Semi-parametric Expert Bayesian Network Learning with Gaussian Processes and Horseshoe Priors
CoRR(2024)
摘要
This paper proposes a model learning Semi-parametric rela- tionships in an
Expert Bayesian Network (SEBN) with linear parameter and structure constraints.
We use Gaussian Pro- cesses and a Horseshoe prior to introduce minimal nonlin-
ear components. To prioritize modifying the expert graph over adding new edges,
we optimize differential Horseshoe scales. In real-world datasets with unknown
truth, we gen- erate diverse graphs to accommodate user input, addressing
identifiability issues and enhancing interpretability. Evalua- tion on
synthetic and UCI Liver Disorders datasets, using metrics like structural
Hamming Distance and test likelihood, demonstrates our models outperform
state-of-the-art semi- parametric Bayesian Network model.
更多查看译文
AI 理解论文
溯源树
样例
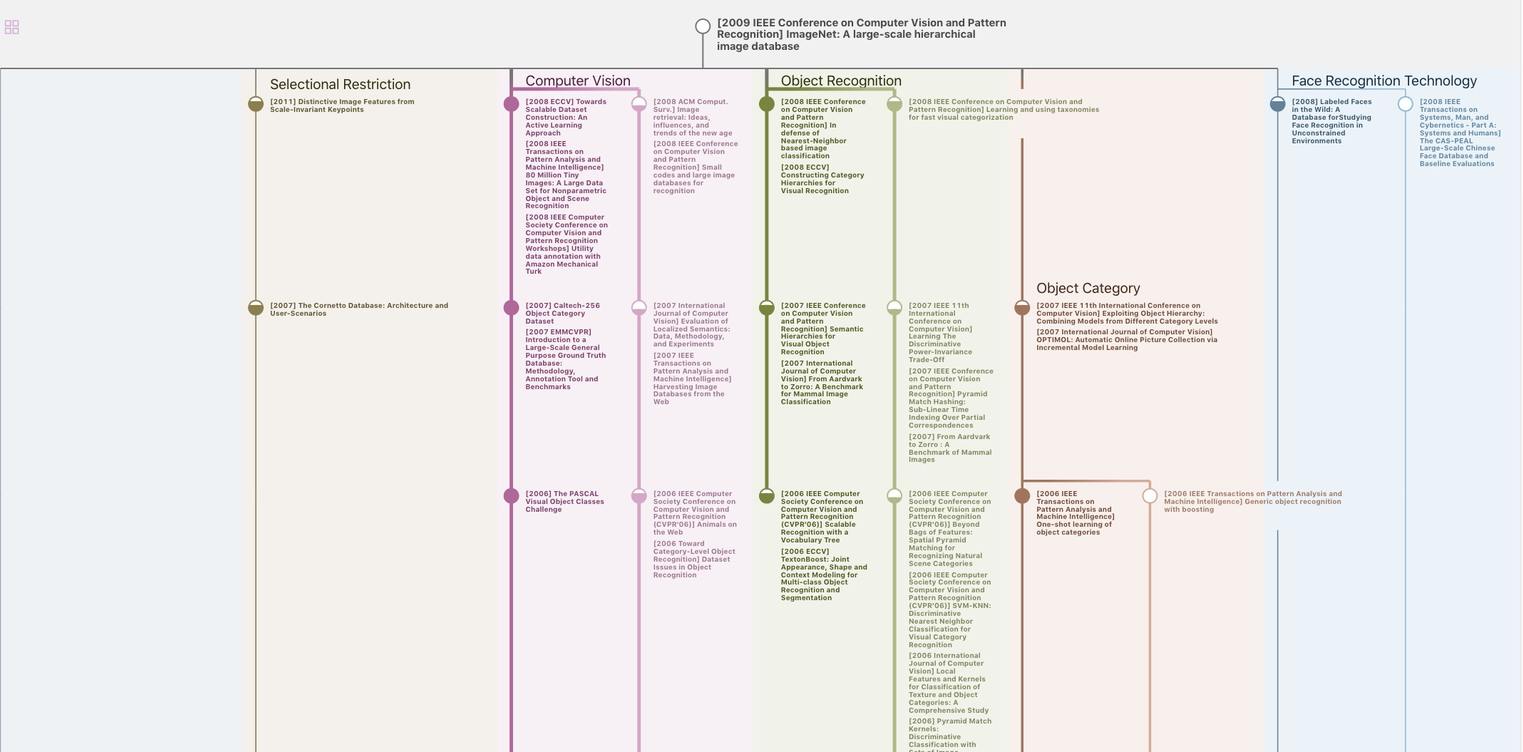
生成溯源树,研究论文发展脉络
Chat Paper
正在生成论文摘要