Evolvable graph neural network for system-level incremental fault diagnosis of train transmission systems
MECHANICAL SYSTEMS AND SIGNAL PROCESSING(2024)
Abstract
Intelligent fault diagnosis and continual learning techniques for train transmission systems are becoming more appealing to ensure the operation safety and reliability of trains. However, existing related methods have the following limitations. 1) They build separate fault diagnosis networks for each key component in train transmission systems, which not only brings burdensome work for training and managing diagnosis networks but also ignores the influence of fault propagation and interaction between adjacent components. 2) They rely on enough classincremental samples for continual learning to obtain the growth diagnosis ability, but the accumulations of incremental fault samples are long -period processes, leading to belated network evolution and hysteretic performance enhancement. To overcome the above -mentioned limitations, an evolvable system -level fault diagnosis framework is proposed for train transmission systems. In the proposed framework, a novel graph neural network is first constructed based on component spatial relationships, which is able to effectively learn and capture the interaction between components and integrate fault diagnosis tasks into a unified framework. Then, an anhysteretic evolution learning mechanism is developed to avoid lengthy waiting for new fault sample accumulations, in which over -fitting due to insufficient incremental fault sample is addressed by employing prototype -based classifiers and diagnostic knowledge is transferred and captured by maintaining and generating prototypes. The effectiveness of the proposed diagnosis framework is verified by taking a case study of fault diagnosis for subway train transmission systems, and its superiority is demonstrated by comparison with some stage -of -the -art diagnosis methods.
MoreTranslated text
Key words
Train transmission system,System-level fault diagnosis,Graph convolutional network,Continual learning,Prototype learning
AI Read Science
Must-Reading Tree
Example
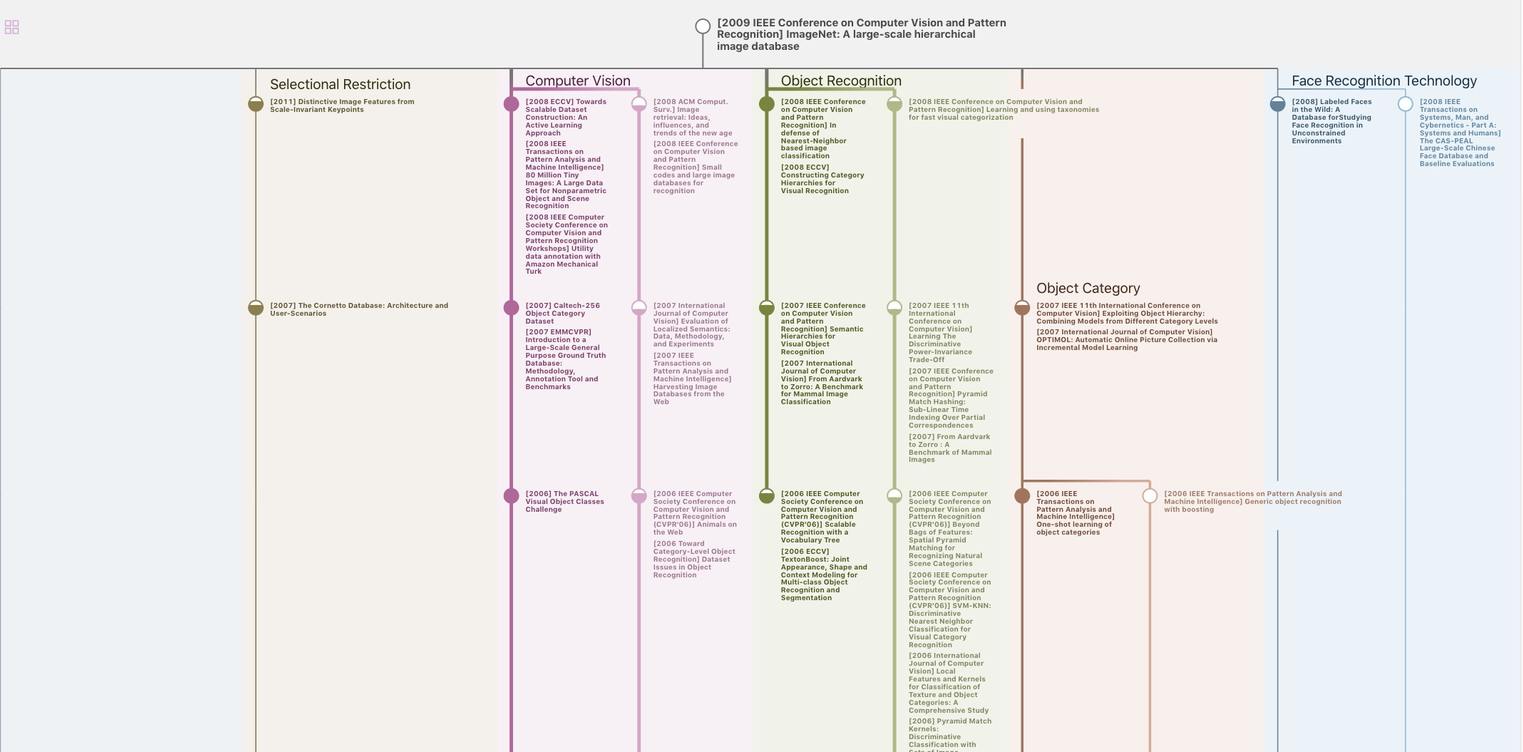
Generate MRT to find the research sequence of this paper
Chat Paper
Summary is being generated by the instructions you defined