Tangent Space Sampling of Video Sequence with Locally Structured Unitary Network.
2023 IEEE International Conference on Visual Communications and Image Processing (VCIP)(2023)
Abstract
This study proposes a novel approach for the analysis of high-dimensional time series data utilizing a linear shift-variant transform. Central to the methodology is the Locally-Structured Unitary Network (LSUN), a self-supervised learning transform proficient in capturing the underlying tangent spaces within high-dimensional datasets. This trait equips LSUN with a distinct advantage over existing techniques such as convolutional dictionary learning, which suffers from decreased interpretability due to the redundant selection of filter kernels. The shift-invariance of convolutional models does not directly correspond to the axes of tangent spaces, a challenge well addressed by LSUN. This study focuses on utilizing LSUN for time series analysis to capture structural variations in sequential frames. To optimize this task, LSUN is utilized with total variation regularization, a method known for its ability to preserve edge information. The effectiveness of the proposed model is demonstrated through a video sequence denoising experiment, illustrating its potential to innovate in areas where the analysis of time-series data is crucial. The findings signal the significant potential for this approach to be extended to targets, such as dynamical system identification, further extending its applicability.
MoreTranslated text
Key words
Tangent space sampling,self-supervised learning,time series data analysis,linear transform,unitarity,shift-variance
AI Read Science
Must-Reading Tree
Example
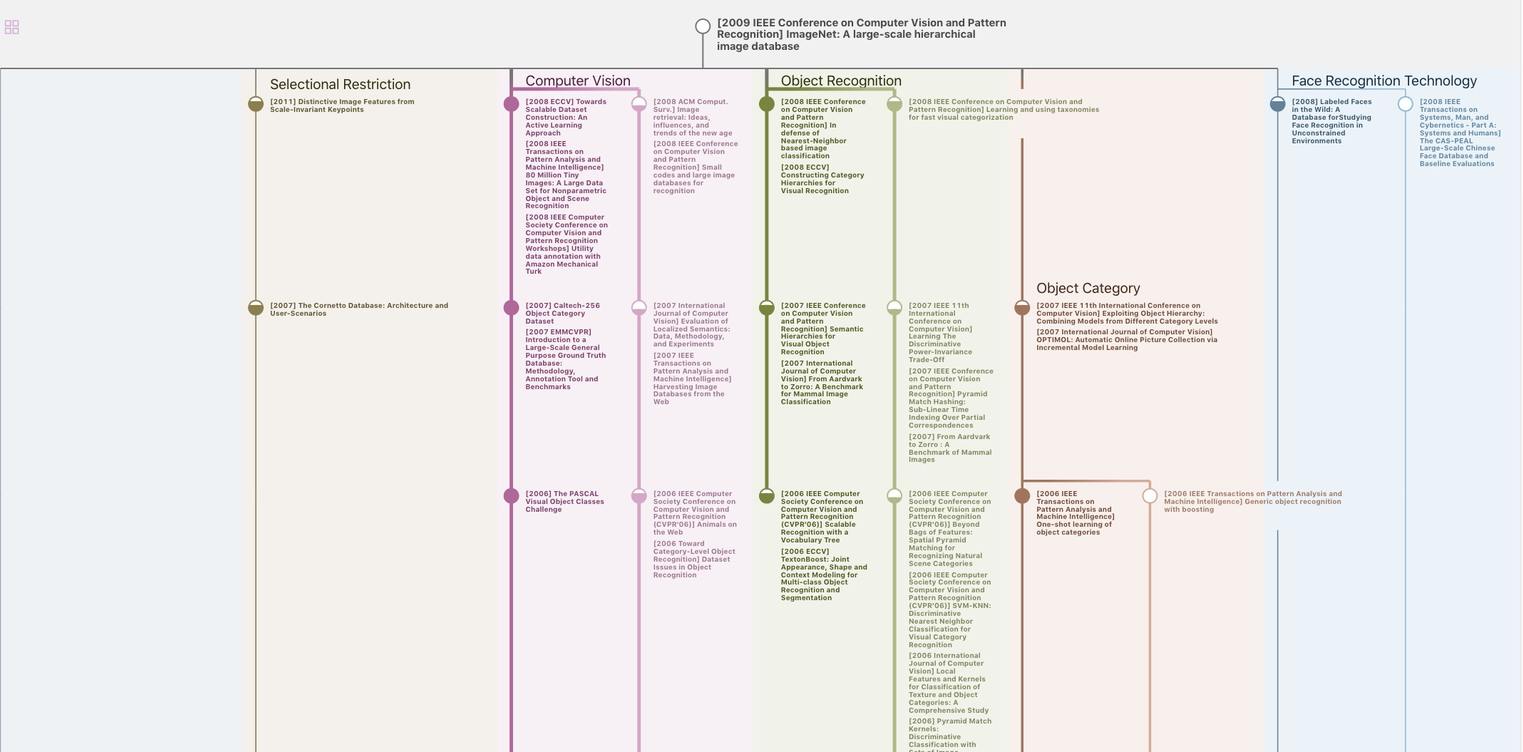
Generate MRT to find the research sequence of this paper
Chat Paper
Summary is being generated by the instructions you defined