A prompt tuning method for few-shot action recognition.
2023 IEEE International Conference on Visual Communications and Image Processing (VCIP)(2023)
Abstract
Vision-language pre-training models learn visual concepts from image-text or video-text pairs, which can be adopted for visual-textual tasks. In this paper, we adopt these concepts as prior knowledge to solve the unreliable problem of minimizing the loss of limited training samples in few-shot action recognition tasks. In particular, a two-stage framework of vision-language pre-training and prompt tuning is designed. In the pre-training stage, multi-modal encoding models are jointly trained on video-text pairs to learn the semantic correspondence between video and text. In the prompt tuning stage, a prompt module with instance-level bias is trained on a few video samples to utilize the pre-trained concepts for the classification task. The experimental results show that the proposed method is superior to the baseline and state-of-the-art few-shot action recognition methods on two public video benchmarks.
MoreTranslated text
Key words
Few-shot learning,Action recognition,Prompt tuning,Vision-language pre-training
AI Read Science
Must-Reading Tree
Example
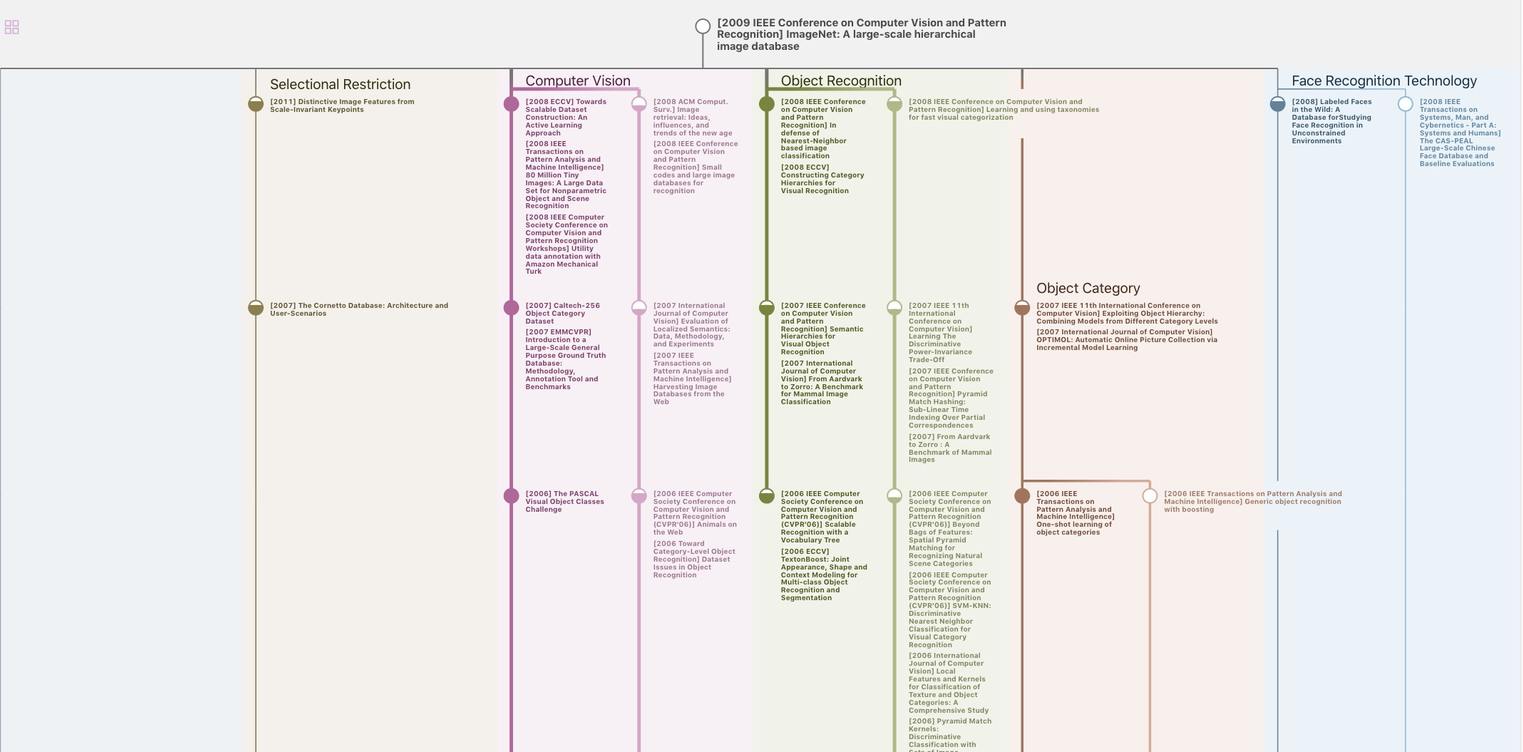
Generate MRT to find the research sequence of this paper
Chat Paper
Summary is being generated by the instructions you defined