Vehicles.
2023 IEEE International Conference on Systems, Man, and Cybernetics (SMC)(2023)
摘要
Autonomous vehicles, commonly known as self-driving cars, are rapidly gaining popularity due to their numerous advantages, such as reducing traffic, pollution, and emissions while increasing safety, convenience, and transportation connectivity. In order to accurately track the motion signal, these vehicles are now utilising advanced control techniques, such as model predictive control (MPC). However, the efficiency of MPCs heavily relies on properly tuning their weights. The primary function of the MPC is to recalculate the optimal values for the vehicle control commands, such as desired speed, steering angle, etc., while considering the dynamic model of the autonomous vehicle. The existing linear MPC models cannot reach higher efficiency because of using fixed weights without considering the error. This paper introduces a novel approach for developing an MPC model with a time-varying weights algorithm for autonomous vehicles. The study aims to minimise motion tracking errors such as lateral position and yaw angle errors. Relevant MPC weights are calculated online using fuzzy logic-based units considering the lateral position and yaw angle errors. The proposed linear time-varying MPC was designed and developed using MATLAB software, resulting in improved motion tracking performance with 31.62% and 20.89% reduction of the root means square error of lateral position and yaw angle.
更多查看译文
关键词
Autonomous Vehicles,Model Predictive Control,Time-varying Model,Control Of Autonomous Vehicles,Time-varying Weights,Time-varying Model Predictive Control,Linear Model,Root Mean Square Error,Dynamic Model,Lateral Position,Position Error,Tracking Error,Self-driving,Motion Tracking,Yaw Angle,Steering Angle,Vehicle Dynamics Model,Mean Square Error,Efficient System,Weight Matrix,Fuzzy Control,Input Rate,Fuzzy Logic,Fuzzy Rules,Membership Function,State-space Model,Yield Rate,Bicycle Model,Lateral Control,Advanced Tracking
AI 理解论文
溯源树
样例
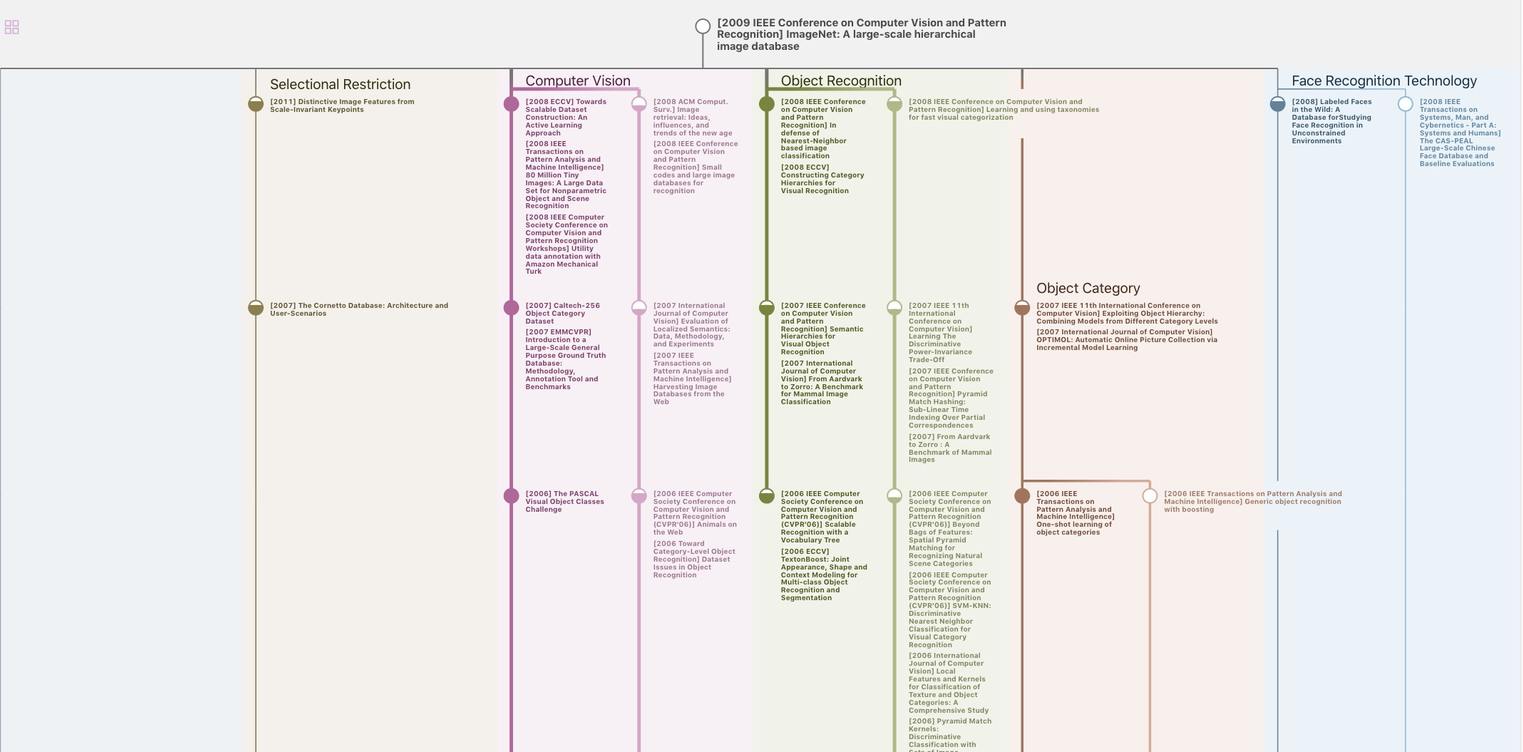
生成溯源树,研究论文发展脉络
Chat Paper
正在生成论文摘要