Sparse inference Type II fuzzy models optimized by Q-leaming algorithm
2023 38th Youth Academic Annual Conference of Chinese Association of Automation (YAC)(2023)
摘要
This paper presents a sparse inference method for improving the structure of Type II fuzzy systems, which can extract fuzzy rules from data structures without any assumptions and has advantages in solving modelling problems for such non-linear systems as industrial production. Typically, the fuzzy rule base extracted from the data is often incomplete, resulting in reduced stability of data-driven fuzzy systems. At the same time, we also propose a method to automatically adjust the fuzzy set based on Q-learning algorithm and a self-learning strategy to adjust the parameters of fuzzy rules, which can effectively avoid forgetting the old knowledge. Finally, we use the proposed method for fitting functions and predicting the residual resistance of sailing boats as well as compare the results with those obtained by other methods in the literature. The experimental results show that the data forecast using the Type II fuzzy system model are in satisfactory agreement with the actual data.
更多查看译文
关键词
Sparse inference method,Type II fuzzy systems,data-driven,Q-leaming,self-learning strategy
AI 理解论文
溯源树
样例
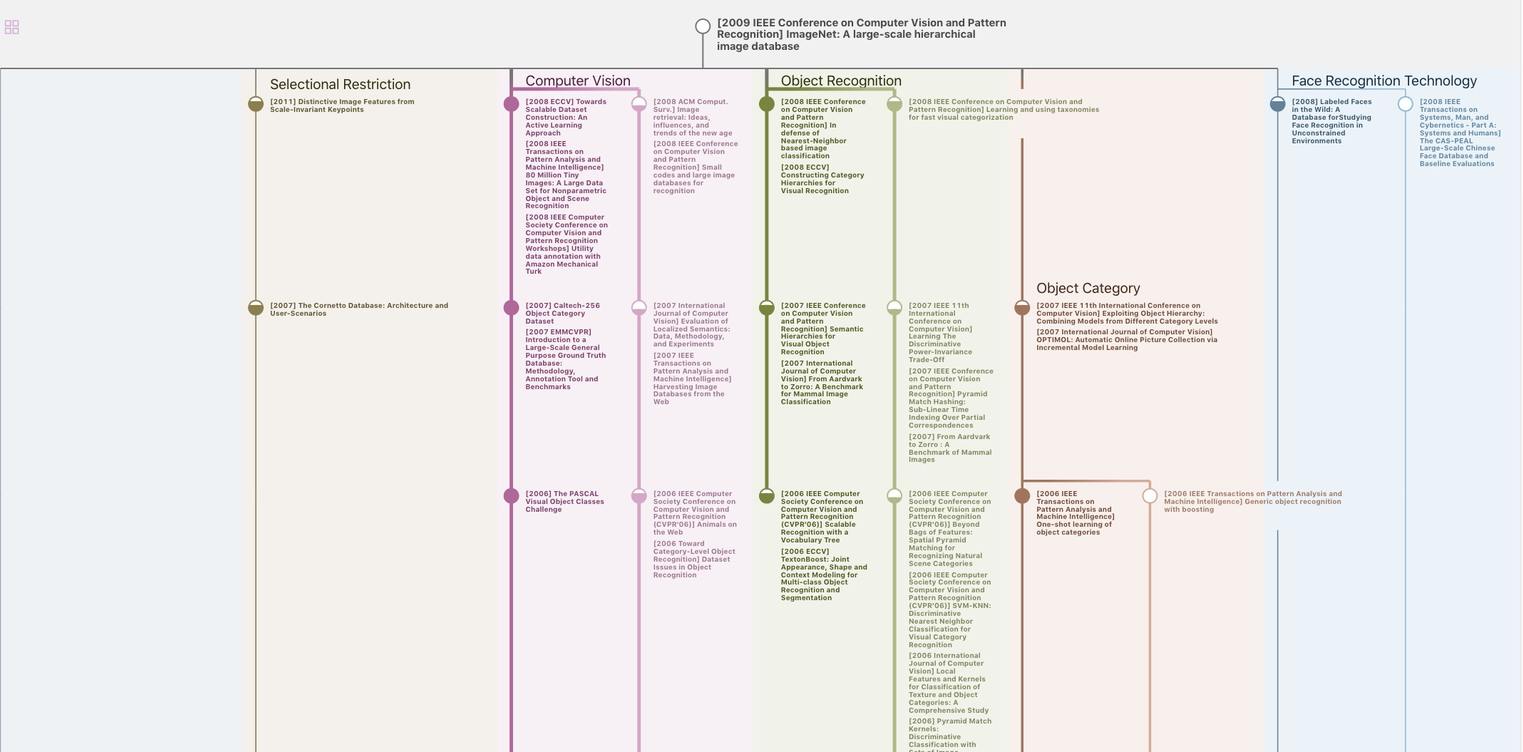
生成溯源树,研究论文发展脉络
Chat Paper
正在生成论文摘要