Are Deep Point Cloud Classifiers Suffer From Out-of-distribution Overconfidence Issue?
2023 IEEE International Conference on Systems, Man, and Cybernetics (SMC)(2023)
摘要
3D point cloud perception using deep neural networks (DNNs) has been a trend for various application scenarios. However, the black-box nature of DNNs will bring many hidden risks as in the 2D image field. In this paper, we present a preliminary evaluation on the out-of-distribution (OOD) overconfidence issue of deep point cloud classifiers, which has been proven to exist in deep 2D image classifiers, i.e., OOD inputs will lead to overconfident predictions on predefined categories. We also investigate whether a simple thresholding baseline and two modern OOD detection solutions can handle the issue by detecting OOD samples. Extensive experiments with four representative deep point cloud classifiers train/evaluate on different in/out-of-distribution point clouds validate the severity and knottiness of the OOD overconfidence issue. Our investigation will provide the groundwork for future studies on handling the OOD overconfidence issue of DNN classifiers for 3D point clouds.
更多查看译文
关键词
Point Cloud,Deep Clouds,Deep Point Cloud,Overconfidence Issue,Neural Network,Deep Neural Network,Field Images,2D Images,3D Point Cloud,Deep Neural Network Classifier,Simple Baseline,Deep Learning,Covariance Matrix,Positive Samples,False Positive Rate,Multilayer Perceptron,Distance Distribution,Error Detection,Mahalanobis Distance,Prediction Confidence,Point Cloud Classification,Point Cloud Dataset,Fréchet Inception Distance,Fast Gradient Sign Method,Input Point Cloud,Maximum Pooling,Complete Review
AI 理解论文
溯源树
样例
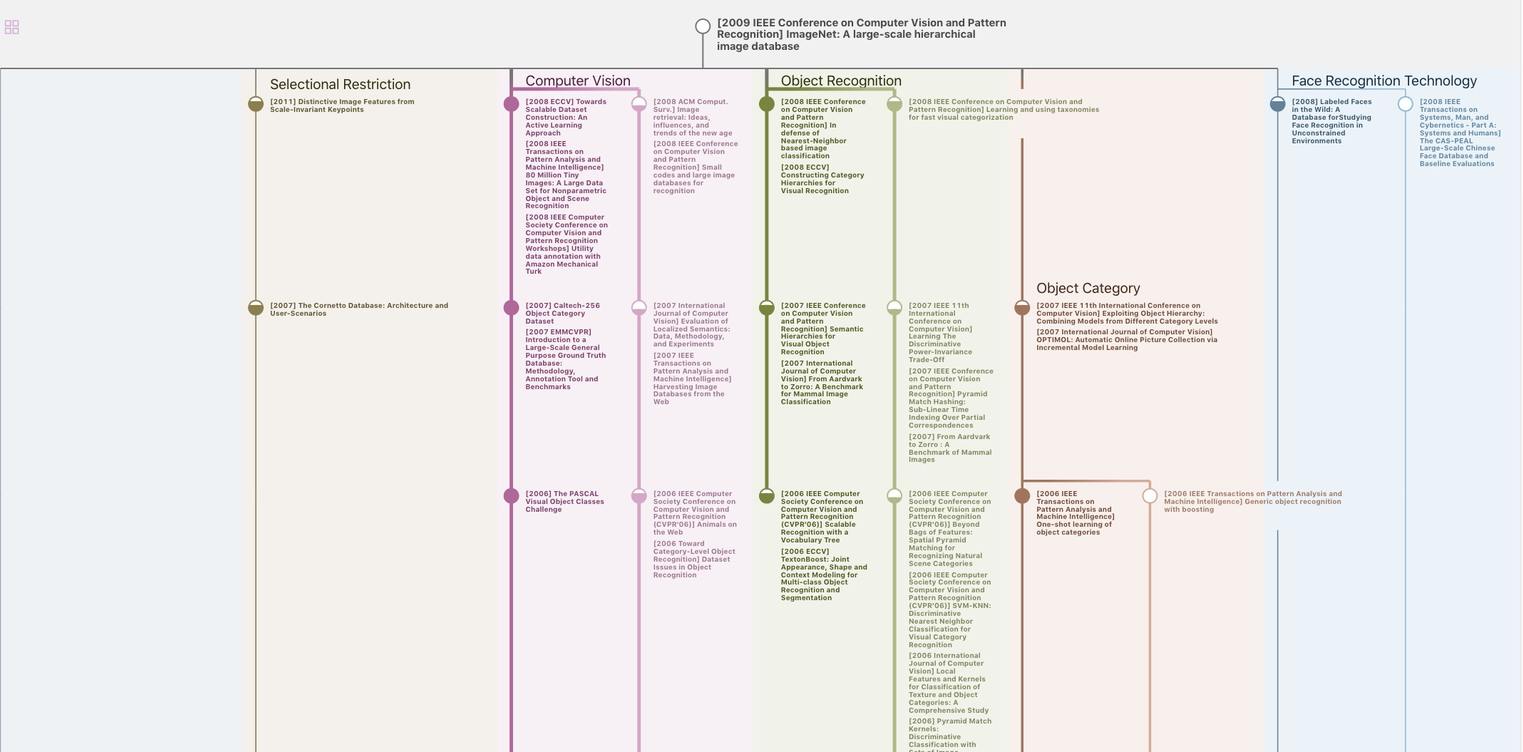
生成溯源树,研究论文发展脉络
Chat Paper
正在生成论文摘要