OntoMedRec: Logically-Pretrained Model-Agnostic Ontology Encoders for Medication Recommendation
CoRR(2024)
摘要
Most existing medication recommendation models learn representations for
medical concepts based on electronic health records (EHRs) and make
recommendations with learnt representations. However, most medications appear
in the dataset for limited times, resulting in insufficient learning of their
representations. Medical ontologies are the hierarchical classification systems
for medical terms where similar terms are in the same class on a certain level.
In this paper, we propose OntoMedRec, the logically-pretrained and
model-agnostic medical Ontology Encoders for Medication Recommendation that
addresses data sparsity problem with medical ontologies. We conduct
comprehensive experiments on benchmark datasets to evaluate the effectiveness
of OntoMedRec, and the result shows the integration of OntoMedRec improves the
performance of various models in both the entire EHR datasets and the
admissions with few-shot medications. We provide the GitHub repository for the
source code on https://anonymous.4open.science/r/OntoMedRec-D123
更多查看译文
AI 理解论文
溯源树
样例
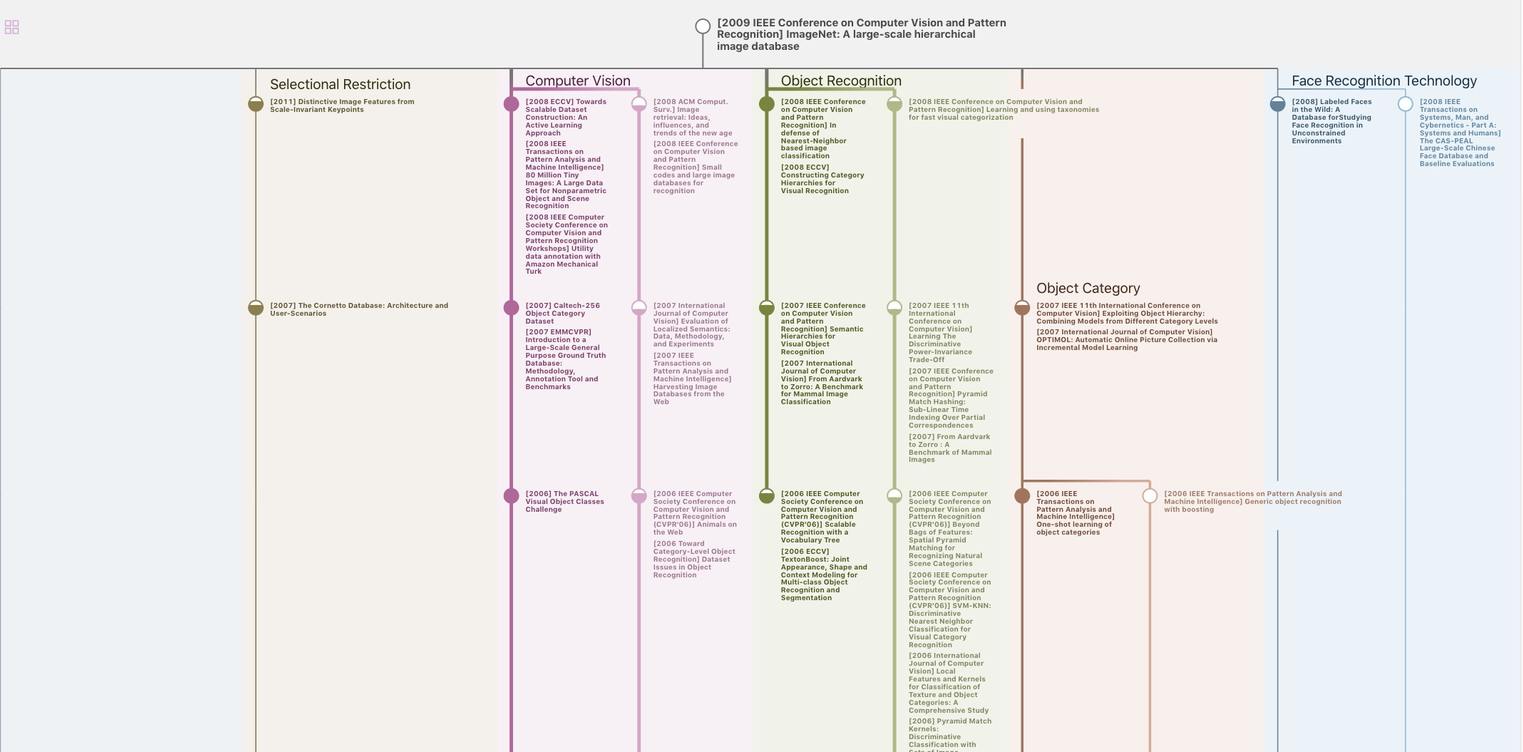
生成溯源树,研究论文发展脉络
Chat Paper
正在生成论文摘要