Image segmentation of exfoliated two-dimensional materials by generative adversarial network-based data augmentation
CHINESE PHYSICS B(2024)
摘要
Mechanically cleaved two-dimensional materials are random in size and thickness. Recognizing atomically thin flakes by human experts is inefficient and unsuitable for scalable production. Deep learning algorithms have been adopted as an alternative, nevertheless a major challenge is a lack of sufficient actual training images. Here we report the generation of synthetic two-dimensional materials images using StyleGAN3 to complement the dataset. DeepLabv3Plus network is trained with the synthetic images which reduces overfitting and improves recognition accuracy to over 90%. A semi-supervisory technique for labeling images is introduced to reduce manual efforts. The sharper edges recognized by this method facilitate material stacking with precise edge alignment, which benefits exploring novel properties of layered-material devices that crucially depend on the interlayer twist-angle. This feasible and efficient method allows for the rapid and high-quality manufacturing of atomically thin materials and devices.
更多查看译文
关键词
two-dimensional materials,deep learning,data augmentation,generating adversarial networks,07.05.Pj,68.65.-k,84.35.+i,87.64.M-
AI 理解论文
溯源树
样例
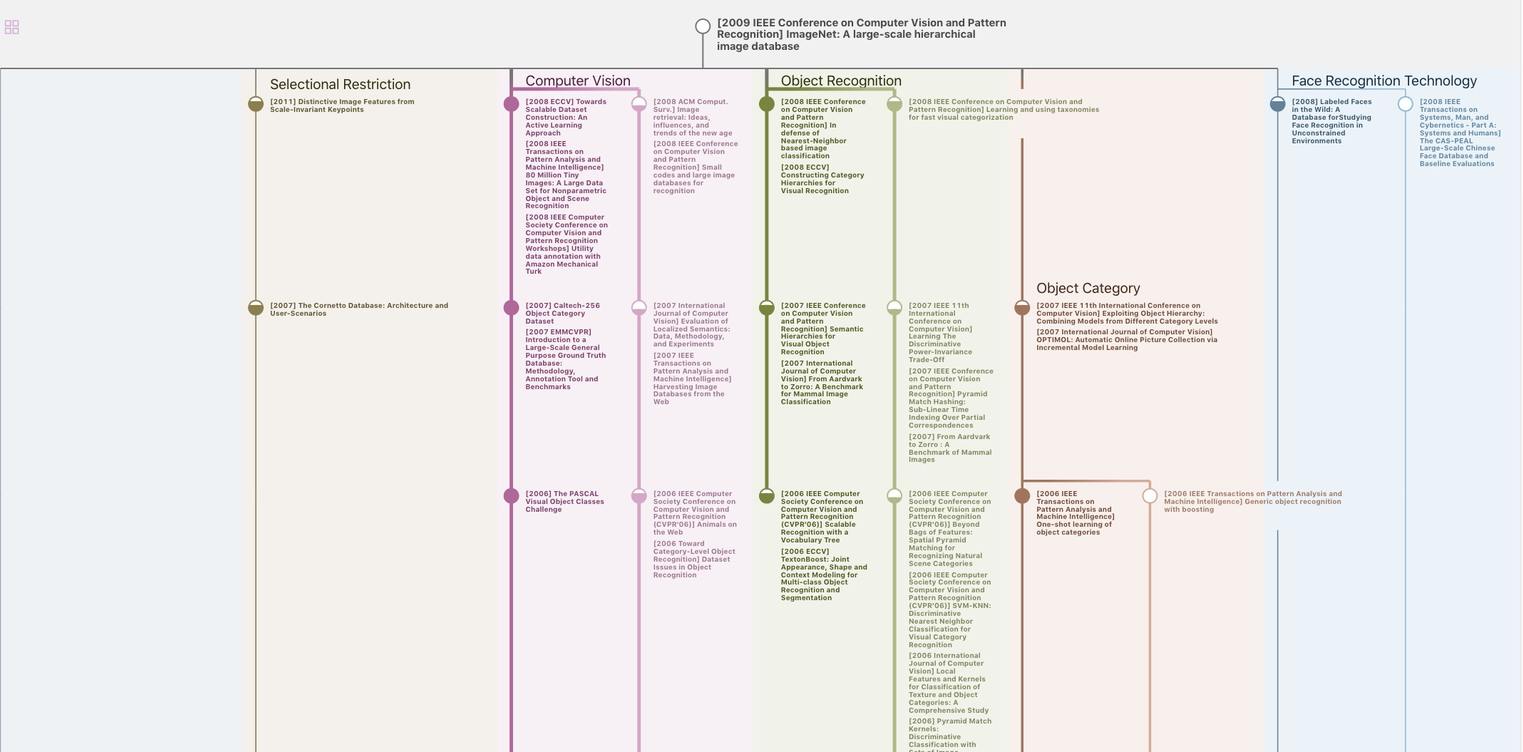
生成溯源树,研究论文发展脉络
Chat Paper
正在生成论文摘要