Decoding Neural Activity for Part-of-Speech Tagging (POS).
2023 IEEE International Conference on Systems, Man, and Cybernetics (SMC)(2023)
摘要
Decoding Part of Speech(POS) tagging directly from electroencephalography (EEG) signals whilst user overtly spoke (voiced speech) sentences could improve direct speech brain-computer interfaces (BCIs) using imagined or inner speech. To the best of our knowledge, earlier work uses machine learning approach using 74,953 sentences/tokens recorded in 75 EEG sessions. The tokens can be found in 4,479 phrases consisting of terms from the English Online treebank which contains the record of weblogs, newsgroups, reviews, and Yahoo Answers. The results demonstrated the feasibility of POS decoding from EEG based on word class, word frequency, and word length with accuracy of 71%, 86%, 89%, respectively. We believe that there is significant room for improvement with more advanced artificial intelligence. In this paper, we further extend the existing work with end-to-end transformers. Our results presents transformer model outperforms benchmark traditional ML results with +20% in length, +13% for the open vs closed class and +12% in frequency. In our empirical analysis, we find the decoding performance was better when using multi-electrode recordings as compared to single-electrode recordings.
更多查看译文
关键词
Part-of-speech,Postage,Word Frequency,Transformer Model,Word Length,Inner Speech,EEG Session,Training Set,Cognitive Processes,Classification Accuracy,Support Vector Machine,Hierarchical Structure,Language Processing,Vertical Line,Deep Learning Models,Long Short-term Memory,EEG Data,EEG Signals,Line Plots,Decoding Accuracy,Rapid Serial Visual Presentation,Gated Recurrent Unit,Neural Synchrony,EEG Dataset,Theta Band,Linear Support Vector Machine,Class Information,Language Structure,Independent Component Analysis
AI 理解论文
溯源树
样例
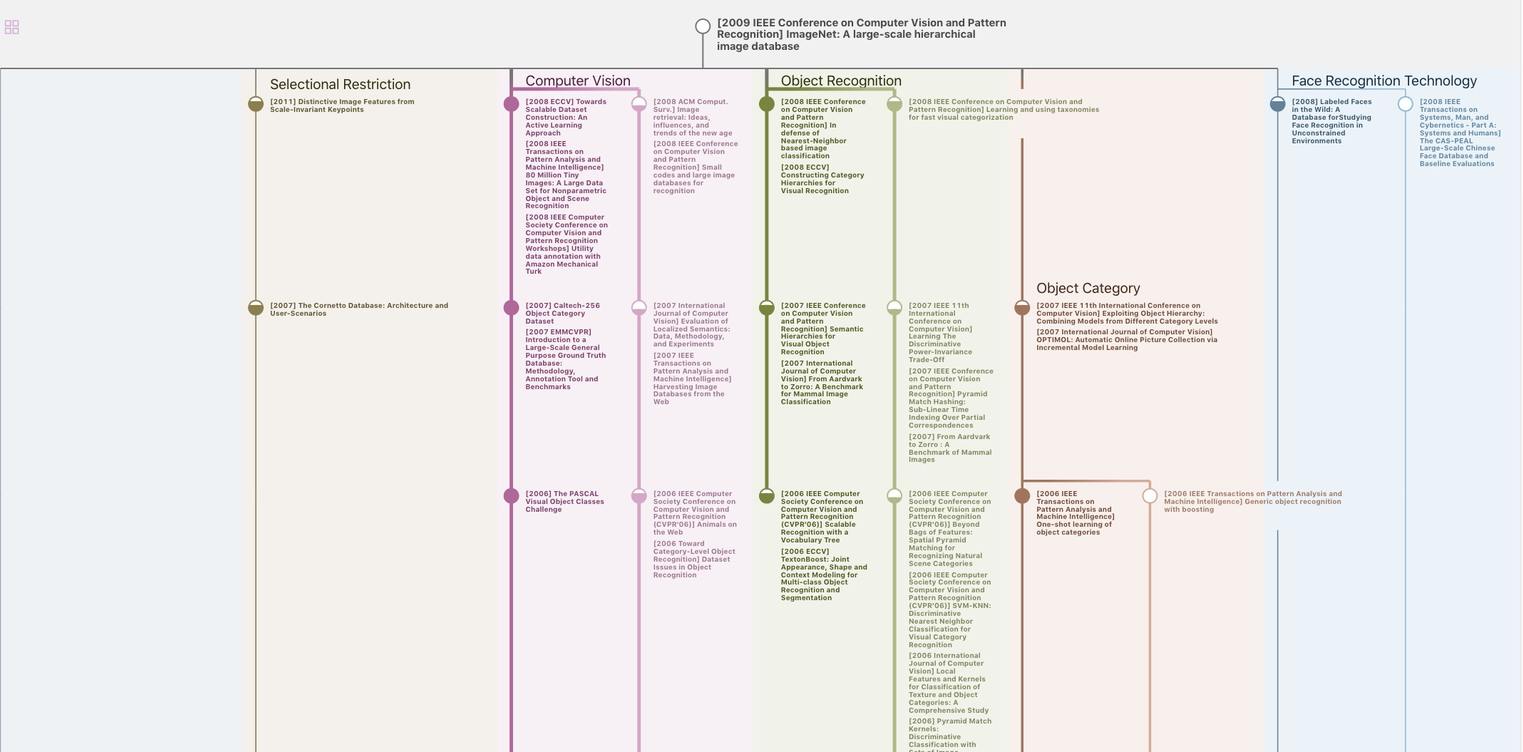
生成溯源树,研究论文发展脉络
Chat Paper
正在生成论文摘要