SupCon-ViT: Supervised contrastive learning for ultra-fine-grained visual categorization.
2023 International Conference on Digital Image Computing: Techniques and Applications (DICTA)(2023)
摘要
With the increasing availability of datasets exhibiting fine granularity and subtle differences between categories, fine-grained visual categorization tasks have gained significant attention across various domains. However, the focus often lies solely on overall dataset performance metrics such as top-l accuracy, while lacking a comprehensive understanding of the underlying factors. This paper addresses this gap by presenting a detailed analysis of the CUB-200-2011 dataset through extensive experiments. We identify and investigate specific ultra-fine-grained subsets that significantly impact the overall accuracy of the dataset. To enhance the performance of ultra-fine-grained visual classification, we propose SupCon-ViT, an ultra-fine-grained visual categorization network based on supervised contrastive learning. The key component of our approach is a supervised contrastive learning module, which effectively guides the network to learn discriminative local features within samples. This is accomplished by continuously pulling closer the normalized embeddings from the same class and pushing away embeddings from different classes. As a result, our approach achieves discriminative local representations, leading to improved network classification performance. Experimental results demonstrate the effectiveness of our proposed method on four ultra-fine-grained subsets of the CUB dataset. Notably, our approach achieves significant performance improvements without requiring additional expert information during training. This work contributes to the broader understanding of fine-grained visual categorization and offers a practical solution to enhance the accuracy of ultrafine-grained visual classification tasks.The code is available at https://github.comnucinda01ove/SupCon-ViT-pytorch.
更多查看译文
关键词
Ultra-fine-grained visual categorization,Supervised contrastive learning,Transformer
AI 理解论文
溯源树
样例
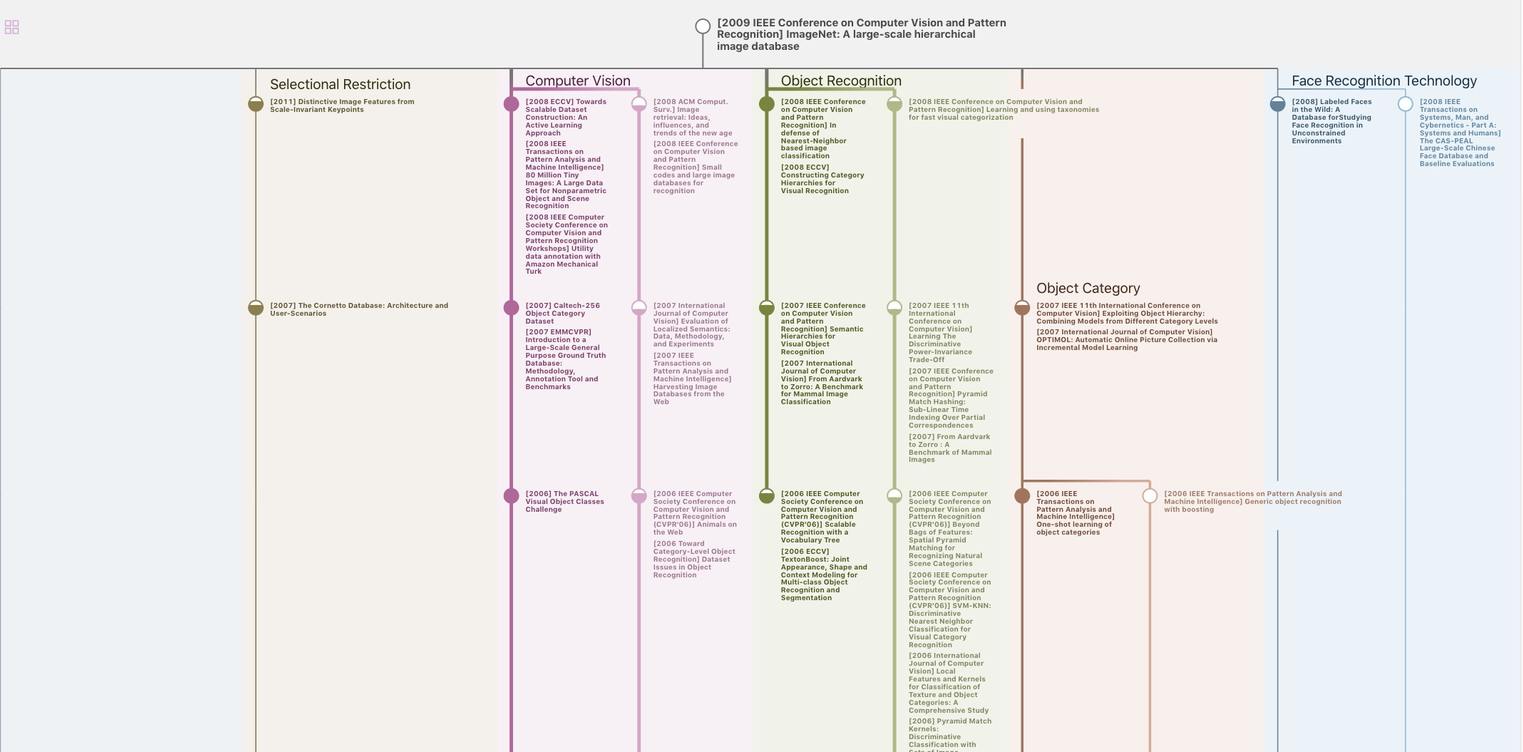
生成溯源树,研究论文发展脉络
Chat Paper
正在生成论文摘要