Adaptive Deep Learning for Efficient Visual Pose Estimation aboard Ultra-low-power Nano-drones
CoRR(2024)
摘要
Sub-10cm diameter nano-drones are gaining momentum thanks to their
applicability in scenarios prevented to bigger flying drones, such as in narrow
environments and close to humans. However, their tiny form factor also brings
their major drawback: ultra-constrained memory and processors for the onboard
execution of their perception pipelines. Therefore, lightweight deep
learning-based approaches are becoming increasingly popular, stressing how
computational efficiency and energy-saving are paramount as they can make the
difference between a fully working closed-loop system and a failing one. In
this work, to maximize the exploitation of the ultra-limited resources aboard
nano-drones, we present a novel adaptive deep learning-based mechanism for the
efficient execution of a vision-based human pose estimation task. We leverage
two State-of-the-Art (SoA) convolutional neural networks (CNNs) with different
regression performance vs. computational costs trade-offs. By combining these
CNNs with three novel adaptation strategies based on the output's temporal
consistency and on auxiliary tasks to swap the CNN being executed proactively,
we present six different systems. On a real-world dataset and the actual
nano-drone hardware, our best-performing system, compared to executing only the
bigger and most accurate SoA model, shows 28
the same mean absolute error (MAE), 3
and the absolute peak performance, i.e., 6
更多查看译文
AI 理解论文
溯源树
样例
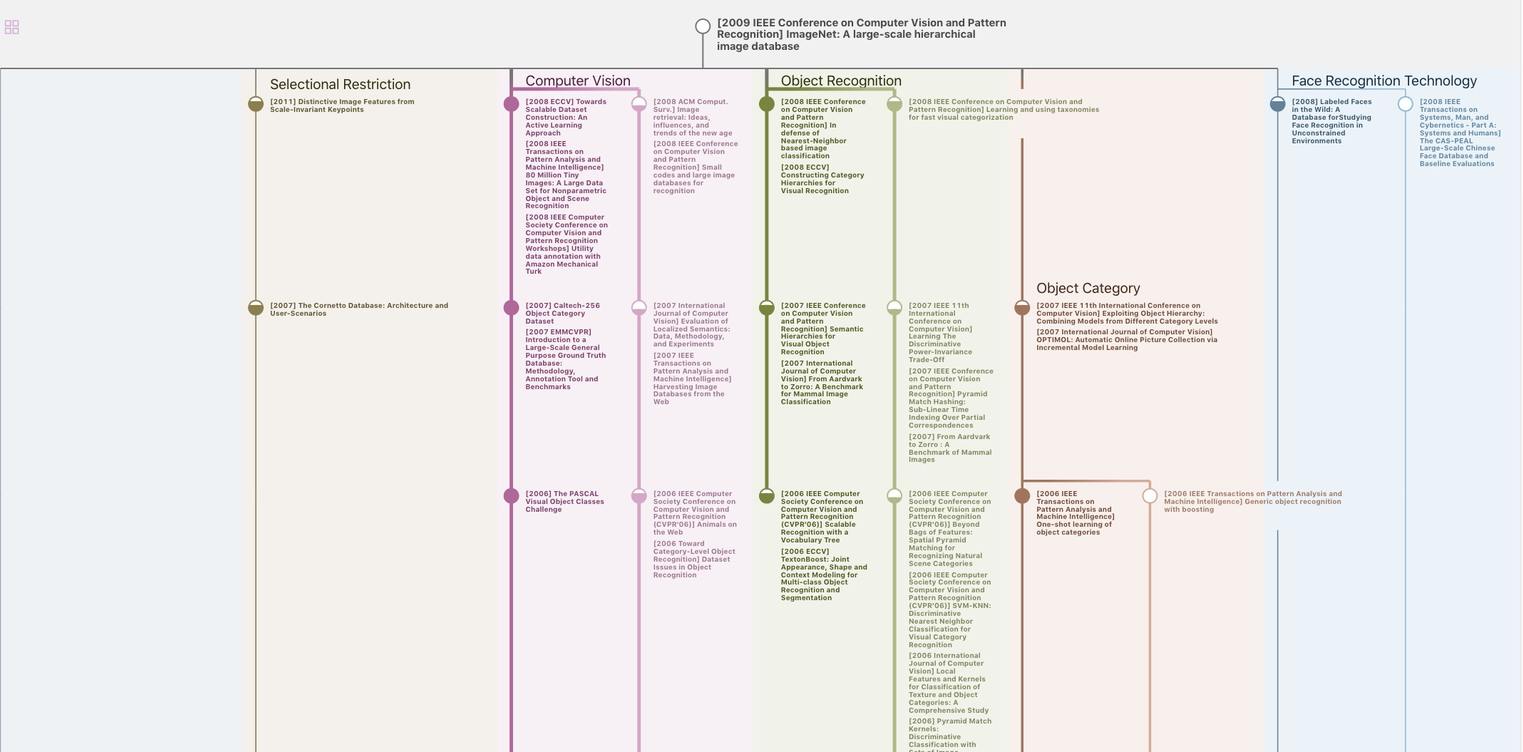
生成溯源树,研究论文发展脉络
Chat Paper
正在生成论文摘要