Overcoming Delayed Feedback via Overlook Decision Making.
2023 IEEE International Conference on Systems, Man, and Cybernetics (SMC)(2023)
摘要
Reinforcement learning is one of the most general paradigms to solve sequential decision making issues on the assumption that the action selection and environmental feedback are instantaneous, however, unfortunately this assumption is rarely true with regard to such ubiquitous delays in real-world system which could degrade the performance of reinforcement learning algorithms. The most common solution to solve a fixed delay problem is to design a forward dynamic model which is used to predict the newest state by recursively iterating over long steps so that a predicted state can be got and it would be taken as the agent's observation to make the newest decision. However, there exists cumulative errors during the iterative process which make long-term prediction inaccurate and further affect agent's decision. Motivated by the goal to reduce cumulative errors, we propose a new algorithm named Multi-step Prediction model with Delayed Observation(MPDO), aiming at accurately predicting future state at longer horizons for better decision making. Our approach includes two parts: a multi-step prediction model and a strategy training based on proximal policy optimization algorithms(PPO). Our model only needs a small amount of data to conduct dynamic modeling quickly, and the accuracy of prediction and iteration speed are higher than traditional methods. Experiments on Gym and MuJoCo show that MPDO achieves higher performance in such different tasks with different delays compared with other state-of-the-art methods, which verify our method's effectiveness.
更多查看译文
关键词
Reinforcement Learning,Delayed Environment,Dynamic Modeling
AI 理解论文
溯源树
样例
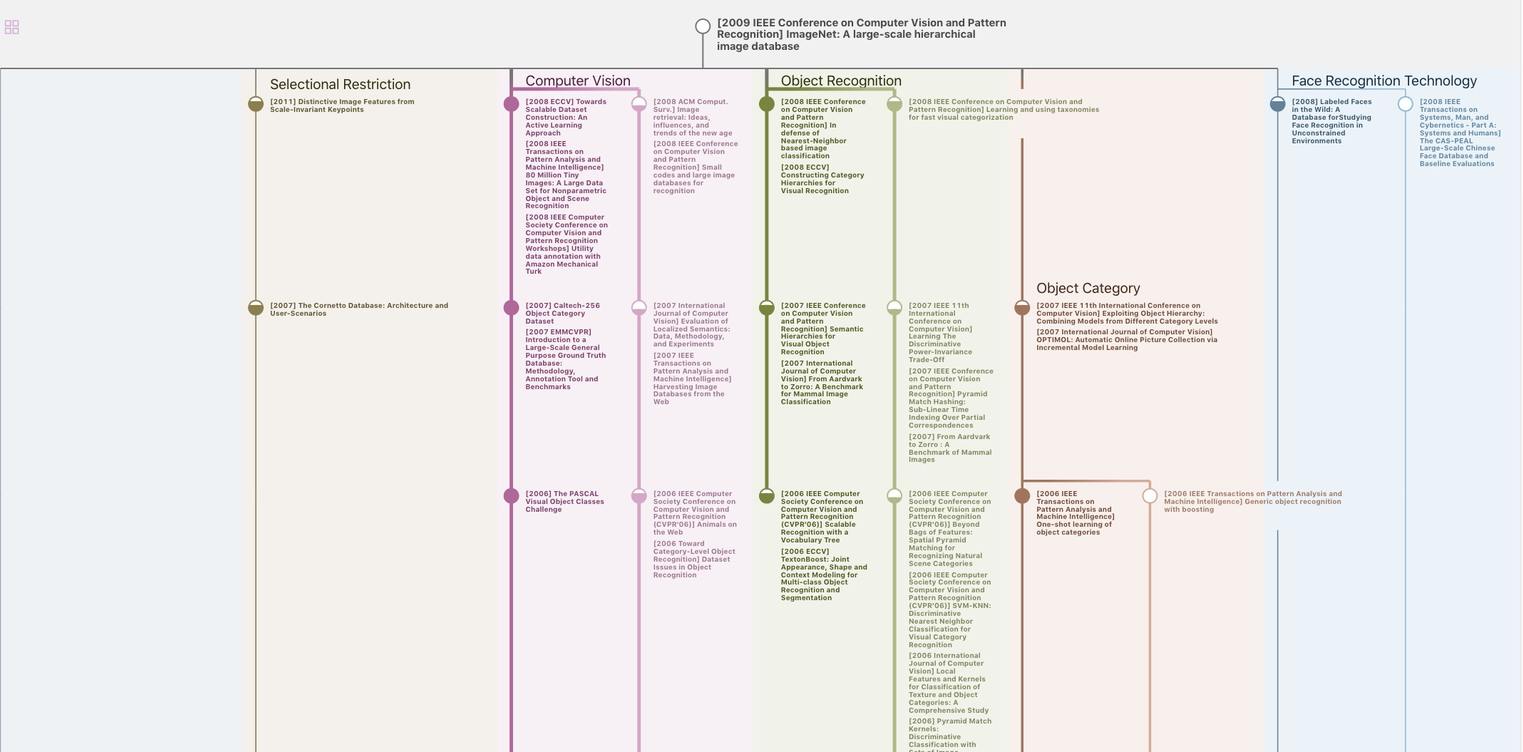
生成溯源树,研究论文发展脉络
Chat Paper
正在生成论文摘要