Weighted ensemble CNN for lung nodule classification: an evolutionary approach
Multimedia Tools and Applications(2024)
摘要
Lung Cancer is the deadliest cancer with maximum mortality rates all over the world. If lung cancers are not detected at an early stage, they will continue to be the leading cause of death due to cancer. Convolution neural networks (CNNs), a type of deep learning approach, have produced better outcomes for diagnosing malignant lung nodules. However, CNN can have variance in output which can be reduced using the ensemble learning technique. CNN ensemble approach also reduces the generalization error and increases the accuracy of lung nodule malignancy prediction using the ensemble CNN learning technique. In this study we reduce the variance in output and also improve the performance of lung nodule classification by using the weighted average ensemble technique on convolution models such as VGG16, Densenet, and Alexnet, and the weights are optimized using an evolutionary algorithm like grey wolf optimizer, whale swarm optimizer, firefly optimizer, differential evolution, particle swarm optimizer, and bat optimizer. The proposed approach uses a heterogeneous ensemble with a weighted approach and is evaluated on the LUNA 16 dataset, on which it has achieved an accuracy, sensitivity, and specificity of 96%, 97.43%, and 93.08% respectively, with an AUC score of 0.95 using an evolutionary algorithm like Bat Optimizer to optimize the weights.
更多查看译文
关键词
VGG16,Densenet,Alexnet,Bat optimizer
AI 理解论文
溯源树
样例
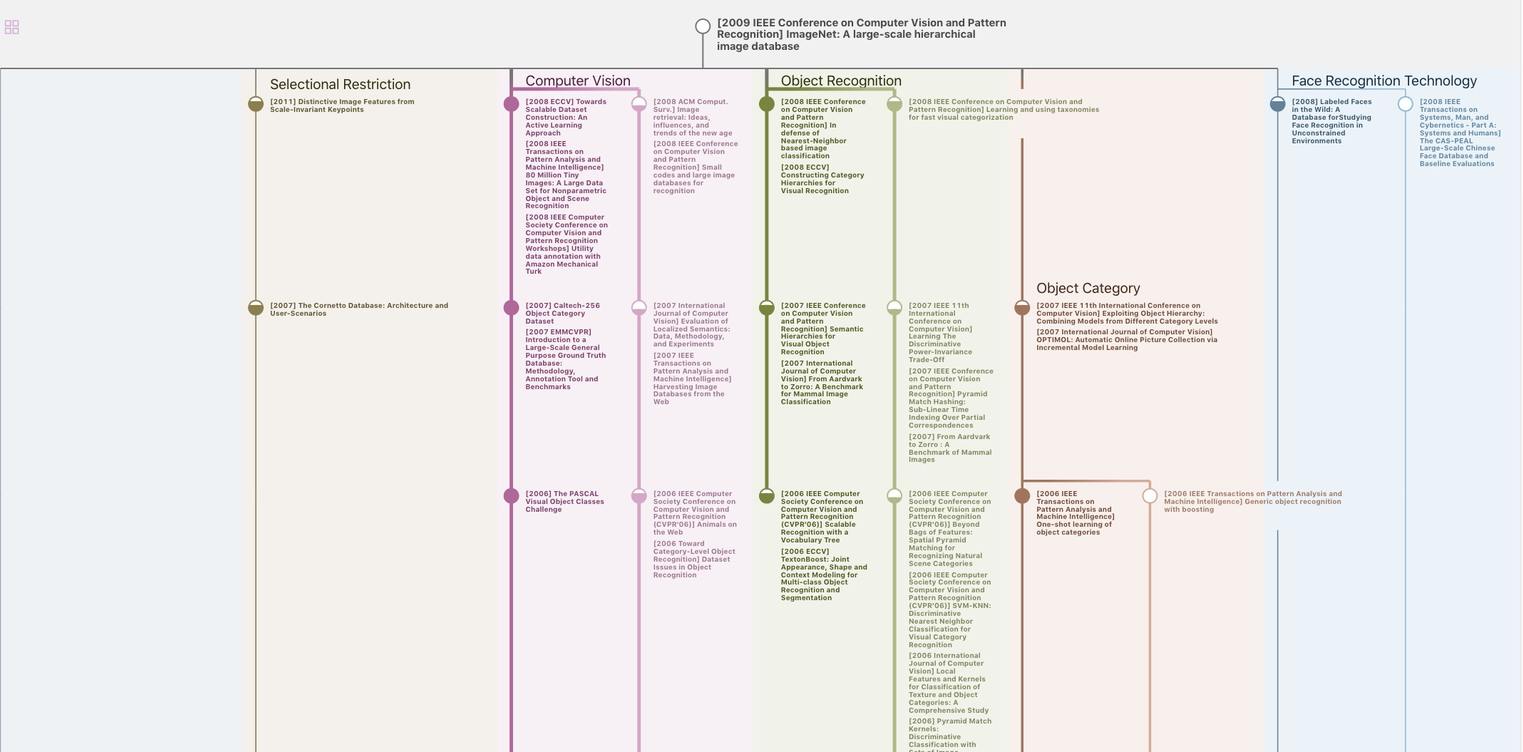
生成溯源树,研究论文发展脉络
Chat Paper
正在生成论文摘要