An Edge Deployment Framework to Scale AI in Industrial Applications
2023 IEEE 7TH INTERNATIONAL CONFERENCE ON FOG AND EDGE COMPUTING, ICFEC(2023)
摘要
Artificial Intelligence (AI) is increasingly explored in various domains and industries. Many companies experiment with AI, but too often those experiments are one-off analyses based on outdated data and the resulting models never make it into production. This paper proposes a framework for building and operating AI models at the industrial edge. The center of this framework is the model artifact, a model-generating entity. We analyze three AI model use-cases and user roles involved in industrial AI applications to illustrate the challenges in deploying and operating AI applications in industrial edge scenarios. We propose to structure the AI models into predefined artifacts that enable deployments with only a few clicks. The edge device links sensor data with the model input and returns the model output as feedback back into the industrial process. Model training, deployment, and management can be carried out in a scalable manner even by a non-expert. Several models can be managed in parallel, and data can be linked to the respective sensor or machine.
更多查看译文
关键词
Edge Computing,Artificial Intelligence,IoT
AI 理解论文
溯源树
样例
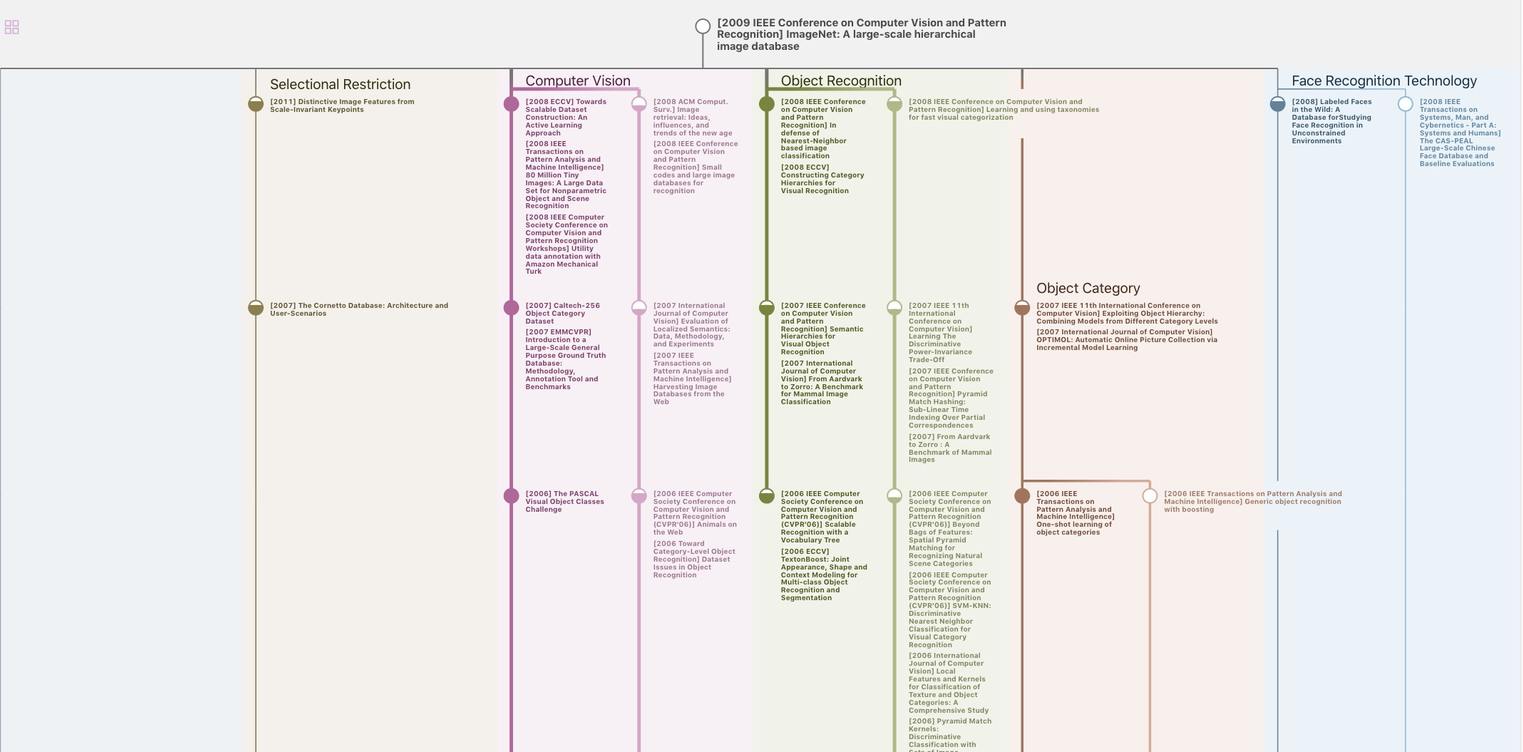
生成溯源树,研究论文发展脉络
Chat Paper
正在生成论文摘要