Self-supervised Defocus Map Estimation and Auxiliary Image Deblurring Given a Single Defocused Image.
2023 International Conference on Digital Image Computing: Techniques and Applications (DICTA)(2023)
Abstract
In this paper, we propose an end-to-end self-supervised Deep Neural Network (DNN) for Defocus Map Estimation (DME). Currently, such defocus maps are estimated by DNNs with fully supervised learning. For training, such networks need large datasets annotated by defocus amount or scene depth, which are challenging to obtain. The networks with self-supervised training arrive to overcome the limitation represented by the Ground Truth (GT) data. In this line, we propose a self-supervised learning neural network for DME from a single defocused image. Our method is based on a recently proposed DNN called 2HDED:NET that we enriched with a defocus simulation module that makes possible the self-supervised training for DME. In addition to the defocus map, our network reconstructs the All-in-Focus (AIF) image through supervised learning. We test the network on synthetic and realistic benchmarks and demonstrate that it is an effective solution for DME and image deblurring when a single defocused image is available.
MoreTranslated text
Key words
Posterior Mode,Image Deblurring,Defocused Images,Defocus Map,Defocus Map Estimation,Neural Network,Deep Neural Network,Self-supervised Learning,Self-supervised Training,Loss Function,Training Set,Convolutional Neural Network,Learning Network,Stochastic Gradient Descent,Generative Adversarial Networks,Image Sensor,Depth Map,Peak Signal-to-noise Ratio,Point Spread Function,Deep Neural Network Model,Region-based Methods,Depth Estimation,Camera Array,Multi-task Learning,Auxiliary Task,Thin Lens,Structural Similarity Index,Realistic Dataset,L1-norm,Training Dataset
AI Read Science
Must-Reading Tree
Example
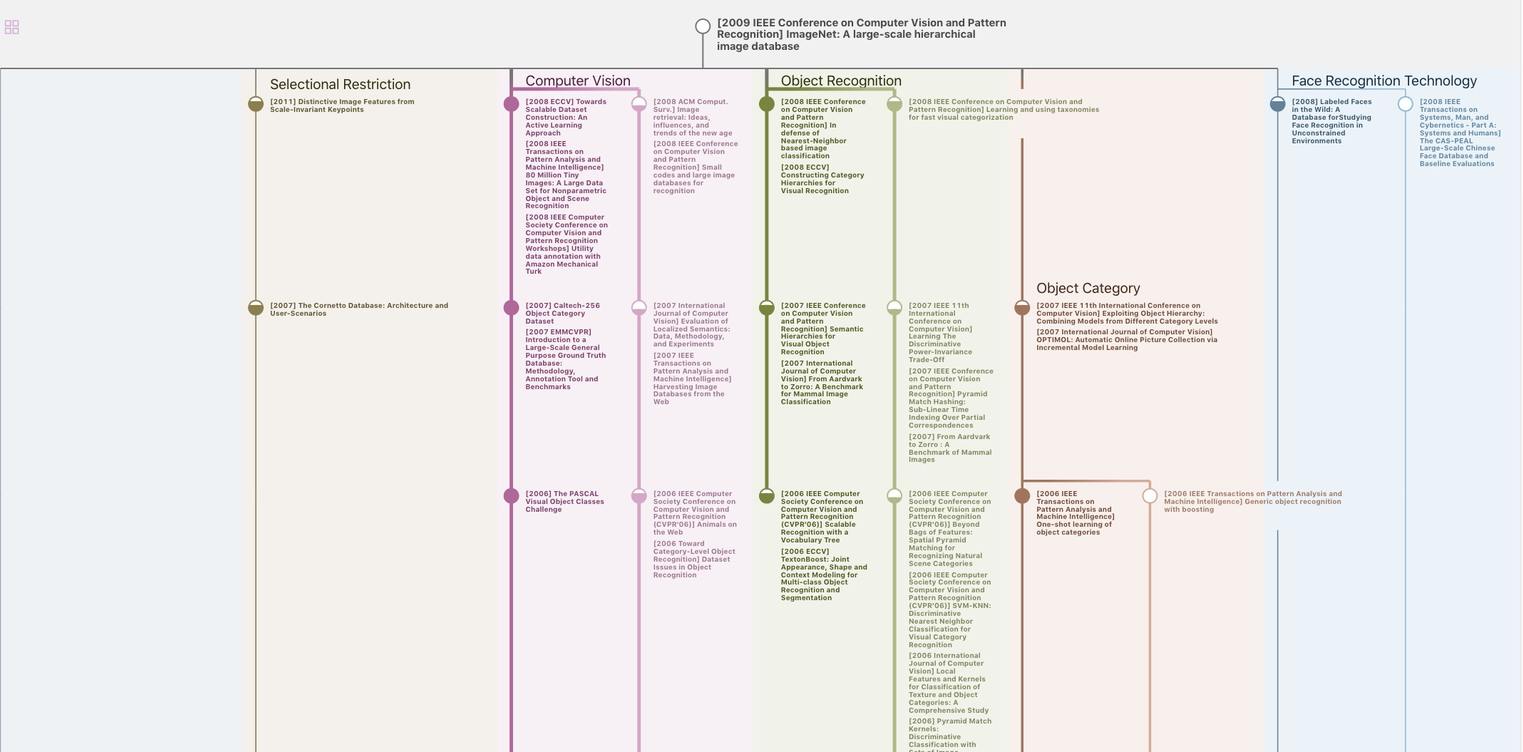
Generate MRT to find the research sequence of this paper
Chat Paper
Summary is being generated by the instructions you defined