Choroidal Nevi Classification in Fundus Images Using a Patch-Based Deep Learning Approach
2023 IEEE EMBS Special Topic Conference on Data Science and Engineering in Healthcare, Medicine and Biology(2023)
摘要
Choroidal nevi are difficult to identify and require regular eye screening. While high-resolution fundus images are commonly used to identify choroidal nevi, manual review is time-consuming and requires specialized knowledge. Deep learning shows promise for accurate classification of eye diseases. However, these models require extensive labelled data, which is challenging for some medical conditions, like choroidal nevi. The objective of this study is to use a patch-based approach to classify fundus images as having choroidal nevus or not. We address data limitations, and the challenges posed by high-resolution images, through two key strategies. First, we uniformly extract tiled patches, expanding the training dataset, allowing a more detailed analysis of lesions, and preserving image quality by avoiding downsampling. However, this introduces a class imbalance issue which we effectively address through a second strategy involving different augmentations applied to the underrepresented class, mitigating the class imbalance issue, and further increasing the training set size. This approach achieved 92.61% accuracy, 90.47% recall, and 93.82% precision, outperforming the model trained on full-size images. We conclude that using a patch-based approach with noise and contrast enhancements outperforms conventional and simpler patch-based models.Clinical Relevance: This research tests a method for automating choroidal nevi identification to inform clinical diagnosis.
更多查看译文
关键词
Deep Learning,Fundus Images,Patch-based Approach,Choroidal Nevus,High-resolution Images,Contrast Agent,Full-size Images,Underrepresented Classes,Data Augmentation,ImageNet,Stochastic Gradient Descent,Training Experience,Dark Spots,Geometric Transformation,Eyelashes,Low Contrast Images
AI 理解论文
溯源树
样例
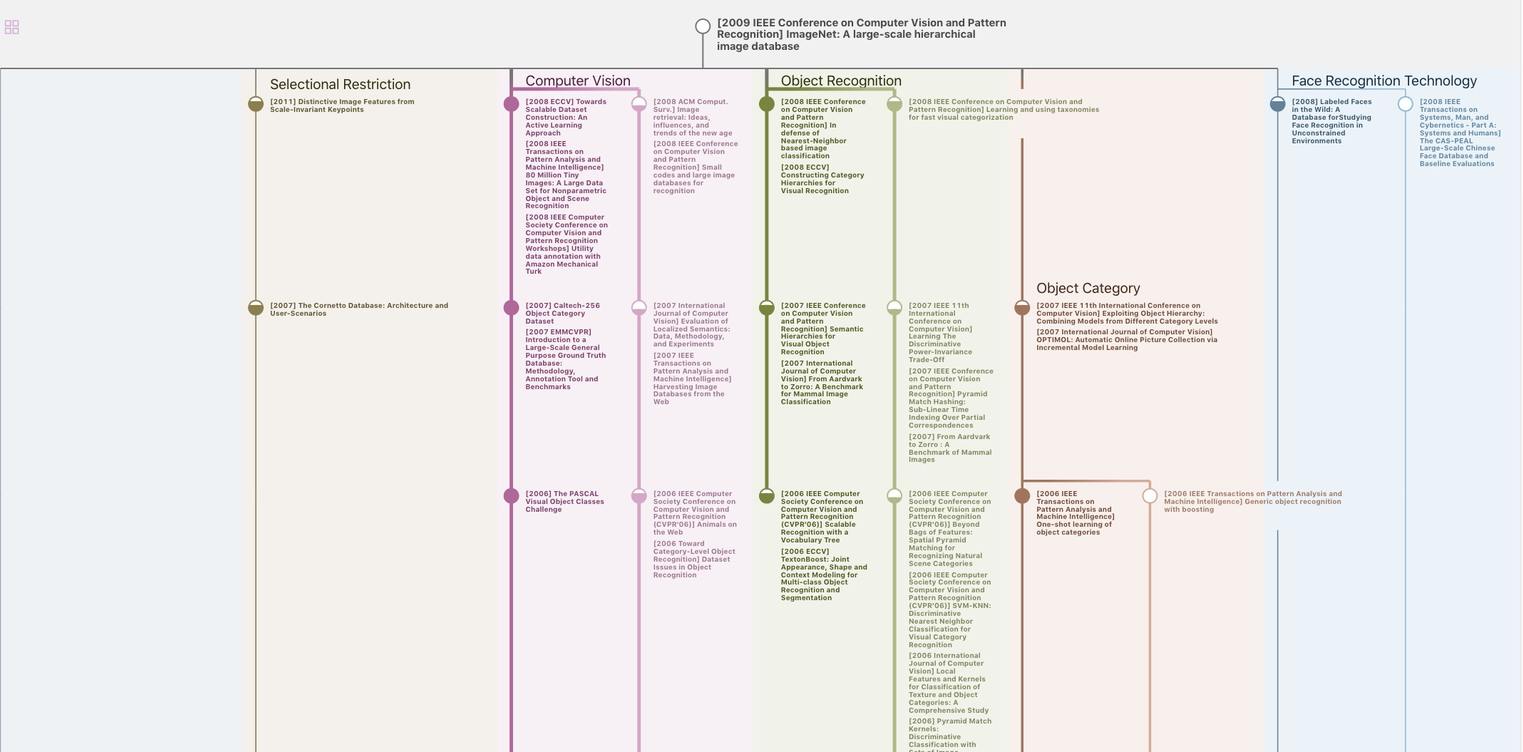
生成溯源树,研究论文发展脉络
Chat Paper
正在生成论文摘要