Group Lasso with Checkpoints Selection for Biological Data Regression.
2023 IEEE International Conference on Systems, Man, and Cybernetics (SMC)(2023)
摘要
Some unique characteristics of biological data are (1) that they are always High-Dimension and Low-Sample-Size (HDLSS) and (2) there are changes in the data distribution, such as an imbalance in classes, distribution and covariate shifts, etc. In this paper, we propose a Group Lasso with Checkpoints SElection (GL_CSE) algorithm to tackle both issues. To address the first issue, we utilize a group Lasso regression model tailored for HDLSS data to perform feature selection on predefined groups of features, alleviating overfitting and being invariant under group-wise orthogonal reparameterizations. To address the second issue, we propose the checkpoint selection method to extract important model checkpoints while training on group Lasso via two proposed metrics, i.e., the average KL-divergence between training and validation features and the Frobenius error of the covariance matrices between training and validation features. Both metrics aim to select model checkpoints with minimal drifts between the training and validation features. The results of our experiments indicate that our proposed GL_CSE algorithm achieves better performance compared to other baseline methods in terms of the MSE and R
2
measurements. Specifically, on the biological age dataset, our GL_CSE method achieves 0.8799 and 0.9883 for the MSE and R2 measurements, respectively. Additionally, we also show that our proposed checkpoint selection method performs better than regular K-fold cross-validation. Specifically, on the biological age dataset, GL_CSE (Q2) achieves 0.9045 MSE and 0.9880 R2, respectively, which outperforms the regular K-fold cross-validation results, i.e., 1.0612 MSE and 0.9871 R2, respectively.
更多查看译文
关键词
HDLSS,biological data,group Lasso regression,checkpoint
AI 理解论文
溯源树
样例
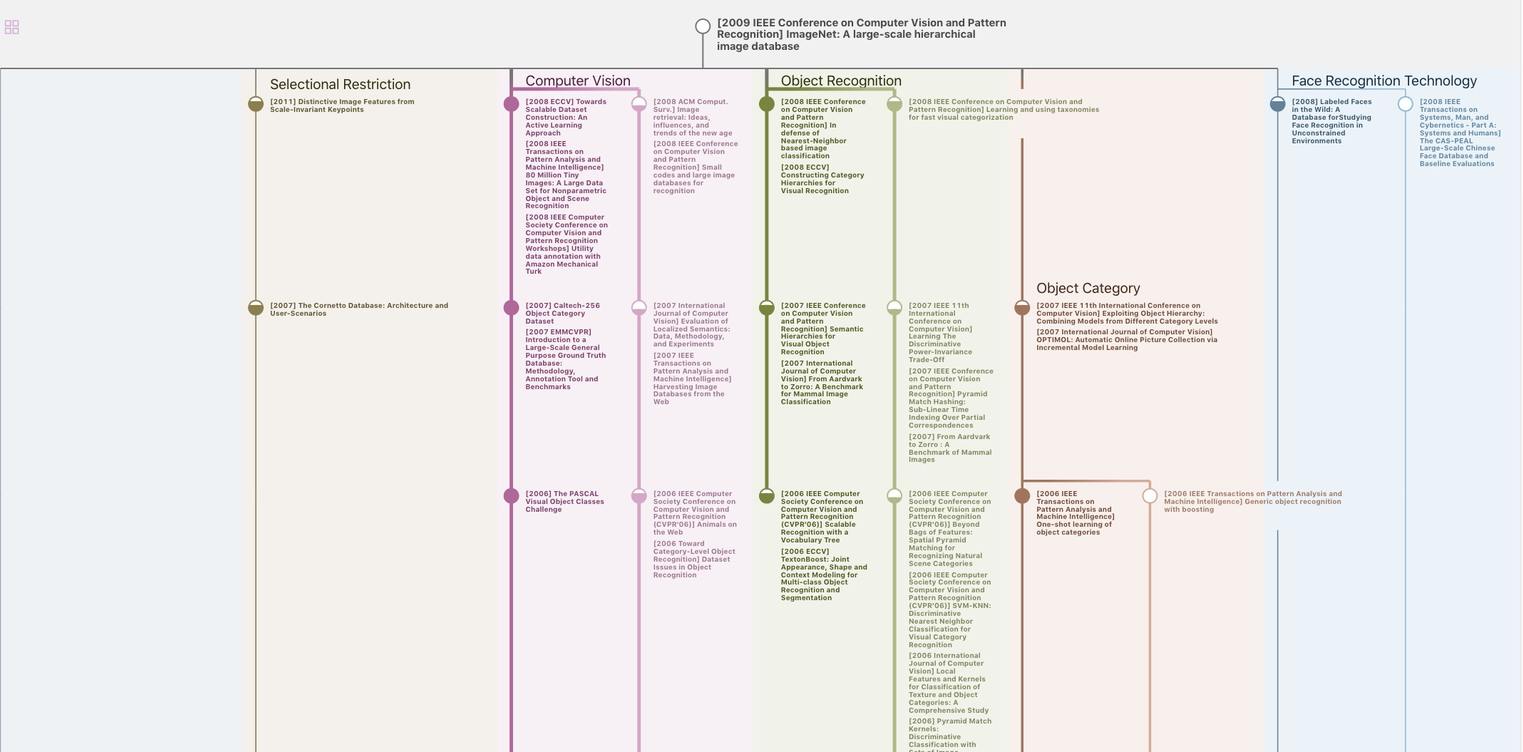
生成溯源树,研究论文发展脉络
Chat Paper
正在生成论文摘要